No.6896
First I need to decide on a representation. There are many ways of representing real numbers. Some well-known representations are Dedekind cuts and Cauchy sequences. Dedekind cuts represent a real number by saying which rational numbers (fractions) are smaller than it and which are equal or greater. Cauchy sequences represent a real number with a sequence of rational numbers that converge to it. You can look up the exact definitions on Wikipedia, which include the all-important details of how to formulate the definitions without referencing the real numbers and creating a circular definition. Another representation that everyone knows is to represent a real number as an infinite string of decimal digits.
Dedekind cuts are a very simple and natural-feeling representation, but I haven't yet been able to figure out how to make addition work with them in a way I can actually implement on a computer. Maybe I could make it work if I read a bit more about what other people have done.
Cauchy sequences in their full generality feel a bit too complicated for my taste. It's possible the complexity at the beginning makes the implementation of stuff later on easier, though. But I'm going to try something else first.
Infinite decimals are nice, and I want whatever representation I use to be convertible into decimal form (rounded at some arbitrary point). You couldn't store an infinite decimal in memory, of course, but you could imagine a function which takes the position of a digit as input, computes the digit, and outputs the digit. A big problem with this is the 0.999... = 1.000... issue. If you are representing a number very close to 1, computing whether the digit in the ones place is 0 or 1 could take a very long time, since you have to compute the number to a high enough precision to tell whether it is larger or smaller than 1. If the number happens to be exactly one, it might even cause our program to get into an infinite loop.
What I'm going to try first is something more general than the decimal representation (and with the problem I've describe avoided, I hope) and more specific than the Cauchy representation.
No.6897
>>6895Thanks. I'm going to be using an interactive theorem prover called Coq, which allows you to write programs and prove theorems about those programs. It's important to verify that these things actually satisfy the properties of real numbers.
I'm hoping to get to the point where I can construct complex numbers and prove the fundamental theorem of algebra. That's the theorem that every polynomial of degree n [for example, x^3 - 7x + 6 has degree 3 because the highest power is x^3] can be factored into n linear factors [in this case, (x-1)*(x-2)*(x+3)], each of which indicates a solution to the equation when the polynomial is set equal to zero [x^3 - 7x + 6 = 0 has solutions x=1, x=2, and x=-3]. It's a very useful theorem, but the proof isn't trivial. Ideally I'll not only have a proof but a constructive proof, that is, a verified program that finds all the solutions.
But how far I get ultimately depends on how fast this goes and when I get tired of it.
No.6898
>>6897>It's a very useful theorem, but the proof isn't trivial.I believe it, but I'm a bit confused how one would even go about constructing a solution to find all solutions to a polynomial, since there's stuff like >4th degree polynomials having no equation to find their solutions.
No.6899
>>6896>What I'm going to try first is something more general than the decimal representation (and with the problem I've describe avoided, I hope) and more specific than the Cauchy representation.My initial attempt will go like this: A real number will be represented by a function that outputs a fractional approximations to the number given the denominator of the fraction. Specifically, given an integer n, it will output an integer m such that m/n is less than 1/n away from the number. For example, if the number is pi, and you called the function with the integer 7, both 21 and 22 would be acceptable outputs. If you called the function with the number 100, it would output either 314 or 315.
Given this representation, it would be easy to extract the decimal digits of a number; simply call the function with the desired power of 10 as an argument. But you would never be sure the last digit was correct, since it could be rounded up or down. This is necessary to avoid the 0.999... = 1.000... related hangup I mentioned. If the number if very close to 1, it doesn't have to compute the number to a very high precision; it could simply give you the approximation 1000/1000 for any number between 0.999 and 1.001, because it wouldn't have to know which side of 1 the number was on.
No.6900
>>6898There's no formula for the solutions using only the tools of addition, subtraction, multiplication, division, integer powers, and nth roots. But that doesn't mean you can't find the solutions numerically to whatever precision you desire.
No.6901
>>6898>>6900One proof goes like this: Draw two complex planes, in one of which we'll be plotting inputs to the polynomial, and in the other we'll be plotting the outputs. If you move your point in the input plane around a sufficiently big circle, it can be shown that the point in the point in the output plane goes around the origin n times, where n is the degree of the polynomial. The number of times a loop goes around a point is called its winding number.
If you split the loop in the input plane into two smaller loops, you get two smaller loops in the output plane. If the dividing line between the two output subloops passes through the origin, that means you've found an input that gives an output of zero. Then you can use polynomial long division to find an (n-1)-degree equation with the remaining solutions. But usually there will not be a solution precisely on the dividing line. In that case, the winding numbers of both subloops should add up to the original winding number. If you further divide the loops into smaller and smaller pieces, focusing on the subloops that have nonzero winding number, you find smaller and smaller input loops whose corresponding output loops make smaller and smaller loops around the origin. As the loops become smaller, they approach points in the input plane which map to the origin in the output plane. These are your solutions.
Of course, making this all rigorous, especially the idea of winding numbers, is a bit of work.
No.6905
Finally got Coq updated to the latest version (8.13.2).
The Coq standard library
https://coq.inria.fr/library/index.htmlcomes with implementations of arbitrary-size integers and rationals, which I'll be using instead of re-implementing since it's the reals I'm interested in learning about. It also comes with an implementation of constructive reals (marked experimental), but using that would defeat the point of this exercise. Possibly of interest is the standard library's abstract interface for constructive reals; at some point I'd like to see if I can get my implementation to implement the interface. But I'll ignore it for now since I don't want to influence my first design attempt too much.
No.6913
To implement
>>6899 we could consider defining the reals as follows:
Definition R := Z -> Z.
"Z" is the type of arbitrary-size integers (imported indirectly via QArith), so this is saying the type "R" is the type of functions from integers to integers.
But not all functions that take integers as input and output will be valid for our purpose. As illustrated in the pic, a function that maps
1 => 1 [0/1 < x < 2/1]
2 => 2 [1/2 < x < 3/2]
3 => 2 [1/3 < x < 3/3]
4 => 3 [2/4 < x < 4/4]
could be a valid function since all these ranges overlap. But a function that maps
1 => 1 [0/1 < x < 2/1]
2 => 2 [1/2 < x < 3/2]
3 => 2 [1/3 < x < 3/3]
4 => 6 [5/4 < x < 7/4]
because it is impossible for our number to be both greater than 5/4 and less than 3/3.
Coq has the capacity to define subset types. A member of a subset type is a member of the original type along with a proof that it satisfies some property. The proof part is just there for type checking purposes, and gets removed if you ask to turn a Coq function into an executable program.
We need all our lower bounds to be lower than our upper bounds, so we can define our type as follows:
Require Import QArith.
Definition Rfun := positive -> Z.
Definition is_valid_Rfun f :=
forall x y, (f x - 1) # x < (f y + 1) # y.
Definition R := {f | is_valid_Rfun f}.
I've also changed the input type from "Z" to "positive". This simplifies the definition of the validity condition. If x was negative, [f(x)-1]/x would become the upper bound and [f(x)+1]/x the lower bound, and I'd have to add logic to handle that. The "positive" type is used by Coq as one of the arguments for the constructor "#" of the rational type "Q", so it's also convenient for this reason. And I don't have to worry about what to do if the function is called with value 0.
No.6916
To find the sum of two numbers at precision 1/n, we'll need to ask for the addends (numbers to be added) at a higher precision. You might think we need precision 1/(2n) because the maximum possible error on the sum is 1/(2n) + 1/(2n) = 1/n. But suppose we want a sum with precision 1/10 and so request the addends with precision 1/20, and the rational approximations we get back are 6/20 and 1/20. When we add them together, we get 7/20, it has to be rounded to either 6/20 = 3/10 or 8/20 = 4/10, introducing another 1/20 of error.
What we'll instead need to request the addends at precision 1/(4n). Each addend has an error less than 1/(4n), and then we introduce an additional error of at most 2/(4n) when we round the numerator to the nearest multiple of 4 so that the fraction can be reduced from denominator 4n to n.
So the addition of two of our functions can be defined as
Definition Rfun_plus (f g : Rfun) : Rfun :=
fun x => ((f (4 * x)%positive + g (4 * x)%positive + 2) / 4)%Z.
Here, the ": Rfun" part are type annotations. Coq can infer types in many scenarios, so I'll only add them when they're not obvious, either to the reader or to Coq. The "%positive" and "%Z" are scope keys. They tell Coq that symbols like "+", "-", "*", and "/" inside the "%Z" are operations on numbers of the "Z" type, that is, integers. And inside the "%positive", they're operations on numbers of the "positive" type, positive integers.
We're not done with addition yet, since we've only defined an operation on the type "Rfun". To make this into an operation on "R" (functions with proof of validity as defined in
>>6913), we'll need to write a function that takes proofs the input functions are valid and returns a proof the output function is valid. In other words, a proof of the implication "if the input is valid, then the output is valid." This is just one example of how a logical idea (implication) can be understood in terms of a computational idea (functions). There are many others, and this relationship between logic and programming, called the Curry–Howard correspondence, is fundamental to how Coq works.
https://en.wikipedia.org/wiki/Curry%E2%80%93Howard_correspondence
No.6917
>>6916>In other words, a proof of the implication "if the input is valid, then the output is valid."Before I start proving this, I want to define a few other operations on the raw "Rfun" type. To prove that one of our functions is valid, we said we needed to prove that all the lower bounds were lower than all the upper bounds (
>>6913). We can generalize this idea to define a few other things. If we want to prove that one number is less than or equal to a second number, we need to prove that the lower bounds of the first number are all lower than the upper bounds of the second number. To prove that two numbers are equal, we need to prove that the lower bounds of both numbers are lower than the upper bounds of both numbers.
We can define "less than or equal" like this:
Definition Rfun_le (f g : Rfun) : Prop :=
forall x y, (f x - 1) # x < (g y + 1) # y.
Given this definition, we can define two numbers to be equal if each is less than or equal to the other. And we can restate our definition of validity in terms of "less than or equal"; a function makes a valid number if it is less than or equal to itself.
Definition Rfun_eq (f g : Rfun) : Prop :=
Rfun_le f g /\ Rfun_le g f.
Definition is_valid_Rfun (f : Rfun) : Prop :=
Rfun_le f f.
I've explicitly indicated the type of Rfun_le, Rfun_eq, and is_valid_Rfun to emphasize the difference between booleans (type "bool" in Coq) and propositions (type "Prop"). For a program to tell the numbers between two numbers that are equal and two numbers that are merely very, very close, it would have to compute both numbers to infinite precision. So we will not attempt to write a function that takes in two numbers and delivers a true or false answer -- type "bool" -- telling whether they are exactly equal. But we can in some cases show that two numbers are exactly equal by a mathematical proof. What we have done is define the type of proof that will be required to demonstrate equality. If a proof of this type exists for two given numbers, the proposition that they are equal is true. If it can be shown that no proof of this type can exist, the proposition is false. In Coq and other type theory based systems, we represent propositions as types whose members are proofs. "Prop" is a higher-order type whose members are types of proofs.
No.6919
>>6917I think I'll add the type annotation to Rfun and R too. These also define types, so their type is a higher-order type of types. But instead of being in "Prop", they are in a different type of types, called "Set", which obeys slightly different rules. There are several reasons for the design decision in Coq to keep "Set" and "Prop" separate, but one of them is so that proof objects, whose types are in "Prop", can be removed from Coq functions when they are turned into programs executable outside Coq.
Definition Rfun : Set :=
positive -> Z.
Definition R : Set :=
{f | is_valid_Rfun f}.
Also, let's stick all the code so far in a public place:
https://github.com/ccd0/toyreals0
No.6921
I should give due credit to some inspirations. The method of representing real numbers described in
>>6899 is similar to the "Eudoxus reals" of Schanuel in that we are considering functions from integers to integers, but the tests of validity and equality are different. And the definition of "less than or equal"
>>6917 and some of the definitions that follow from it are basically the same as Conway's definition for surreal numbers.
No.6925
File:uu.png (1.15 MB,1280x720)
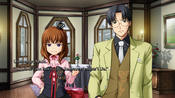
>>6917Since "less than or equal" doesn't output a real number, we don't have to prove anything to define it on "R" instead of on "Rfun". We can just use "proj1_sig" to extract a member of "Rfun" from a member of the subtype "R".
Definition Rle (x y : R) : Prop :=
Rfun_le (proj1_sig x) (proj1_sig y).
Since the definition of equality doesn't depend directly on the implementation, I decided to get rid of the definition on "Rfun" an just define it on "R" directly.
Definition Req (x y : R) : Prop :=
Rle x y /\ Rle y x.
We can also define "greater than or equal" as "less than or equal" flipped around:
Definition Rge (x y : R) : Prop :=
Rle y x.
You might think we would define "less than" as the negation of "greater than or equal". But instead we will define it like this:
Definition Rfun_lt (f g : Rfun) : Prop :=
exists x y, (f x + 1) # x <= (g y - 1) # y.
Definition Rlt (x y : R) : Prop :=
Rfun_lt (proj1_sig x) (proj1_sig y).
The reason to choose this definition has to do with the nature of existence proofs. To prove the negation of A >= B, we would need to assume that every lower bound of B is lower than every upper bound of A, and derive a contradiction from that assumption. From this information, we could reasonably conclude that there is some lower bound of B which is greater than or equal to some upper bound of A. But it wouldn't tell us how to find them.
Mathematics has many examples of things that can be proven to "exist" even though nobody can show you one of them. One famous example is the Banach–Tarski paradox, which says that there's a way to decompose a ball (the interior of a sphere) into five pieces which can be moved and rotated so as to reassemble them into two identical copies of the original ball.
Existence proofs in Coq are not like this, at least not by default. To prove that something exists with a given property, you have to write a program that will actually find it, along with a proof of the property for that object. You can add axioms to Coq to let you reason more like a typical mathematician, but then if you try to run your proof as a program, it can't finish because it needs a proof of the axiom, and you don't have one.
By defining "less than" as requiring a direct existence proof, we require a proof of A < B to explicitly tell us to what precision we need to calculate A and B to find an upper bound on A less than or equal to a lower bound on B. (A is strictly less than its upper bounds, and B is strictly greater than its lower bounds.)
Some reasonable questions:
1. Why should we care what information the proof objects contain when they will be erased upon creating an executable program outside Coq?
2. If the proof doesn't tell us how to find the bounds on A and B showing A < B, can't we just find out by calculating the numbers at increasingly greater precision? We know it will eventually terminate since the bounds have to exist.
I think I have a partial answer to (1), which I'll talk about it in a later post after I've completely tested my thoughts. But I'm still trying to figure these questions out.
No.6926
>>6899>But you would never be sure the last digit was correct, since it could be rounded up or down.Correction: It could be more than the last digit incorrect. For example, 0.9991 could be rounded to 1.000.
No.6935
>>6916>But suppose we want a sum with precision 1/10 and so request the addends with precision 1/20, and the rational approximations we get back are 6/20 and 1/20. When we add them together, we get 7/20, it has to be rounded to either 6/20 = 3/10 or 8/20 = 4/10, introducing another 1/20 of error.Thinking on this some more, it seems wasteful, so I've changed the implementation a little. Now "Rfun" is
Definition Rfun : Set :=
positive -> Q.
and the idea is that if you request the number with precision 1/n, any denominator is acceptable as long as the result is within 1/n of the correct value. The implementations of <=, < and + have been updated accordingly:
Definition Rfun_le (x y : Rfun) : Prop :=
forall tolx toly, x tolx - (1 # tolx) < y toly + (1 # toly).
Definition Rfun_lt (x y : Rfun) : Prop :=
exists tolx toly, x tolx + (1 # tolx) <= y toly + (1 # toly).
Definition Rfun_plus (x y : Rfun) : Rfun :=
fun tol => Qred (x (2 * tol)%positive + y (2 * tol)%positive).
The reason for the old behavior was to make computing decimals easier, so I've written a function "RQapprox_w_den" that emulates the old behavior, returning a fraction p/q within 1/q of the true value. I've also added an "RQapprox" function that at the moment just calls the "Rfun" type function in the number to return an approximation within 1/n of the true value. This is intended to create a layer of abstraction so functions can call "RQapprox" to get approximations and won't have to be updated if the underlying implementation of "R" is changed again.
Require Import Qround.
Definition RQapprox_w_den (den : positive) (x : R) : Q :=
Qfloor (RQapprox (2 * den) x * (Zpos den # 1) + (1 # 2)) # den.
Definition Rfun_plus (x y : Rfun) : Rfun :=
fun tol => Qred (x (2 * tol)%positive + y (2 * tol)%positive).
No.6941
>>6935>Definition Rfun_le (x y : Rfun) : Prop :=> forall tolx toly, x tolx - (1 # tolx) < y toly + (1 # toly).oops, should be
Definition Rfun_lt (x y : Rfun) : Prop :=
exists tolx toly, x tolx + (1 # tolx) <= y toly - (1 # toly).
No.6942
I've noticed a problem with this representation
>>6899 which also impacts the slightly revised version in
>>6935. Consider the function which when asked to calculate a real number with precision 1/n, outputs the rational number 1/n. So for any positive integer n, this number is supposed to greater than 0/n and less than 2/n. That makes the number greater than 0, but for any positive rational number p/q, the number is less than 2/(2q) and therefore less than p/q. So this implies an infinitesimal number. But there's nothing in the validity condition that forbids this function.
(If we go by our definition of "Rfun_lt" instead of the intended relationship of the number with its bounds, this number is not greater than the number represented by a function that always returns 0, which would be the most obvious way to define 0 in this system. But it is greater than what we would probably define to be its additive inverse, a function that when asked to calculate to precision 1/n returns -1/n.)
There's nothing inherently wrong with infinitesimals, but we're trying to model the reals, and the reals don't include infinitesimals. If a number system includes infinitesimals, that makes it something other than the reals.
Fortunately, this is pretty easy to fix at this point. We just need to change the meaning of calculating to precision 1/n to mean calculating with error less than
or equal to 1/n. Given this change, "<" must be changed to "<=" and vice versa in the definitions of "Rfun_le" and "Rfun_lt":
Definition Rfun_le (x y : Rfun) : Prop :=
forall tolx toly, x tolx - (1 # tolx) <= y toly + (1 # toly).
Definition Rfun_lt (x y : Rfun) : Prop :=
exists tolx toly, x tolx + (1 # tolx) < y toly - (1 # toly).
No.6943
>>6942Note that although there's nothing wrong with infinitesimals, there is something wrong with equality not being transitive, that is, having numbers such that a = b and b = c but a <> c as mentioned in the parenthetical remarks. So even if you wanted infinitesimals, you'd need to fix things. On that note I should correct
>>6921. This was not the definition of "less than or equal" that Conway used for the surreals. His definition was that the first number was less than all the second number's upper bounds, and the first number's lower bounds were all less than the second number (if we take x < y to mean not y <= x). Not sure why I got that mixed up in my head.
No.6948
Working on a function to decide whether one real is less than or greater than another given a proof that they're not equal (so we don't get stuck forever testing at greater and greater precision). The fact that a proof of "less than" gives me two possibly different levels of precision rather than one is annoying me. Thinking about changing the validity condition so that the intervals the number is supposed to be in are required to be nested; that is, when you raise the precision, the lower bounds can only get higher, and the upper bounds can only get lower.
No.6951
>>6948>Working on a function to decide whether one real is less than or greater than another given a proof that they're not equal (so we don't get stuck forever testing at greater and greater precision).While thinking about this, I thought it might be easier to do comparison to zero first, which was my original goal anyway. (I'm thinking forward to division, where dividing by zero is disallowed but dividing by positive and negative numbers close to zero are both allowed and produce drastically different results.)
So I added a conversion from the rational type "Q" to the real type "R".
Definition Q2Rfun (x : Q) : Rfun :=
fun tol => x.
Theorem Q2Rfun_valid : forall x, is_valid_Rfun (Q2Rfun x).
intros x tol1 tol2.
apply Qplus_le_r.
compute.
discriminate.
Qed.
Definition Q2R (x : Q) : R :=
exist is_valid_Rfun (Q2Rfun x) (Q2Rfun_valid x).
This makes the first proof that any particular "Rfun" function is valid.
No.6963
>>6948>>6951Some progress towards this. I've proven that if x is nonzero, then it either has a negative upper bound or a positive lower bound.
Theorem Rneq0_exists_witness :
forall x, Rneq x (Q2R 0) -> exists t,
RQapprox t x + (1 # t) < 0 \/ 0 < RQapprox t x - (1 # t).
Proof is in the repo. Usually Coq proof scripts aren't very informative to read unless you're stepping through them, anyway.
So even in code where the proof object containing the number we have to input to the function to find one of these bounds has been thrown away, it should be possible (although not terribly efficient) to test every positive number until we find one that generates either a negative upper bound or a positive lower bound.
There's a module called ConstructiveEpsilon where they've worked out how to recover numbers from thrown-away proof objects in this manner. The non-obvious (at least to me) is how to convince Coq that the function terminates. Coq doesn't let you write functions that aren't proven to terminate.
https://coq.inria.fr/library/Coq.Logic.ConstructiveEpsilon.html
No.6964
I've also proven that the approximations generated for a real number x are within 1/n of x as defined by the "less than or equal to" function defined on R.
Theorem RQapprox_spec_l :
forall t x, Rle (Q2R (RQapprox t x - (1 # t))) x.
Theorem RQapprox_spec_u :
forall t x, Rle x (Q2R (RQapprox t x + (1 # t))).
That's pretty ugly; it ought to look like:
Theorem RQapprox_spec_l :
forall t x, Q2R (RQapprox t x - (1 # t)) <= x.
Theorem RQapprox_spec_u :
forall t x, x <= Q2R (RQapprox t x + (1 # t)).
Or even:
Theorem RQapprox_spec :
forall t x, Q2R (RQapprox t x - (1 # t)) <= x <= Q2R (RQapprox t x + (1 # t)).
But to do that, I need to tell Coq that "<=" means the function "Rleq". I haven't done that yet.
No.6968
>>6948>>6951Comparison to zero is done.
Regarding
>>6925>1. Why should we care what information the proof objects contain when they will be erased upon creating an executable program outside Coq?In my previous testing with ConstructiveEpsilon, it would work when I used a normal constructive proof:
Require Import ConstructiveEpsilon.
Require Import Arith.
Require Import Classical.
Require Extraction.
Definition k := 0.
Lemma lol1 : exists n, k = n.
exists k.
trivial.
Defined.
Definition z1 := proj1_sig (constructive_indefini
te_ground_description_
nat (fun n => k = n) (Nat.eq_dec k) lol1).
Eval compute in z1.
When I had it compute z1, it successfully found 0.
But when I invoked the axiom of the excluded middle, the computation within Coq didn't work:
Lemma lol2 : exists n, k = n.
destruct (classic (exists n, k = n)) as [H|H].
- trivial.
- contradict H.
exists k.
trivial.
Defined.
Definition z2 := proj1_sig (constructive_indefini
te_ground_description_
nat (fun n => k = n) (Nat.eq_dec k) lol2).
Eval compute in z2.
Computing z2 didn't get hung in an infinite loop, but it spat out a program instead of a number. I'll show the output in the next post if there's room.
No.6969
>>6968The output of z2:
= let (a, _) :=
(fix linear_search (m : nat) (b : before_witness (fun n : nat => 0 = n) m) {struct b} : {n : nat | 0 = n} :=
match
match m as n return ({0 = n} + {0 = n -> False}) with
| 0 => left eq_refl
| S m0 => right (O_S m0)
end
with
| left yes => exist (fun n : nat => 0 = n) m yes
| right no =>
linear_search (S m)
(match b with
| stop _ _ p =>
fun not_p : 0 = m -> False =>
match not_p p return (before_witness (fun n : nat => 0 = n) (S m)) with
end
| next _ _ b0 => fun _ : 0 = m -> False => b0
end no)
end) 0
(let (n, p) :=
match classic (exists n : nat, 0 = n) with
| or_introl H => H
| or_intror H =>
match H (ex_intro (fun n : nat => 0 = n) 0 eq_refl) return (exists n : nat, 0 = n) with
end
end in
(fix O_witness (n0 : nat) :
before_witness (fun n1 : nat => 0 = n1) n0 -> before_witness (fun n1 : nat => 0 = n1) 0 :=
match
n0 as n1
return (before_witness (fun n2 : nat => 0 = n2) n1 -> before_witness (fun n2 : nat => 0 = n2) 0)
with
| 0 => fun b : before_witness (fun n1 : nat => 0 = n1) 0 => b
| S n1 =>
fun b : before_witness (fun n2 : nat => 0 = n2) (S n1) =>
O_witness n1 (next (fun n2 : nat => 0 = n2) n1 b)
end) n (stop (fun n0 : nat => 0 = n0) n p)) in
a
: nat
No.6970
>>6968>>6969On the other hand, the extracted programs from
Recursive Extraction z1.
Recursive Extraction z2.
were the same other than the change in variable name.
So I expected when I had comparison with zero done, I would be able to get results in Coq when I used a constructive proof that the number wasn't equal to zero, and that I wouldn't get a satisfactory result otherwise. But my testing so far gives me different results.
Lemma Rneq10 : Rneq (Q2R 1) (Q2R 0).
apply Q2R_neq.
discriminate.
Qed.
Eval compute in Rpositive_bool (Q2R 1) Rneq10.
This constructive proof gives me true, as expected.
Lemma Rneqn10 : Rneq (Q2R (-1)) (Q2R 0).
apply Q2R_neq.
discriminate.
Qed.
Eval compute in Rpositive_bool (Q2R (-1)) Rneqn10.
This constructive proof gives me false, as expected.
Require Import Classical.
Lemma Rneq10 : Rneq (Q2R 1) (Q2R 0).
destruct (classic (Rneq (Q2R 1) (Q2R 0))) as [H|H].
- trivial.
- contradict H.
apply Q2R_neq.
discriminate.
Qed.
Eval compute in Rpositive_bool (Q2R 1) Rneq10.
This non-constructive proof using the axiom of the excluded middle still gives me the correct answer of true.
Axiom Rneq10 : Rneq (Q2R 1) (Q2R 0).
Eval compute in Rpositive_bool (Q2R 1) Rneq10.
Even assuming 1 <> 0 as an axiom works.
Axiom Rneq00 : Rneq (Q2R 0) (Q2R 0).
Eval compute in Rpositive_bool (Q2R 0) Rneq00.
The bogus axiom 0 <> 0 gives me the bogus result true.
I'm still working out what's going on.
No.6971
>>6970Oh, I see what's happening. Because I'm using numbers that I made with "Q2R", the conversion from rationals function, all the rational approximations are the same, so it can calculate the rational approximation whose sign I'm testing without having to know what precision it's calculating to. I'll need to make some numbers with something else to test with.
No.6973
>>6971Addendum: If all of the rational approximations returned are positive, Coq can sometimes figure out that they're positive without knowing the precision. This works for testing, though:
Definition alt1fun : Rfun :=
fun t => 1 - (1 # t).
Lemma alt1fun_valid : is_valid_Rfun alt1fun.
intros t1 t2.
unfold alt1fun.
ring_simplify.
rewrite <- (Qplus_0_l 1) at 2.
apply Qplus_le_l.
discriminate.
Qed.
Definition alt1 :=
exist is_valid_Rfun alt1fun alt1fun_valid.
I had to go through and change "Qed" to "Defined" in the existence proofs because otherwise Coq throws away the value in existence proofs and just keeps track of the fact that they're true. But once that was fixed, Rpositive_bool gave the correct answer of true when given a constructive proof without axioms:
Lemma Rneq_alt1_0 : Rneq alt1 (Q2R 0).
right.
exists 4 % positive, 4 % positive.
reflexivity.
Defined.
Eval compute in Rpositive_bool alt1 Rneq_alt1_0.
But it didn't find the answer when given a proof using the axiom of excluded middle:
Require Import Classical.
Lemma Rneq_alt1_0_indirect : Rneq alt1 (Q2R 0).
destruct (classic (Rneq alt1 (Q2R 0))) as [H|H].
- trivial.
- contradict H.
right.
exists 4 % positive, 4 % positive.
reflexivity.
Defined.
Eval compute in Rneq0_witness alt1 Rneq_alt1_0_indirect.
No.6993
>>6925Even though it's not possible to go from "not less than or equal" to "greater than" without using the axiom of excluded middle, it can be shown pretty easily that the current definition of "less than or equal" is equivalent to "not greater than".
Theorem Rfun_le_not_lt : forall x y, Rfun_le x y <-> ~ Rfun_lt y x.
intros x y.
split.
- intros H1 [tx [ty H2]].
specialize (H1 ty tx).
contradict H2.
apply Qle_not_lt, H1.
- intros H tx ty.
apply Qnot_lt_le.
contradict H.
exists ty, tx.
apply H.
Qed.
So I'm thinking about redefining "less than or equal" as "not greater than".
No.6994
>>6993>So I'm thinking about redefining "less than or equal" as "not greater than".After trying rewriting a few theorems, it looks like it's more trouble than it's worth. The rewrite is easy but it adds needless steps to the proofs. The proof of "RQapprox_spec_l" was what made up my mind; a proof of the form a <= b -> b <= c -> a <= c would have to be replaced with a proof of the form ~ b < a -> b <= c -> ~ c < a.
No.7007
Made some changes to type "R" to simplify things and make it easier to update the underlying implementation if need be.
"R" is now an inductive type instead of piggybacking off the subset type. An inductive type is specified by telling what type of objects you need to use to construct the type. In general, there can be more than one constructor, but for the current implementation of "R" there is only one constructor "Rmake". It takes as arguments a function for getting rational approximations of the number, and a proof that the function satisfies the validity condition.
Inductive R : Set :=
| Rmake (x : Rfun) (p : is_valid_Rfun x) : R.
The function "RQapprox", as before, can be used to get the rational approximations. I've changed it so the real number comes before the precision, because I thought the old order was more confusing, plus this makes it easier to get the function instead of the result of the function, if that's what you want. The theorem "RQapprox_spec" confirms that a function "RQapprox" satisfies the validity condition, so that proofs that need to know this don't have to reach into the "R" object to get the validity proof inside. The theorems formerly known as "RQapprox_spec_l" and "RQapprox_spec_r", which confirmed the intended lower and upper bounds on the number given the approximation, have been renamed to "RQapprox_lower_bound" and "RQapprox_upper_bound".
Everything is set up now to work through the interface of the functions "RQapprox", "Rmake", and "is_valid_Rfun" and the theorem "RQapprox_spec". If the implementation of "R" changes in the future, which it might, these functions can be updated to invoke the new interface while keeping their old behavior.
No.7012
>>7009There's a lot of technical stuff for sure. But I hope the general idea of what I'm doing is comprehensible. Do you understand what I mean when I say that I'm representing real numbers in the computer by computer programs that calculate an approximate value for the number, and that you can ask the program for as precise an approximation you want?
No.7014
>>7012So it's kind of like symbolic computation?
No.7015
>>7014It's similar but there are differences.
Symbolic computation might take an expression like sqrt(2) * sqrt(2) and apply some rule to figure out it's exactly 2. And some expressions it wouldn't be able to do anything with, like 3 * sqrt(2) + 5.
The goal of this thing is to find the approximate value of something like sqrt(2) * sqrt(2) or 3 * sqrt(2) + 5. You could do that with ordinary floating point numbers, but there would be a rounding error at each step, and the accuracy of the answer at the end is dependent on how many steps are involved in the calculation. With this thing, the user decides what accuracy is needed for the final result, and the program figures out how to get it.
If it was trying to do 3 * sqrt(2) + 5 for example, then it would tell the addition program what accuracy you need, and the addition program would have to figure out how accurately 3 * sqrt(2) and 5 need to be computed. And this continues down the chain; the multiplication program, given an accuracy target from the addition program, would have to figure out how accurately 3 and sqrt(2) need to be computed. And so on.
No.7024
>>6968I rewrote the comparison function, and now it works for comparison of any two numbers, not just comparison to zero.
I also moved a lot of proof details out of existence proofs into separate lemmas because the existence proofs need to use "Defined" (see
>>6973) meaning Coq keeps the details of the proof in memory. The only details I need it to keep are how to calculate the number that's been proven to satisfy some property, so the details of verifying it satisfies the property can go into a proof using "Qed".
The new comparison function plus proofs ends up being a bit longer, so I moved it to a separate file. I'm considering trimming it down to just what it needs to compare with zero while keeping the other improvements. But first I want to work a bit on other stuff.
No.7025
>>7015I realized a problem with my model. Consider the operation of squaring a number. Suppose we need the estimate to be within 0.01 of the correct result. If the number we're trying to square is 2, calculating it with an accuracy of 0.001 would work; 1.999^2 and 2.001^2 are both less than 0.01 away from 4. But if we're trying to square 2000, that's not enough accuracy; 1999.999^2 and 2000.001^2 are each a little more than 4 away from the correct result 4000000. We can make it work by changing the accuracy to 0.000001; 1999.999999^2 and 2000.000001^2 are both within 0.01 of 4000000. Those of you who know a little calculus will recognize that the slope of y = x^2 is 2x. The larger the number I want to square, the greater the slope of the function, and the more accuracy I need to achieve my target accuracy on the square of the number.
The problem here is that we first have to know what the number is before knowing with what accuracy to calculate it.
One way to handle this would be to first request the number at some arbitrary accuracy, then use the first estimate of the number to calculate the accuracy we need. The problem with this solution is that it causes an exponential blowup in the number of function calls. Consider calculating (3*pi^2)^4. To calculate (3*pi^2)^4, it calculates 3*pi^2 twice, at two different levels of accuracy. Each calculation of 3*pi^2 calculates pi^2 twice, so we have to calculate pi^2 four times. And each calculation of pi^2 calculates pi twice, so we have to calculate pi eight times.
The easiest approach I've thought of so far is to have each real number come with an upper bound on its absolute value.
No.7026
>>7025>One way to handle this would be to first request the number at some arbitrary accuracy, then use the first estimate of the number to calculate the accuracy we need. The problem with this solution is that it causes an exponential blowup in the number of function calls.>The easiest approach I've thought of so far is to have each real number come with an upper bound on its absolute value.I thought of what might be a simpler way to do it. I could have each real number come with a suggested initial accuracy for computing the first estimate (and perhaps with a precalculated value at that accuracy). Then the convention would be that calculating the output of a function at the initial accuracy only requires calculating the inputs at the initial accuracy.
No.7028
>>7025>The problem with this solution is that it causes an exponential blowup in the number of function calls. Consider calculating (3*pi^2)^4. To calculate (3*pi^2)^4, it calculates 3*pi^2 twice, at two different levels of accuracy. Each calculation of 3*pi^2 calculates pi^2 twice, so we have to calculate pi^2 four times. And each calculation of pi^2 calculates pi twice, so we have to calculate pi eight times.Thinking about this some more, I don't think this kind of behavior can be avoided. For example, if we're calculating sin(x) via the Taylor series x - x^3/3! + x^5/5! - x^7/7! + ..., it will call the function to calculate an approximation to x with each term. This will have to be solved with memoization, which means that functions remember what their result from the last time they were called with a given input instead of computing it all over again.
No.7038
I've decided to change the implementation of a real number to the following:
(1) a sequence of lower and upper bounds for the number, implemented as a stream.
(2) a function which when given a target accuracy, picks a pair of bounds out of the sequence that are sufficiently close to each other
(3) proofs that the bounds of (1) are nonempty and nested, and that the function (2) works
Streams in Coq are a co-inductive type, something I'm not very familiar with and am having to learn about. The gist is co-inductive types can represent infinite data types. Streams represent an infinite sequence of values, which are only actually calculated when needed. Once entries in the sequence have been calculated, they don't need to be calculated again the next time someone asks for that entry. So they can be used to implement memoization.
Here's where Streams are in Coq's standard library:
https://coq.inria.fr/library/Coq.Lists.Streams.htmlIn the middle of rewriting what has to be rewritten now. In good news, I've got my first co-inductive proof working:
Theorem Q2R_all_nonempty :
forall x, QCI.all_nonempty (Q2R_stream x).
Proof.
intro x.
cofix IH.
split; [|exact IH].
unfold QCI.nonempty.
cbn.
apply Qle_refl.
Qed.
No.7044
>>7038Looking at this again, this is too complex. That's only one of three proof obligations just to implement a constant. The implementation details of memoization don't need to be exposed to the function performing the calculation, and they shouldn't be relevant to the proof that the function works. Starting another pass at the redesign.
No.7045
>>7025I found a nice approach. I'm adding a calculated error to the estimates generated by the function, then letting the target accuracy be an arbitrary rational number and requiring target accuracy * error <= 1. Then the target accuracy can be set to zero which gives you the bounds on the number.
No.7062
Yay! Addition has been defined.
Compute R.Qapprox_w_den (R.plus (R.ofQ 3) (R.ofQ 5)) 100.
= 8.00
: Q
No.7076
>>7062Defining addition took a while because I was thinking about how best to prove the validity conditions for the result.
To review and clarify how my implementation of real numbers works right now:
I'm currently representing a real number as a structure with three parts:
(1) A function which takes a target accuracy as input and delivers a rational estimate of the number and an (inclusive) error bound on the estimate.
(2) A proof that the error bounds on the function are consistent; that is, that for any two target accuracies, the resulting error bounds
estimate(target1) - error(target1) <= x <= estimate(target1) + error(target1)
estimate(target2) - error(target2) <= x <= estimate(target2) + error(target2)
overlap. It is sufficient to prove that for any input target accuracies target1, target2,
estimate(target1) - error(target1) <= estimate(target2) + error(target2)
since this automatically implies that
estimate(target2) - error(target2) <= estimate(target1) + error(target1)
estimate(target1) - error(target1) <= estimate(target1) + error(target1)
estimate(target2) - error(target2) <= estimate(target2) + error(target2)
will also hold, by changing the values of target1 and target2.
(3) A proof that the errors on the output acheive the target accuracy, as defined by
target * error(target) <= 1.
For positive values of the target accuracy, this means the error must be less than or equal to 1/target, a number that we can make as small as we want but will never be zero. If the target accuracy is zero or negative, any non-negative error is acceptable.
For part (1), the addition function delivers an estimate of the result (at accuracy = target) by adding the estimates of the inputs (at accuracy = 2 * target). The error on the estimate is obtained by adding the errors on the input estimates.
For the output of the addition function, part (3) is fairly simple to prove. When asked to calculate the result with accuracy = target, it in turn requests the inputs with accuracy = 2*target. Since the inputs are of the real number type, they satisfy part (3) of its definition, meaning the addition function can rely on the errors on the input acheiving their target accuracy:
2 * target * error(input1) <= 1
2 * target * error(input2) <= 1
Adding these inequalities and dividing by 2, we get:
target * (error(input1) + error(input2)) <= 1
target * error(output) <= 1
No.7077
>>7076Part (2) is the one I spent a lot of time thinking about. It's fairly easy to prove for addition, but I wanted to prove it in a way that would also be easy for more complicated functions.
To prove that the error bounds overlap, we first show that the errors are computed correctly. That is, that if x and y are both within a given error range
x0 - dx <= x <= x0 + dx
y0 - dy <= y <= y0 + dy
the result of adding x and y should be within the error range we compute:
(x0 + y0) - (dx + dy) <= x + y <= (x0 + y0) + (dx + dy)
The next step is fairly generic, so let's first imagine we're trying to prove part (2) for a function of one variable. See pic related. When we evaluate the function with two different target accuracies, it requests its input with two different target accuracies. These two requests may result in different estimates of the input number. But since the input is of the real number type, is satisfies part (2) of its definition, meaning the error ranges on the two estimates overlap. Since the error ranges overlap, there is a point within both ranges. We'll use the midpoint between the larger lower bound and the smaller lower bound. Since the point is within both ranges, if we evaluate the function on this point, the output will be within the error ranges for both output estimates, provided the errors were calculated correctly. Thus the error ranges for the output estimates overlap, and part (2) is satisfied. This argument easily generalizes to functions of multiple real numbers.
No.7078
>>7077We can do a bit better, though. Although I haven't gotten to it yet, I'll soon want to prove that adding equal numbers produces equal output. That is, if
a = c
b = d
then
a + b = c + b.
The notion of equal real numbers deserves a little explanation. Our real number type is based on ways of calculating approximations for a number. For any number, there are many ways of calculating it. For example, approximate values of pi can be calculated by the well-known but highly inefficient sum
4 - 4/3 + 4/5 - 4/7 + 4/11 - 4/13 + 4/15 - 4/17 + ...
or the more practical sum
(16/5 - 16/(3*5^3) + 16/(5*5^5) - 16/(7*5^7) + ...) - (4/239 - 4/(3*239^3) + 4/(5*239^5) - 4/(7*239^7) + ...)
or various other methods. So we will have multiple different representations of the same number.
Coq's standard library has a definition of equality for which something like
a = c -> b = d -> a + b = c + b
can be proven trivially. We could try to prove this type of equality for different ways of calculating the same number, but this notion of equality is too strong for our purposes. The reason is that Coq's equality implies that all properties of equal objects be the same. But that isn't true for our representations of real numbers because different ways of approximating a number will yield different estimates of the number.
Since we can't use Coq's definition of equality, we have to define our own. What we are constructing, a type plus a notion of equivalence on that type, is called a setoid. (Or rather, it will be a setoid once I prove reflexivity, symmetry, and transitivity.) The definition I have know is that two representations of a number are equal if the error ranges on the estimates from one always overlap the error ranges on the estimates from the other. Since we're using our own definition of equality, we'll need our own proof of
a = c -> b = d -> a + b = c + b
and corresponding proofs for all the other functions that take real numbers as inputs.
This proof isn't finished yet, but looking forward to having to prove this, I've generalized the proof of part (2) for the output of addition a little. I'm letting the two overlapping estimates for the inputs come from two different representations of a number, provided those representations are equal. Having shown that the two output ranges overlap, we can easily be able to show that the two output numbers are equal.
No.7097
>>7077I made the generic part of this proof into a separate theorem. I couldn't figure out a way to state the theorem for functions of n real numbers that wasn't more trouble than it was worth, so I wrote separate theorems for functions of 1 and 2 real numbers. If I find myself needing to use the theorem for functions of 3 or 4 real numbers, I'll revisit this.
No.7099
>>7078Transitivity relations on the "less than" ("lt") and "less than or equal" ("le") relations are now proven.
That is, if a < b and b < c, a < c.
Also:
If a <= b and b <= c, a <= c.
If a < b and b <= c, a < c.
If a <= b and b < c, a < c.
"Equals" ("eqv") proven an equivalence relation, meaning:
a
== a
If a
== b, b
== a.
If a
== b and b
== c, then a
== c.
And addition proven to be compatible with it:
If a
== b and c
== d, then a + b
== c + d
Also proved that "equals" is equivalent to the negation of "neq", which may be a bad name since "neq", requiring a constructive existence proof, is not equivalent to the negation of "eqv". I think some people use the word "apart" for this reason.
No.7103
So Coq has a very powerful system for declaring notations so that you can write x + y instead of plus x y everywhere. But apparently Coq doesn't allow you to import notations from a module without importing the entire fucking module with all of the namespace pollution that entails. You can declare a submodule within a source file and then declare the notations outside the module so that they can be used without polluting the namespace, but then you can't use your own notations. I looked at how their standard library solves the problem, and the answer was to declare all the notations twice, once inside, then again outside.
https://github.com/coq/coq/blob/V8.13.2/theories/NArith/BinNat.vI guess that's what I'm going to have to do...
No.7120
>>7103Notations for less than etc. and addition are now implemented. Also several other minor proofs have been proven.
No.7125
Always a good feeling to delete code.
$ git commit -am "Simplify."
[master e5c1198] Simplify.
1 file changed, 67 insertions(+), 133 deletions(-)And it still computes, even inside Coq, without getting hung up on opaque stuff. Reworking an old example
>>6973 to the changes in the code since then:
Require Import Coq.QArith.Qminmax.
Definition alt1fun : RE.estimator :=
fun t => let err := (/ Qmax t 1)%Q in
RE.make (1 - err) err.
Lemma alt1_consistent : RE.consistent alt1fun.
Proof.
intros t1 t2.
unfold alt1fun, RE.min, RE.max.
cbn.
ring_simplify.
rewrite <- (Qplus_0_l 1) at 3.
apply Qplus_le_l.
apply Qle_shift_div_r.
- apply Q.max_lt_iff.
right.
reflexivity.
- rewrite Qmult_0_l.
discriminate.
Qed.
Lemma alt1_meets_target : RE.meets_target alt1fun.
Proof.
intro t.
apply Qle_shift_div_r.
- apply Q.max_lt_iff.
right.
reflexivity.
- rewrite Qmult_1_l.
apply Q.max_le_iff.
left.
apply Qle_refl.
Qed.
Definition alt1 :=
R.make alt1fun alt1_consistent alt1_meets_target.
Lemma Rapart_alt1_0 : alt1 =/= (R.ofQ 0).
right.
exists 0%Q, 3%Q.
reflexivity.
Defined.
Compute Rlt_bool alt1 (R.ofQ 0) Rapart_alt1_0. = false
: bool
No.7130
I proved that real numbers that always return the same approximate values for a given target accuracy are equal. That made it easy to prove addition commutative,
Theorem plus_comm : forall x y, x + y == y + x.since it follows from the commutativity of rational number addition. Associativity will require something extra because in x + (y + z), x gets computed at the same target accuracy as y + z, which means that y and z are computed at twice the target accuracy as x.
No.7132
>>7130Associativity done. Wrote a function to compute numbers at a higher target accuracy, and proved that the output of the function was equal to the input. Then used that to fix the hangup with associativity.
No.7152
4chanX is broken :DDDD
No.7158
>>7152Fixed, thanks for bringing it to my attention. For the future I made
>>7155 which will be a better place to talk about breakages.
No.7160
I'm making another minor change to the specification of the real number type. Instead of requiring errors on the returned estimates to satisfy target_accuracy * error_bound <= 1 as explained in
>>7045, I'm changing it to strictly less than one, target_accuracy * error_bound < 1. The reason is that I'm seeing many situations where I want an error smaller than a given value.
One simple example is finding the decimal approximation to a real number. Originally, before I distinguished between target accuracies (propagated backward) and error bounds (propagated forward), I wanted the decimal approximations to either be the correct answer rounded up or rounded down (although you wouldn't know which in any particular case). That is, if I computed a number to 6 decimal places and got the answer 0.463200, it would tell me that the actual number was strictly larger than 0.463199 and strictly smaller than 0.463201. To achieve this, we need to estimate the number with error strictly less than 0.0000005. That's because an estimate that would be rounded to 0.463200 could be anywhere between 0.4631995 (inclusive according to the usual rule) and 0.4632005 (exclusive according to the usual rule). If the estimate is 0.4631995 and the error is 0.0000005 or greater, 0.463199 would be a possible value of the number.
Another recent example is the proof I'm working on of the fact that you can add a number to both sides of an inequality. That is, if x < y, then x + z < y + z.
The proof goes as follows. If x < y, then by the definition I've chosen for "less than", you can get the functions for calculating x and y to provide rational estimates x1, y2 with rational error bounds dx1, dy2 on the estimates respectively such that x1 + dx1 < y2 - dy2. Let's call the difference between these two numbers eps = (y2 - dy2) - (x1 + dx1). Let us request estimates of x + z and y + z each with errors smaller than eps/3. Then the calculation of x + z will request estimates x3, z3 of x and z with error bounds dx3, dz3 smaller than eps/6, and will return x3 + z3 as the estimate of the sum with error bound dx3 + dz3. Similarly, the calculation of y + z will request estimates y3, z3 with error bounds dy3, dz3 smaller than eps/6, and will return an estimate y3 + z3 and an error bound dy3 + dz3. We have
(x3 + z3) + (dx3 + dz3)
< (x3 + z3) - (dx3 + dz3) + 2eps/3 [since (dx3 + dz3) < eps/3]
= (x3 - dx3) + (z3 - dz3) + 2eps/3
<= (x1 + dx1) + (z3 - dz3) + 2eps/3 [the type requires error bounds on different estimates x to be consistent]
= [(y2 - dy2) - eps] + (z3 - dz3) + 2eps/3
= (y2 - dy2) + (z3 - dz3) - eps/3
<= (y2 + dy3) + (z3 - dz3) - eps/3 [the type requires error bounds on different estimates y to be consistent]
< (y2 - dy3) + (z3 - dz3) [since dy3 < eps/6]
= (y2 + z3) - (dy3 + dz3)which satisfies the definition of "less than" in x + z < y + z.
Note that the error bounds still have to use less than or equal; that is, an error bound dx on an estimate x1 is saying that x1 - dx <= x <= x1 + dx. Otherwise, you can define a function that states that the number is between 0 < x < 2eps for any positive rational number eps. But the real numbers aren't supposed to have infinitesimals. Changing the require relationship between target accuracies to error bounds to use "less than" does not create this problem, though. As long as "less than or equal" is used for the error bounds, any set of error intervals satisfies one of the following:
(1) All error intervals include zero, in which case the number being represented is zero.
(2) At least one error interval has a lower bound greater than zero, in which case there is a positive rational less than or equal to the number.
(3) At least one error interval has an upper bound less than zero, in which case there is a negative rational greater than or equal to the number.
In no case is the number an infinitesimal. Conditions (2) and (3) cannot happen simultaneously because the definition of the type disallows inconsistent bounds on the number.
There might be situations I haven't seen yet in which I need the error bounds of estimates to be less than or equal to some target value, rather than strictly less than. But in that case we can just request an error bound strictly less than our target, and it will automatically be less than or equal to our target. This is simpler than what I have to do under the current definition, which is arbitrarily picking some number between 0 and the number I need the error bounds to be strictly less than (for example, by dividing the number by 2), and requesting error bounds less than or equal to that.
No.7161
>>7160Correction, The last three lines should contain y3 not y2:
(x3 + z3) + (dx3 + dz3)
< (x3 + z3) - (dx3 + dz3) + 2eps/3 [since (dx3 + dz3) < eps/3]
= (x3 - dx3) + (z3 - dz3) + 2eps/3
<= (x1 + dx1) + (z3 - dz3) + 2eps/3 [the type requires error bounds on different estimates x to be consistent]
= [(y2 - dy2) - eps] + (z3 - dz3) + 2eps/3
= (y2 - dy2) + (z3 - dz3) - eps/3
<= (y3 + dy3) + (z3 - dz3) - eps/3 [the type requires error bounds on different estimates y to be consistent]
< (y3 - dy3) + (z3 - dz3) [since dy3 < eps/6]
= (y3 + z3) - (dy3 + dz3)
No.7162
>>6925>2. If the proof doesn't tell us how to find the bounds on A and B showing A < B, can't we just find out by calculating the numbers at increasingly greater precision? We know it will eventually terminate since the bounds have to exist.This question was bugging me. The general question is this. Suppose we have a statement P(n) about natural numbers and an algorithm for computing whether P(n) is true for any given number (we say that P is "decidable.") Suppose also that although we haven't found an n such that P(n) is true, we have proven that the assumption that P(n) is false for all n leads to a contradiction. Then we should be able to find such an n such that P(n) is true by simply testing the natural numbers one by one until we find one that works. Since we now have an algorithm for computing n such that P(n) is true, it seems like this ought to count as a constructive proof that there exists an n such that P(n). The question is whether this can be proven in Coq or the intuitionistic logic it is based on, or if it would need to be added as an axiom in order to be used.
After some searching, I found a thread on Reddit asking the question:
https://www.reddit.com/r/math/comments/a2mkm4/can_the_following_proposition_be_proven_in/So apparently this is called "Markov's principle":
https://en.wikipedia.org/wiki/Markov%27s_principleIt is known to be independent of (cannot be proved true or false in) Martin-Lof type theory:
https://arxiv.org/pdf/1602.04530.pdfAnd also of CIC, the logic Coq is based on:
https://link.springer.com/chapter/10.1007/978-3-319-89884-1_9As an interesting aside, the Reddit thread had an interesting explanation of an algorithm which solves NP-hard problems in polynomial time assuming P = NP:
>You enumerate proofs and algorithms. If there is any provably correct algorithm, then the pair of it and its proof will come up in finite time, and easily be checkable as correct. Then just run that polytime algorithm. Finite time plus polytime is still polytime.
No.7164
Additive inverses and subtraction are now implemented. Additive inverses have been proven to be additive inverses, that is, x + (-x) == 0. Also proved some more theorems about adding to both sides of inequalities.
No.7178
While planning out how to implement multiplication, I started thinking about
>>7028 again. I had a plan to deal with the exponential blowup in the number of levels of accuracy to be computed by putting an optimizing layer between each number object and whatever was using the number. When you requested the number at a given accuracy, it would change the request to one of several preset levels of accuracy, and it would record the result so that the next time that accuracy was requested, it wouldn't have to be recalculated. That would fix the exponential blowup problem, but it was unsatisfying because it was still recalculating the same thing over and over again at many different levels of accuracy. I wanted to find a way to avoid this.
An alternate approach to what I'd been doing so far, at which I made an aborted attempt as described in
>>7038, is representing numbers as a sequence that gets more and more accurate. To calculate a number to a desired accuracy, you just keep calculating the items in the sequence until the error level falls low enough. It's possible to get an exponential blowup with this approach too, in the form of exponentially many items to calculate before you get to the desired accuracy, but this can be ameliorated with an optimizing layer that skips items in the sequence when it's not converging fast enough. And there's still the problem of wasteful recalculation.
Then it occurred to me: What if we represent the numbers as infinite sums? Consumers of numbers, rather than receiving better and better approximations and doing a full recalculation based on the full number, would receive small corrections to the original number, and would make small corrections in their calculations. Although we haven't avoided repeatedly recalculating the numbers, with this approach, each recalculation only does new work. It seems much less wasteful. It's also closer to the ways we usually represent and calculate numbers, such as infinite decimals and Taylor series.
After planning out how to do addition, subtraction, and multiplication in this new representation, I've started rewriting the code. Some of the code won't change much; I'm keeping more or less the same definition of "less than", "equals", and "apart". Some parts, like determining whether a number is positive or negative (given it's apart from zero), have become much simpler. Right now, I'm a little more than halfway through the rewrite. I'm re-implementing addition next.
No.7221
>>7178Finally got addition defined again. That was more work than I expected. (I could have gotten it done easier by just adding termwise but I wanted to do it by interleaving terms taking them from the series with the greater contribution to the remaining error first to avoid calculating one of the numbers the far more precision than I needed. That turned out to be a bit complicated to prove convergent.)
No.7255
>>6897Just a quick question, are there any good guides on getting to learn Coq? Thinking that this sounds like a fairly useful tool if it can really verify the validity of a theorem on the real numbers or not.
No.7257
>>7255I like this one:
http://adam.chlipala.net/cpdt/
No.8858
So could this theoretically find new prime numbers faster? Or does that have no relation to anything
No.8859
>>8858I don't see how it would.
No.8907
I think I'm going to start a rewrite along the lines of what I was talking about in
>>7038. I think I know how to avoid the problems I was having. I've also realized that I can avoid the exponential blowup problem I was talking about by just keeping things simple and just adding/subtracting/multiplying/dividing the sequences termwise. Downside is that some numbers will be calculated to much greater precision than they need to be, but it's not an exponential waste. Maybe at some point in the future I'll implement an efficiency-focused model which represents numbers in some specific base.
The current model is kind of cute despite being impractical for calculations due to the exponential blowup issue, so I'll keep it around on another branch.
I'm also going to try writing out more of my proofs as I go along. I'd like it to be at a level where people can understand more or less what's going on even if they can't read the code. Hopefully this time around there will be more math to write about and less fiddling with technical issues. I'll start with an overview of what the model is and why you might want to model real numbers this way.
No.8908
>>8907Real numbers are the numbers we use to measure quantities such as distance, time, weight, and volume. Some of these quantities can be represented as integers, such as 0, 1, 2, 3 and so on, and -1, -2, -3 and so on. Others can be represented as fractions. such as 1/2 or 3 1/3. Real numbers which can be represented as fractions p/q where p and q are integers are called rational numbers. For example, -2 is rational because it can be represented as -2/1, and 3 1/3 is rational because it can be represented as 10/3.
Not all real numbers can be represented as rational numbers. A classic example is the length of the diagonal of a 1 x 1 square, which equals the square root of 2. It can be proven that there are no integers p and q such that (p/q)² = 2. But we can find p/q which approximately square to 2, such as (14/10)² = 198/100. We can also notice that (15/10)² = 225/100, which indicates that √2 is somewhere between 14/10 and 15/10. With more work, we can approximate √2 with higher accuracy. For example, we can show that it is between 141/100 and 142/100 and that it is between 1414/1000 and 1415/1000.
We can continue the process indefinitely to create an infinite list of approximations. For any natural number k (natural numbers meaning 0, 1, 2, ...), we can take 10^k and look for the smallest integer m such that m² ≤ 2*100^k. Dividing both sides by 100^k and taking the square root, we have m/10^k ≤ √2. Similarly, we can look for the largest integer n such that n² ≥ 2*100^k, and we obtain √2 ≤ n/10^k. We obtain the following list of bounds:
k = 0: 1 ≤ √2 ≤ 2
k = 1: 1.4 ≤ √2 ≤ 1.5
k = 2: 1.41 ≤ √2 ≤ 1.42
k = 3: 1.414 ≤ √2 ≤ 1.415
k = 4: 1.4142 ≤ √2 ≤ 1.4143
k = 5: 1.41421 ≤ √2 ≤ 1.41422
k = 6: 1.414213 ≤ √2 ≤ 1.414214
k = 7: 1.4142135 ≤ √2 ≤ 1.4142136
k = 8: 1.41421356 ≤ √2 ≤ 1.41421357
k = 9: 1.414213562 ≤ √2 ≤ 1.414213563
k = 10: 1.4142135623 ≤ √2 ≤ 1.4142135624
...
Does this list uniquely identify √2 or could there be some other number that satisfies all of these requirements? Suppose there are two numbers that satisfy the k = 0 requirement. Then they can be at most 1 apart. If two numbers satisfy the k = 1 requirement, they can be no more than 0.1 apart, and if they both satisfy the k = 2 requirement, they can be no more than 0.01 apart. In order to satisfy all requirements, the numbers must be no more than 1/10^k apart for every possible value of k no matter how large. In other words, the difference between the numbers must be infinitesimal. Since we're talking about real numbers, which ignore infinitesimal differences between the quantities they measure, that means they're the same number.
We will model real numbers as infinite sequences of intervals. (Although we can't really make an infinite list in a computer program, we can make a list that expands whenever you need an item that hasn't been computed yet.) Each interval will be specified by its minimum and maximum value, which will be rational numbers. Each interval in the sequence tells us that the real number we're describing is at least as large as its minimum value and no larger than its maximum value. We will require:
¥ That the minimum of each interval be less than or equal to the maximum.¥ That each interval is at least as strict as the intervals before it; the minimums must be greater than or equal to previous minimums, and the maximums must be less than or equal to previous maximums.¥ That for any positive rational number, there will eventually be an interval in the sequence whose length (maximum - minimum) is smaller than that number. This will ensure that only one real number meets our infinite list of conditions.
No.8910
>>8909I think I understood it. You compare irrationals to rationals by seeing where in that infinite sequence that fits the irrational the rational can be seen as clearly larger/smaller, right?
No.8911
>>8910I think you've got the idea. One thing I should emphasize is that this point all we've got so far is a description of the number. I haven't written a comparison algorithm for this type yet, but comparing it with a rational number would work pretty much as you describe, going down the list until we find a point in the sequence at which the rational number we're comparing to is outside the bounds.
I should also point out that we can use this type to describe rational numbers. The obvious way to encode x = 1/3 would be like this:
k = 0: 1/3 ≤ x ≤ 1/3
k = 1: 1/3 ≤ x ≤ 1/3
k = 2: 1/3 ≤ x ≤ 1/3
k = 3: 1/3 ≤ x ≤ 1/3
...
But we can also do it like this:
k = 0: 0 ≤ x ≤ 1
k = 1: 0.3 ≤ x ≤ 0.4
k = 2: 0.33 ≤ x ≤ 0.34
k = 3: 0.333 ≤ x ≤ 0.334
...
No.8913
So far we have a datatype that represents real numbers. Next, let's define some operations on that datatype, starting with "less than." We say that one real number x is less than another real number y if at some point k in the two sequences there is a maximum possible value for x which is less than the minimum possible value for y. For example, consider the following two sequences representing the real numbers π and √10:
k = 0: 3 ≤ π ≤ 4
k = 1: 3.1 ≤ π ≤ 3.2
k = 2: 3.14 ≤ π ≤ 3.15
...
k = 0: 3 ≤ √10 ≤ 10/3 (≈ 3.333)
k = 1: 60/19 (≈ 3.158) ≤ √10 ≤ 19/6 (≈ 3.167)
k = 2: 2280/721 (≈ 3.16227) ≤ √10 ≤ 721/228 (≈ 3.16228)
...
At k = 2, we have π ≤ 3.15 < 2280/721 ≤ √10. This means that π < √10.
Note that this isn't meant to be a comparison function that can compute whether number is smaller than another, although it suggests how we could go about writing one. That will come later. At this point, we're just defining what "less than" means for this datatype so that we can write proofs of one real number being less than another in Coq.
This also gives us a definition of "greater than": x is greater than y if y is less than x.
If we've defined "less than" in a sensible way, it should not be possible for a real number x to be both less than and greater than a second real number y. We'll need to prove that this is in fact impossible under our definition. We could do it easily enough with what we have now, but let's first develop some tools that will be helpful in similar proofs.
First we need some notations. It's typical for [a, b] to denote the numbers between a and b, with the endpoints a and b included. We'll use [a, b]Q to denote the rational numbers between a and b inclusive. The symbol "∈" means "in" (or more precisely "is an element of"), so x ∈ [a, b]Q means x is a rational number and a ≤ x ≤ b. We'll use x[k] to denote the rational numbers in the kth (starting at zeroth) interval being used to define the real number x. So for example, if x is the representation of √2 given in
>>8908, then x[3] is the interval of rational numbers [1.414, 1.415]Q. The irrational number x = √2 would not itself be an element of x[3], but the rational numbers 1.414, 1.4145, and 1.415 would be. We'll write min(x[k]) and max(x[k]) to mean the minimum and maximum of x[k], respectively.
Next, we'll observe some facts about x[k] that follow immediately from our requirements in
>>8908. First, the minimum and maximum of x[k] are both elements of x[k], and thus every x[k] is nonempty. Second, if k2 ≥ k1, then any rational number r in x[k2] is also in the prior interval x[k1], because the intervals are nested; min(x[k1]) ≤ min(x[k2]) ≤ r ≤ max(x[k2]) ≤ max(x[k1]).
The following alternate characterization of "less than" will be useful: x is less than y if and only if there exists a k such that every r ∈ x[k] is less than every s ∈ y[k].
Proof:
1. Suppose x < y. By our original definition there is a k such that max(x[k]) < min(y[k]). For any r ∈ x[k] and s ∈ y[k], we have r ≤ max(x[k]) < min(y[k]) ≤ s.
2. Suppose there exists a k such that every r ∈ x[k] is less than every s ∈ y[k]. Let r = max(x[k]) and s = min(y[k]). Then x < y by our original definition.
Finally, time for the main proof:
Let x and y be real numbers. Suppose x < y and x > y. Then there is a k1 such that every r ∈ x[k1] is less than every s ∈ y[k1], and there is a k2 such that every s ∈ y[k2] is less than every r ∈ x[k2]. Let k3 be the larger of k1 and k2, and let r and s be rational numbers in x[k3] and y[k3], respectively. Since k3 ≥ k1 and k3 ≥ k2, r is in both x[k1] and x[k2], and s is in both y[k1] and y[k2]. It follows that r < s and s < r, a contradiction.
No.8914
>>8908>(Although we can't really make an infinite list in a computer program, we can make a list that expands whenever you need an item that hasn't been computed yet.)And here lies the main problem with this concept: it admits that we can't make an infinite list in a computer program, implying that all real numbers can be represented as a finite list for the purpose of this system, which can expand to the real number with further computation.
Since the list contains finite information (characters), it has the same properties as integers and is countable. Indeed, all finite lists can be mapped to an infinite set of unique integers, which is a subset of all integers.
However, according to Cantor's diagonal argument, there exist real numbers that cannot be mapped from the set of all integers, which means these real numbers can't be represented with a finite list. It's impossible to represent these numbers in any computer program that can be possibly written with finite amount of characters.
No.8915
>>8914That is true of course. Only computable numbers, of which there are countably many, can actually be represented in a computer. And we can go further and say that only finitely many of these can be represented in any particular computer. This is not really an issue, though. There's nothing in Coq that assumes that all functions are computable (or at least there's not supposed to be; sometimes proof systems unexpectedly are discovered to be inconsistent). If we want to model the full uncountable real numbers, we can do so in Coq, because we can invoke axioms to assume the existence of noncomputable functions. Without assuming these axioms, whether noncomputable functions exist is left ambiguous.
No.8916
>>8915>Only computable numbers, of which there are countably many, can actually be represented in a computer.self-correction:
Only computable numbers, of which there are countably many, can actually be represented in a computer using this datatype.
No.8917
>>8915>>8916You're not fully addressing this, which applies to all numbers, not just computable ones: It's impossible to represent these numbers in any computer program that can be possibly written with finite amount of characters.
If you want to represent all real numbers with computable and noncomputable functions, you still cannot do some real numbers in finite number of characters, because otherwise it would imply that real numbers are countable.
No.8918
>>8917Invoking axioms to assume the existence of uncountably many functions doesn't mean you represent those functions on the computer. It just means you assume their existence. You have computable functions which you can actually represent and an uncountably many other functions which you can't represent but can still reason about.
No.8919
>>8916>Only computable numbers, of which there are countably many, can actually be represented in a computer using this datatype.Thinking about this a bit more, even this might be an overstatement. But I'm pretty sure that if Coq works as it's supposed to, we can say:
Only computable numbers, of which there are countably many, can actually be represented in a computer using this datatype without invoking axioms.
Maybe there are axioms we can invoke which will allow us to represent certain uncomputable numbers as members of our datatype, such as the limits of Specker sequences.
https://en.wikipedia.org/wiki/Specker_sequenceWell, they definitely exist since we can assume anything as an axiom; the real question is whether they make the system inconsistent. For any proof system strong enough to model the arithmetic of natural numbers, which certainly includes Coq, it is impossible to prove the system's consistency without invoking an even more powerful proof system whose consistency is even more questionable. So we never really know for sure if we have consistency.
If we manage to define and represent some uncomputable numbers as members of our data type by invoking axioms, then we wouldn't be actually be able to do anything computationally with the numbers. The axiom would look like a function we have to run to compute the intervals, but which we can't run because it doesn't have a definition. Of course, we wouldn't be able to define all uncomputable numbers that way. We would have three kinds of numbers:
1. numbers we can represent without axioms, which we can compute
2. numbers which we can represent using additional axioms that don't make our system inconsistent, which we can't compute
3. numbers which we can postulate the existence of using additional axioms that don't make our system inconsistent, which we can't represent but which we can reason about collectively
All would be members of our datatype in an abstract sense, but obviously the numbers in (3) wouldn't exist as actual data.
As for why I added "using this datatype" as the first correction, it's pretty easy to have a datatype in Coq that can represent limits of Specker sequences without invoking any axioms. Just remove the convergence requirement from the current definition, and you get interval sequences whose intersection can have such numbers as their minimum. It doesn't do anything to let us compute those numbers, though.
I'll investigate this more when I get around to working on proofs of completeness properties of real numbers. My plan is to try to prove theorems like "If we assume X axiom, then the least upper bound property holds for this datatype." I'm planning on using on the axioms mentioned in
https://www.researchgate.net/publication/225243273_Sets_in_types_types_in_sets or logical equivalents. These should be relatively safe.
No.8920
Back to properties of "less than." Looking at
>>8913 again, I see that the final proof is very similar to what we need to prove that "less than" is transitive, so here's a revised proof where we prove transitivity first. I'm going to drop the alternate characterization of "less than" because after planning out some of my subsequent proofs it wasn't as useful as I thought it would be.
First, let's prove that for any real number x, all the bounds are consistent; that is, every lower bound min(x[k1]) is less than or equal to every upper bound max(x[k2]).
Proof:
Let k3 be the larger of k1 and k2. Then min(x[k1]) ≤ min(x[k3]) ≤ max(x[k3]) ≤ max(x[k2]).
Now we prove that the "less than" operation we have defined for our real numbers is transitive. "Transitive" means that if x < y and y < z, then x < z.
Proof:
Suppose x < y and y < z. Then there is a k1 such that max(x[k1]) < min(y[k1]) and a k2 such that max(y[k2]) < min(z[k2]). Let k3 be the larger of k1 and k2. Then max(x[k3]) ≤ max(x[k1]) < min(y[k1]) ≤ max(y[k2]) < min(z[k2]) ≤ min(z[k3]). Thus x < z.
(If this seems circular, notice that what we're using is the transitivity of "less than" for rational numbers and what we're proving is the transitivity of "less than" for reals.)
Another important theorem to prove is that no number is less than itself; that is, it is not possible that x < x.
Proof:
Assume to the contrary that x < x. Then there is a k such that max(x[k]) < min(x[k]). This contradicts min(x[k]) ≤ max(x[k]).
Now we will prove that x cannot be both greater than and less than y as a consequence of the previous two theorems.
Proof:
Assume to the contrary that x < y and x > y. Because "less than" is transitive, we have x < x, a contradiction.
No.8921
More stuff about "less than." We want to prove that if x < y, then either z < y or z > x. (The contrapositive of this will turn out to be the transitive property of "less than or equal.")
First, some more lemmas. The width of the intervals (max - min) is decreasing. In other words, if k2 ≥ k1, then max(x[k2]) - min(x[k2]) ≤ max(x[k1]) - min(x[k1]).
Proof:
Let x be a real number and suppose k2 ≥ k1. We have max(x[k2]) ≤ max(x[k1]) and min(x[k2]) ≥ min(x[k1]). Subtracting them, we get the desired result.
[I called this the "length" of the intervals in
>>8908, but I think "width" works better.]
Another lemma: If x < y, then there exists a natural number k and a rational number eps > 0 such that for every n ≥ k, every element of y[n] exceeds every element of x[n] by at least eps; in other words, r ∈ x[n] and s ∈ y[n] imply s - r ≥ eps.
Proof:
Suppose x < y. Then there is a k such that max(x[k]) < min(y[k]). Let eps = min(y[k]) - max(x[k]). Given n ≥ k, r ∈ x[n], and s ∈ y[n], we have r ∈ x[k] and s ∈ y[k]. Subtracting r ≤ max(x[k]) from s ≥ min(y[k]), we have s - r ≥ eps.
Now to prove the main theorem of the post. The idea is that there is a gap between intervals for x and y, and we can find an interval for z that's smaller than the gap, which means it has to be either completely right of the x interval or completely left of the y interval. It's not large enough to overlap both.
eps
x[k1] <--------> y[k1]
[-------------------] [-------------------------------]
[---------------] [---------------------------]
x[k3] [-------] y[k3]
z[k3]
Let x < y. Choose k1 and eps > 0 by the previous lemma. There is a k2 such that the width of z[k2] is less then eps. Let k3 be the larger of k1 and k2; the width of z[k3] will also be less then eps. Compare max(x[k3]) with min(z[k3]). If max(x[k3]) < min(z[k3]), then x < z and we are done. If instead min(z[k3]) ≤ max(x[k3]), then subtracting them from min(y[k3]) gives us min(y[k3]) - min(z[k3]) ≥ min(y[k3]) - max(x[k3]) ≥ eps > max(z[k3]) - min(z[k3]). Therefore min(y[k3]) > max(z[k3]), and y > z.
No.8922
>And to auoide the tediouſe repetition of theſe woordes : is equalle to : I will ſette as I doe often in woorke vſe, a paire of paralleles, or Gemowe lines of one lengthe, thus: =, bicauſe noe .2. thynges, can be moare equalle. And now marke theſe nombers.-- Robert Recorde,
The Whetstone of Witte.
So far, we've defined a type to represent real numbers, and defined what "less than" and "greater than" mean for the type. Now for the rest of the order relations.
The real number x is
apart from y if x is either less than or greater than y. We write x <> y.
The real number x is
equal to y if x is not apart from y. We write x = y.
The real number x is
at most y if x is not greater than y. We write x ≤ y.
The real number x is
at least y if y is at most x, which means that x is not less than y. We write x ≥ y.
Coq already defines "=" and "<>" relations in the standard prelude. But if we used these, we would be saying that the representations of the numbers were equal or different, respectively, when we want to say that the numbers they represent are equal or apart. So to avoid clashes, in the Coq code we will write equals and apart as:
x == y
x =/= yRecall that these properties were proven in
>>8920:
¥ There is no real number x for which x < x.¥ If x < y, then it cannot also be true that y < x.Let's now prove some more basic logical properties of these relations.
¥ For every real number x, x ≤ x. Similarly, x ≥ x.Proof: Since x < x is always false, x ≥ x and x ≤ x are always true.
¥ There is no real number x for which x <> x.Proof: Assume to the contrary that x <> x. Then either x < x or x > x. In both cases we have a contradiction.
¥ For every real number x, x = x.Proof: Since x <> x is always false, x = x is always true.
¥ If x <> y, then y <> x.Proof: Let x <> y. Then x < y, making y > x, or x > y, making y < x. In either case y <> x.
¥ If x = y, then y = x.Proof: Let x = y. Assume to the contrary that y <> x. Then x <> y, a contradiction.
¥ If x < y, then x <> y.¥ If x > y, then x <> y.Proof: These follow immediately from the definition of "apart."
¥ If x < y, then x ≤ y. Similarly, if x > y, then x ≥ y.Proof: Let x < y. Then it cannot also be true that x > y. This means that x ≤ y.
¥ If x = y, then x ≤ y.Proof: Let x = y. Assume to the contrary that x > y. Then x <> y, a contradiction.
¥ If x = y, then x ≥ y.Proof: Let x = y. Assume to the contrary that x < y. Then x <> y, a contradiction.
¥ If x ≤ y and x ≥ y, then x = y. The converse is also true; if x = y, then x ≤ y and x ≥ y.Proof: Let x ≤ y and x ≥ y. Assume to the contrary that x <> y. Then either x < y, contradicting x ≥ y, or x > y, contradicting x ≤ y. For the converse, both conclusions follow from previous theorems.
¥ If x ≤ y and x <> y, then x < y. The converse is also true.Proof: Let x ≤ y and x <> y. Then either x > y, contradicting x ≤ y, or x < y. For the converse, both conclusions follow from previous theorems.
¥ If x ≥ y and x <> y, then x > y. The converse is also true.Proof: Let x ≥ y and x <> y. Then either x < y, contradicting x ≥ y, or x > y. For the converse, both conclusions follow from previous theorems.
No.8925
In
>>8920 we proved that "less than" is transitive, meaning that if x < y and y < z, then x < z. Now we prove the transitive property for some other relations.
¥ If x ≤ y and y ≤ z, then x ≤ z.Proof: Suppose x ≤ y and y ≤ z. Assume to the contrary that z < x. By the theorem in
>>8921, this means that either y < x, contradicting x ≤ y, or y > z, contradicting y ≤ z.
¥ If x < y and y ≤ z, then x < z.Proof: Suppose x < y and y ≤ z. Since x < y, we have either z < y, which contradicts y ≤ z, or z > x.
¥ If x ≤ y and y < z, then x < z.Proof: Suppose x ≤ y and y < z. Sinxe y < z, we have either x < z, in which case we are done, or x > y, which contradicts x ≤ y.
¥ If x = y and y = z, then x = z.Proof: Suppose x = y and y = z. Then we have x ≤ y and y ≤ z, implying x ≤ z. We also have x ≥ y and y ≥ z, implying x ≥ z. Therefore x = z.
No.8927
>>8926How much of this still makes sense? There are a lot of little theorems here, and it's probably a bit tedious to follow every proof, but the definitions are the most important part. Are the definitions of "less than" and "greater than" in
>>8913 clear enough? What about "apart," "equal," "at most," and "at least" in
>>8922?
No.8928
I think if kissu allowed for some math notation it'd be easier to understand some things, but I haven't given this a proper readover yet so I'll do it in the shower and report back with results.
No.8929
>>8927To me personally, not much of it because I hate math and thinking about math. I just took that screenshot with the subs because it made me think of this thread. Sorry if it confused you, it's just meant to be a cute image.
No.8932
>>8913I can understand the proof aspect of this post and that essentially what it boils down to is that you will use the intervals as a point of comparison, and once you can group the irrationals into two disconnected intervals, like [1,2] and [3,4] to name something extremely basic, you can say with confidence that one is larger than the other.
The following argument I'd have to think about a lot harder to come to a conclusion on since taking into account the density of the rationals it should be possible to create an interval for every irrational number such that its endpoints are rationals and the two intervals are separate. Although now that this thought comes to mind I realized what
>>8914 meant since theoretically you'd need an infinite list to find disconnected rational intervals between some irrationals. In practicality I'm not sure where this would come up so it could be something possible to ignore. Maybe that's what you are somewhat arguing but I'd need to familiarize myself more with the axioms you guys brought up. I'll admit some of that discussion is definitely going over my head.
>>8920>>8921>>8922>>8925These made sense. Although my mind started to drone out a bit while reading the long list of logical properties.
No.8934
>>8932Also how are you fitting your infinite sequences to the irrationals automatically? Does it involve Taylor series's, since that seems like it'd make the most sense in terms of what I know about them.
No.8935
>>8934>Also how are you fitting your infinite sequences to the irrationals automatically? Does it involve Taylor series's, since that seems like it'd make the most sense in terms of what I know about them.You can use any function that takes a natural number as input and returns a pair of rational numbers as outputs, provided you can prove it satisfies the conditions at the end of
>>8908. I'll cover some more examples of functions that calculate irrational numbers in future posts. Taylor series are one method we can use to implement these functions.
>>8932Well, what
>>8914 points out is definitely a legitimate limitation. You can't represent every real number on a computer. If you could represent any function from naturals to pairs of rationals you could do it, but you can't do that either. You can prove theorems about those functions even if you can't represent them, though.
No.8936
>>8915After thinking about it some more, I realize that I was wrong about axioms helping to prove that there are uncountably many real numbers. In fact, I'm pretty sure it will be possible to prove the current datatype is uncountable in Coq without adding any axioms at all.
But wait! If we take the meaning of "functions" to be computer programs that we can write down in the language of Coq, they are clearly countable. Every finite computer program can be represented as a natural number, so we have a one-to-one function from functions we can define in Coq to natural numbers. That's true, but we won't be able to define this function within Coq.
Of course, the meaning of "functions" in Coq isn't necessarily restricted to functions we can write down in Coq. That's only one model of Coq's logic. Another model is the one in
https://www.researchgate.net/publication/225243273_Sets_in_types_types_in_sets. This well-known paper models the logic underlying Coq in ZFC. And the meaning of "functions" in that model is this:
>On the predicative level, types are simply interpreted as sets. In particular the function type Πx : A.B is interpreted by the full set of set-theoretic function between the interpretations of A and B.So it seems that in this model, our real number datatype really would be uncountable (at least according to ZFC).
By adding axioms to Coq's logic we can rule out certain models. So can we assume an axiom in Coq that rules out all models where we have only countably many functions without ruling out all models? I think the answer is no.
Let's look at the analogous situation in ZFC. We can construct models of the real numbers in ZFC that ZFC says are uncountable. But is there a model of ZFC in which they really are countable? By putting together
https://en.wikipedia.org/wiki/G%C3%B6del%27s_completeness_theorem and
https://en.wikipedia.org/wiki/L%C3%B6wenheim%E2%80%93Skolem_theorem, we have that either ZFC is inconsistent or it has a finite or countably infinite model. So even though ZFC might tell you your real numbers are uncountable, if ZFC is any good at all, it's possible to reinterpret the idea of "set" in a way that there are only countably many "sets" in your model of ZFC, making the real numbers you've constructed countable too.
Do these same theorems apply to Coq? I'm not enough of an expert in this to say for sure. These are theorems about first-order logic, and Coq is not first-order logic but higher-order logic. But the main obstacle to these theorems in higher-order logic is that they don't work if you assume what's called "full semantics," meaning that you're only allowed to model a functions in higher-order logic as meaning all possible functions in the metatheory you're using to model it. This seems like a stupid restriction to me, and it would rule out the concrete model of Coq as actual ones and zeros in a computer. If we use instead what's called Henkin semantics, then "all possible functions" in the theory we're modeling doesn't have to be interpreted as all possible functions in the metatheory. Henkin semantics more or less reduces higher-order logics to fancy many-sorted first order logics, so I would think a lot of the same theorems would apply, but I could be wrong here.
No.8937
One thing you might ask is whether it makes any sense to just define things to be equal. It can make sense, but we need to do it consistently. What we want to do is break up our representations of real numbers into what are called equivalence classes. All the representations in an equivalence class are said to represent the same number.
To make consistent equivalence classes, we need our "equals" relation to satisfy three properties: reflexivity (x = x), symmetry, (x = y implies y = x), and transitivity (x = y and y = z imply x = z). A relation that has these properties is called an equivalence relation. We have proven these properties in
>>8922 and
>>8925.
Define the equivalence class of x to be the set of representations y such that y = x. We want every representation of a real number to be in exactly one equivalence class, namely its own. If x belongs to the equivalence class of y, then the equivalence classes of x and y need to be the same in the sense that they have exactly the same members.
¥ Every representation x belongs to its own equivalence class.Proof: This is just reflexivity (x = x).
¥ If x belongs to the equivalence class of y, then for every z, z is in the equivalence class of x if and only if it is in the equivalence class of y.Proof: Suppose x is in the equivalence class of y (x = y). We are given a representation z. If z is in the equivalence class of x (z = x), then z is in the equivalence class of y (z = y) by transitivity. If z is in the equivalence class of y (z = y), then we have y = x by symmetry and then z is in the equivalence class of x (z = x) by transitivity.
We can then verify that
¥ x = y if any only if they are members of the same equivalence class.Proof.
1. Suppose x = y. Then x and y are both members of the equivalence class of y, x by the hypothesis and y by reflexivity.
2. Suppose both x and y are members of the equivalence class of z; in other words, x = z and y = z. Then z = y by symmetry and x = y by transitivity.
No.8941
Another thing about our "equals" relation: If x and y represent the same number, it wouldn't make sense for x to be less than z but for y not to be less than z. Our relations and functions should return the same or equivalent results when you substitute equivalent input values. For example, for the "less than" relation, we need to have
if x1 = x2 and y1 = y2, then x1 < y1 if and only if x2 < y2.
A function or relation that works this way is said to be compatible with the equivalence relation. We need to prove that all our relations are compatible with our "equals" relation:
¥ "Less than" (and similarly, "greater than") is compatible with "equal to."
Proof: Suppose x1 = x2 and y1 = y2. If x1 < y1, then x2 ≤ x1 < y1 ≤ y2. If x2 < y2, then x1 ≤ x2 < y2 ≤ y1.
¥ "Apart from" is compatible with "equal to."
Proof: Suppose x1 = x2 and y1 = y2. The statement x1 <> y1 means x1 < y1 or x1 > y1, which is true if and only if x2 < y2 or x2 > y2, or in other words, x2 <> y2.
¥ "At most" (and similarly, "at least") is compatible with "equal to."
Proof: Suppose x1 = x2 and y1 = y2. The statement x1 ≤ y1 means that x1 > y1 is false, which is the case if and only if x2 > y2 is false, or in other words, x2 ≤ y2.
¥ "Equal to" is compatible with itself.
Proof: This is a consequence of it being an equivalence relation. Suppose x1 = x2 and y1 = y2. If x1 = y1, then x2 = x1 = y1 = y2 by symmetry and transitivity. If x2 = y2, then x1 = x2 = y2 = y1, also by symmetry and transitivity.
No.8947
This post might be more confusing than most so please tell me how much of it makes sense.Usually when we prove a theorem, the main thing we care about is whether the theorem is true. But sometimes we can get valuable information from how a theorem is proved. For example, reading a proof of "A or B" might tell us which of "A" or "B" was true in a given situation. And a proof that "there exists an X such that Y" might tell us which "X" we need. Proofs that supply this information are called "constructive" proofs, and proofs that don't are "non-constructive."
By default, Coq requires all proofs be constructive. If we want to write non-constructive proofs, we have to explicitly assume the tools we need for non-constructive proofs as axioms. One of those tools for writing non-constructive proofs is the law of the excluded middle (LEM). It says that for any statement P, we can deduce that P is either true or false. It doesn't tell us whether the statement is true or false, only that it must be one or the other.
To be more specific, Coq's logic without axioms is a form of intuitionistic logic.
https://en.wikipedia.org/wiki/Intuitionistic_logicIntuitionistic logic might seem inconvenient at first, but it offers the practical advantage of letting us more easily make a distinction between constructive and non-constructive proofs. More importantly it also works well with the Curry–Howard correspondence, which we can talk about more later.
https://en.wikipedia.org/wiki/Curry%E2%80%93Howard_correspondenceWe have defined x ≤ y as the opposite of x > y, so you might think that if we have a proof that x ≤ y is false, Coq will let us prove that x > y is true. But this would be a non-constructive proof, because it doesn't tell at what point k in the sequences of intervals we find a min(x[k]) larger than max(y[k]). One way to prove it would be to assume the LEM.
¥ Assuming LEM, if x ≤ y is false, then x > y is true.Proof: Suppose x ≤ y is false. By LEM, either x > y is either true or it is false. If x > y is false, we have x ≤ y, which is a contradiction. Therefore x > y is true.
Similarly, we can prove that
¥ assuming LEM, if x = y is false, then x <> y is true.The proof is pretty much the same.
With LEM, we can go from any proof of "'P is false' is false" to "P is true" even if P is something that we'd normally need constructive details to prove.
Proof: Suppose "P is false" is false. By LEM, either P is either true or it is false. If P is false, we have a contradiction. Therefore P is true.
If you're wondering why we can prove something is false in Coq with a contradiction argument, that's because "P is false" is defined in the logic of Coq as "if P, then a contradiction." Starting from "not P" and arriving at a contradiction would give us only the double negative of P, unless we use LEM as above.
Another case where we can prove a double negative in Coq without axioms, but need an axiom such as the LEM to prove the positive statement is the law of trichotomy:
¥ It is not false that "x < y, x = y, or x > y."Proof: Assume to the contrary that "x < y, x = y, or x > y" is false. We know that x <> y is false, because if x <> y, then either x < y or x > y, either of which would imply "x < y, x = y, or x > y" is true. Thus x = y is true, and so is "x < y, x = y, or x > y," contradicting our assumption it was false.
Why do we expect not to be able to prove trichotomy constructively? Because that would mean that we have a way to compute for any given pair of real numbers whether or not they are equal. Confirming that two numbers are equal would in general require us to compute both numbers to infinite precision. To make the impossibility more clear, we could define a real number in decimal form as follows: It starts with 0.999 and the 9's go on until a given Turing machine halts. Is this number equal to 1? We can't know without solving the halting problem.
If we have a proof that P is not false and a proof that P implies Q, we can prove that Q is not false.
Proof: Suppose that P is not false and that P implies Q. Assume to the contrary that Q is false. Then if P must be false because if it were true, then Q would be true, a contradiction. This contradicts our hypothesis that P is not false.
So facts that follow from the law of trichotomy can also be proven not false, and can be proven true if we assume LEM:
¥ If x ≤ y, it is not false that "x < y or x = y."Proof: Suppose x ≤ y. Then x < y or x = y follows from trichotomy because x ≤ y eliminates the x > y case.
A similar proof holds for
¥ If x ≥ y, it is not false that "x > y or x = y."Another thing we can prove the double negation of is "P or not P" itself.
Proof: Assume to the contrary that "P or not P" is false. Then P must be false, because if it were true, then "P or not P" would follow, contradicting our premise. But P being false also contradicts our premise.
As a result, we have for any real numbers x and y that:
¥ "x < y or x ≥ y" is not false.¥ "x > y or x ≤ y" is not false.¥ "x <> y or x = y" is not false.Of course, if we assume LEM, all three of these are trivially true.
And since x < y implies x ≤ y, and since x = y implies x ≤ y and x ≥ y, we have as a consequences, for any real numbers x and y:
¥ "x ≤ y or x ≥ y" is not false.¥ "x <> y or x ≤ y" is not false.¥ "x <> y or x ≥ y" is not false.Again, true assuming LEM.
No.8950
There's an important connection between proofs and programs called the Curry–Howard correspondence. Many interactive theorem provers including Coq are built around this concept.
https://en.wikipedia.org/wiki/Curry%E2%80%93Howard_correspondenceThe idea is that you can look at the logical connectives in a statement you want to prove and use them to figure out the programming datatype you'll need to represent a proof of the statement. For example, if you want to prove "if A then B," what you need is a function that takes a proof of "A" as input and delivers a proof of "B" as output. If you want to prove "A and B," you need a pair of proofs, one that proves "A" and another that proves "B." To prove "A or B" constructively, you need a tagged union, which means a bit saying whether you've proved A or B, plus the proof of A or proof of B.
There's also a correspondence between the operations on these datatypes and valid steps in a proof. For example, just as you can use "if A then B" and "A" to deduce "B," you can apply a function from A to B to an object of type A to get an object of type B. Proofs written in Coq are programs, and Coq verifies your proof by type-checking your program.
Here are some common logical operations and the datatype for their proofs. The notation |A| means the number of elements of the type A.
logical operation | datatype of proofs | number of elements of datatype
------------------------------+--------------------------------+--------------------------------------
contradiction | empty type | 0
A or B | tagged union | |A| + |B|
A and B | pair | |A| * |B|
if A then B | functions from A to B | |A|^|B| [†]
not A | functions from A to empty type | 0^|A| (1 if |A| = 0, 0 otherwise) [†]
there exists x such that A(x) | pair of x and A(x) | sum over x of |A(x)|
for all x, A(x) | functions from x to A(x) | product over x of |A(x)| [†][†] if we consider functions with equal outputs for equal inputs to be equal themselves (functional extensionality)
Note in particular how the types used to prove "A or B" and "there exists x such that A(x)" contain more information that just whether the statement is true or false. Whereas a proof of "not (not (A or B))" hides this extra information.
No.8957
Now let's write a function that tests whether a number is less than or greater than another. As was mentioned previously, we should not expect to have a good way of comparing real numbers that are equal to each other, since we would need to calculate both numbers to infinite precision to confirm that they are indeed equal. But if we know that two numbers are not equal, we should be able to tell which one is smaller and which one is larger. To enforce this condition, we require a proof of x <> y as one of the arguments of the comparison function.
If we proved x <> y constructively, the details of the proof will already tell us which number is larger and which is smaller. But Coq won't let us use that information. The reason? One of the features of Coq is program extraction, where you can take a program that you've written in Coq and have Coq convert it to a supported language (currently OCaml, Haskell and Scheme). When Coq does this conversion, it strips out all proof objects from the code. So to get a result we can use in an extracted program, we will have to work out which number is the larger and which is the smaller all over again.
The procedure is simply to test each pair of intervals until we find a pair for which one interval's maximum is less than the other's minimum. Then based on which interval is less than the other, we know which number is less than the other.
Coq's standard library supplies a function (constructive_ground_epsilon_nat in Coq.Logic.ConstructiveEpsilon) that tests a property of natural numbers sequentially on 0, 1, 2, and so on until it finds a number that works, at which point it returns the number. The function takes as input three things:
1. The property that we are testing.
2. A procedure that tests whether the property is true or false for any given number, and returns the answer along with proof.
3. A constructive proof that at least one number with the property exists. This assures that the procedure terminates.
For (1), the property we are testing is for a given natural number k, if either max(x[k]) < min(y[k]) or max(y[k]) < min(x[k]).
For (2), we first compare max(x[k]) with min(y[k]); if the first is not smaller, we then compare max(y[k]) with min(x[k]). There's a comparison function for rational numbers in Coq's standard library that provides proof along with its result, so it is easy for us to provide proof of our result.
For (3), we use the proof of x <> y we were provided. We have either x < y, giving us a k such that max(x[k]) < min(y[k]), or x > y, giving us a k such that max(y[k]) < min(x[k]).
In addition to returning which number is less than the other, let's also return a proof. There is a theorem from Coq's standard libary that guarantees that the natural number returned by constructive_ground_epsilon_nat has the property it's supposed to, so we know that either max(x[k]) < min(y[k]) or max(y[k]) < min(x[k]). We compare max(x[k]) with min(y[k]) as before, obtaining either a proof that max(x[k]) < min(y[k]) is true or a proof that it is false. In the first case, we have x < y immediately, and in the second case, since max(x[k]) < min(y[k]) has been contradicted, we must have max(y[k]) < min(x[k]), meaning x > y.
We return our result along with our proof as a tagged union type, but a different tagged union type than the one used for proofs of "or." For this type, only the proofs will be removed upon program extraction, and the boolean result of whether x < y or x > y remains.
No.8959
>>8957The function described here takes a proof of x <> y, meaning "x < y or y < x," and figures out which one is true, with proof. We can easily generalize it to a function taking a proof of "w < x or y < z" and finding one of them which is true, with proof.
This could be useful in conjunction with the theorem "if x < y then z < y or z > x" to compare numbers in an approximate sense when we don't have a proof they're not equal. Specifically, if we want to compare z with x, but don't know anything else about the two numbers, we can find a number y slightly larger than x, and use these tools to figure out whether z < y or z > x. We'll need to define addition first before we can do this, though.
>>8947Another thing I should mention about the law of excluded middle: You might think that removing the law of excluded middle from the usual classical logic to get intuitionistic logic gives you a weaker subset of classical logic that can't prove all the theorems classical logic can. That's one way to think about it. But there's a better way of thinking about it based on something called double-negation translation.
https://en.wikipedia.org/wiki/Double-negation_translationIt has been shown that for any theorem we can prove in classical logic, we can make a translated version by inserting double negations at the appropriate points, and this translated theorem will be provable in intuitionistic logic. There are several examples of double negation translations in
>>8947 where we could prove the original statement only using LEM but could prove the double negated version in Coq without any extra axioms.
Because of double negation translation, we can think of intuitionistic logic not as reducing the theorems that we can prove, but as expanding the theorems we can state. We can take not (not (A or B)) in intuitionistic logic as meaning what plain "or" did in classical logic; that is, we know that one of the two is true, but we don't necessarily know which. Whereas the plain "or" in intuitionistic logic means that we know that one of the two is true, and we know which one. It's a bit like the "or" in "Would you like soup or salad?"; you're not supposed to just answer "Yes."
If you want to learn more about the kind of logic Coq uses, a good place to start would be learning natural deduction.
https://en.wikipedia.org/wiki/Natural_deductionThis is a formal way of expressing a logical argument so it can be checked for validity, like truth tables or various other methods. But it resembles the way people write proofs in ordinary language a lot more than truth tables do. And unlike truth tables, it doesn't automatically presume the law of the excluded middle, so you can use it for either intuitionistic or classical logic depending on whether you allow excluded middle or an equivalent as an axiom / deduction rule.
Here's a free textbook that starts out with an explanation of natural deduction and goes on to explain type theory. The system of type theory described in the book isn't exactly the same as the one used in Coq, but it's similar, so if you understand it, you should be able to go on to understand the system Coq uses.
https://www.cs.kent.ac.uk/people/staff/sjt/TTFP/
No.8961
>>8960Can you give me an example? It's hard to know what you mean by programming stuff since this topic blurs the lines between math and programming.
No.8962
>>8961Looking over it again, I am a bit more confused than I previously thought. So to try and understand if I'm thinking about this right, do you use an external program to find a point at which one max is less than the other min and then feed them into Coq for comparison, or does the Coq function you specified allow you to do the comparison entirely within Coq.
No.8963
>>8962Well, here's the relevant part of the code:
Definition find_lt_witness (w x y z : R) (p : w < x \/ y < z) : nat :=
constructive_ground_epsilon_nat _
(fun k => or_dec (QIlt_dec w.[k] x.[k]) (QIlt_dec y.[k] z.[k]))
(or_ex_ex_or _ _ _ p).
The function constructive_ground_ep
silon_nat from Coq's standard library does the job of iterating through natural numbers. We feed that function as input another function we define whose job it is to be called by constructive_ground_ep
silon_nat with the natural number to be tested and run the test. That's the
(fun k => or_dec (QIlt_dec w.[k] x.[k]) (QIlt_dec y.[k] z.[k]))part. Our function looks up the relevant intervals in the sequences and calls another function QIlt_dec to decide whether the interval w[k] has a maximum less than x[k]'s minimum, and similarly for y[k] and z[k].
Here's QIlt_dec:
Definition QIlt_dec (rs ss : Qinterval) : decidable (rs < ss)%QI :=
match Qlt_le_dec (max rs) (min ss) with
| left p => left p
| right p => right (Qle_not_lt _ _ p)
end.This function calls Qlt_le_dec with the maximum of one interval and the minimum of the other interval as arguments. Qlt_le_dec is another function from the Coq standard library, and it does the actual comparison of the rational numbers.
No.8964
>>8963All of this can be run entirely within Coq.
We can also use Coq's program extraction feature to turn all of this Coq code into OCaml, Haskell or Scheme code. And that code we could run outside Coq.
No.8965
>>8957>>8959We should also show that our comparison function is compatible with equality. That is, if x1 = x2 and y1 = y2, if our comparison function tells us that x1 < y1, it should tell us that x2 < y2, and if it tells us that x1 > y1, it should tell us that x2 > y2.
Proof:
Suppose x1 = x2 and y1 = y2.
Case 1. Suppose our comparison function tells us that x1 < y1. Then x2 < y2 by the compatibility of less than with equals. If our comparison function told us that x2 > y2, that would be a contradiction. So it must tell us that x2 < y2.
Case 2. Suppose our comparison function tells us that x1 > y1. Then x2 > y2 by the compatibility of greater than with equals. If our comparison function told us that x2 < y2, that would be a contradiction. So it must tell us that x2 > y2.
Note that our more general test that tells us which of w < x or y < z is true is not compatible with equality, since it is possible for w < x and y < z to be simultaneously true, in which case the inequality which is selected will depend on how fast the intervals converge. An explicit counterexample will be provided a bit later. Nevertheless the function can still be useful.
No.8966
A question that's natural to ask upon seeing how
>>8957 works is why we can't take a proof of x ≤ y being false and use it to guarantee that a search for k that proves x > y will terminate. Or similarly, why can't we take a proof of not (not (w < x or y < z)) [essentially a non-constructive proof of w < x or y < z] and use the search method to figure out which of w < x or y < z is true constructively.
I don't think there's any way to do this in Coq without axioms, but there's an axiom we can assume that's weaker than the full law of excluded middle, and unlike LEM, keeps all our proofs constructive. It's called Markov's principle and it essentially says that we can do this kind of search. I talked about it a bit during the first pass in
>>7162.
https://en.wikipedia.org/wiki/Markov%27s_principleMore concretly, Markov's principle for any statement P(n) we might make about a natural number, if the following two conditions are met:
1. P is decidable, meaning we have a way to determine whether P(n) is true or false for any given n. In other words, for all n, P(n) or not P(n) with the constructive sense of "or."
2. It is not true that for all natural numbers n, P(n) is false.
then we can prove constructively that there is a natural number n for which P(n) is true. We can find this number by checking the natural numbers one by one until we find it.
The second condition is equivalent to: It is not false that there exists an n such that P(n).
Proof:
a. Suppose it is not true that P(n) is false for all n. Assume to the contrary that it is false that there exists an n such that P(n). Then given any n, we can prove that P(n) is false for that n, since otherwise there would exist an n such that P(n). So it is true that P(n) is false for all n, which contradicts our hypothesis.
b. Suppose it is not false that there exists an n such that P(n). Assume to the contrary that it is true that P(n) is false for all n. Then it must be false that there exists an n such that P(n), since if such an n did exist, we have that P(n) is false for it. This contradicts our hypothesis.
For brevity in what follows, let's say that one rational interval is less than another if the first interval's maximum is less than the second's minimum. In other words, we write [a,b]Q < [c,d]Q if b < c.
We already know that whether one rational interval is less another in this fashion is a decidable question. This shows that
¥ Assuming Markov, if x ≤ y is false, then x > y is true.and similarly
¥ Assuming Markov, if x ≥ y is false, then x < y is true.The meaning of w < x or y < z is that there is either some k such that w[k] < x[k], or some k such that y[k] < z[k]. An equivalent statement is that there is some k such that w[k] < x[k] or y[k] < z[k].
Proof:
a. Suppose there is either some k such that w[k] < x[k], or some k such that y[k] < z[k]. If there is some k such that w[k] < x[k], then for that same k we have w[k] < x[k] or y[k] < z[k]. If there is some k such that y[k] < z[k], then for that same k we have w[k] < x[k] or y[k] < z[k].
b. Suppose there is some k such that w[k] < x[k] or y[k] < z[k]. We either have w[k] < x[k], which we can say is true for this k, or we have y[k] < z[k], which we can say is true for this k.
We can now show that
¥ Assuming Markov, if "w < x or y < z" is not false, then "w < x or y < z" is true.Proof:
Suppose "w < x or y < z" is not false. Then by the equivalence shown above and
>>8947>If we have a proof that P is not false and a proof that P implies Q, we can prove that Q is not false.we have that "there exists a k such that w[k] < x[k] or y[k] < z[k]" is not false. We know how to decide whether w[k] < x[k] and whether y[k] < z[k], so "w[k] < x[k] or y[k] < z[k]" is decidable. By Markov's principle, there exists constructively a k such that w[k] < x[k] or y[k] < z[k].
An immediate consequence is
¥ Assuming Markov, if x = y is false, then x <> y is true.Of course, all of these were also consequences of the law of the excluded middle. If we assume LEM, we can deduce Markov's principle as a theorem.
Proof:
Suppose it is not true that P(n) is false for all natural numbers n. By LEM, either there exists an n such that P(n) or there does not. In the first case, we are done. In the second case, we can show that P(n) is false for all n, because for any n, if P(n) was true, then there would exist an n such that P(n), which is a contradiction.
No.8967
It's time to begin constructing some actual real numbers! The simplest numbers we can construct are the rational numbers. Rational numbers also work as real numbers, so we should have a function that takes objects of rational number type and turns them into their real number type equivalents. Let's call this function Q2R.
First part we need is to decide the sequence of intervals we'll be using. An easy choice is to just make the original rational number both the minimum and maximum of all intervals:
r ≤ Q2R(r) ≤ r, r ≤ Q2R(r) ≤ r, r ≤ Q2R(r) ≤ r, ...
So Q2R(r)[k] = [r, r]Q.
But we're not done yet. In order to make these real numbers we have to prove they satisfy the conditions.
>That the minimum of each interval be less than or equal to the maximum.
Easy: min(Q2R(r)[k]) = r ≤ r = max(Q2R(r)[k])
>That each interval is at least as strict as the intervals before it; the minimums must be greater than or equal to previous minimums, and the maximums must be less than or equal to previous maximums.
Also easy:
min(Q2R(r)[k2]) = r ≥ r = min(Q2R(r)[k1])
max(Q2R(r)[k2]) = r ≤ r = max(Q2R(r)[k1])
>That for any positive rational number, there will eventually be an interval in the sequence whose length (maximum - minimum) is smaller than that number. This will ensure that only one real number meets our infinite list of conditions.
Easy; let k = 0 (any natural number would work). Since eps > 0, we have width(Q2R(r)[k]) = r - r = 0 < eps.
With that out of the way, we need to prove that these numbers have the same order as real numbers that they did as complex numbers.
¥ r < s if and only if Q2R(r) < Q2R(s).
Proof:
1. Suppose r < s. Then max(Q2R(r)[0]) = r < s = min(Q2R(s)[0]), making Q2R(r) < Q2R(s).
2. Suppose Q2R(r) < Q2R(s). Then there is a k such that r = max(Q2R(r)[k]) < min(Q2R(s)[k]) = s.
¥ r > s if and only if Q2R(r) > Q2R(s).
Proof: This is the same statement as the previous with r and s switched.
¥ r <> s if and only if Q2R(r) <> Q2R(s).
Proof:
1. Suppose r <> s. We have r < s, r = s, or r > s, and the r = s case has been eliminated. So r < s or r > s, which is equivalent to Q2R(r) < Q2R(s) or Q2R(r) > Q2R(s). Thus Q2R(r) <> Q2R(s).
2. Suppose Q2R(r) <> Q2R(s). Then Q2R(r) < Q2R(s) or Q2R(r) > Q2R(s), and r < s or r > s. In either case we have r <> s.
¥ r = s if and only if Q2R(r) = Q2R(s).
(In both cases here, "=" represents equality of values, not identical representations. Equivalent fractions should map to representations of equal real numbers.)
Proof:
1. Suppose r = s. This contradicts r <> s being false, which is equivalent to Q2R(r) <> Q2R(s) being false. Thus Q2R(r) = Q2R(s).
2. Suppose Q2R(r) = Q2R(s). Then Q2R(r) <> Q2R(s) is false, which is equivalent to r <> s being false. For rational numbers it is possible to test if two numbers are equal, so even constructively we can say that r = s is true or false. If it's false, we have a contradiction with r <> s being false, so it must be true.
¥ r ≤ s if and only if Q2R(r) ≤ Q2R(s).
Proof: r ≤ s is true if and only if r > s is false, which is equivalent to Q2R(r) > Q2R(s) being false, which is the same as Q2R(r) ≤ Q2R(s).
¥ r ≥ s if and only if Q2R(r) ≥ Q2R(s).
Proof: This is the same statement as the previous with r and s switched.
No.8968
Now that we have our mapping from rationals to reals, we can prove an important theorem: that the bounds we use to define our numbers work as advertised. That is,
¥ Q2R(min(x[k])) ≤ x ≤ Q2R(max(x[k])).Proof:
We are given a real number x and a natural number k.
1. To prove Q2R(min(x[k])) ≤ x, assume to the contrary that Q2R(min(x[k])) > x. Then there is a k2 such that max(Q2R(min(x[k]))[k2]
) > max(x[k2]). Simplifying the left-hand side, we have min(x[k]) > max(x[k2]). This contradicts our lemma from
>>8920 showing the bounds are consistent, or in other words, that min(x[k]) ≤ max(x[k2]).
2. To prove x ≤ Q2R(max(x[k])), assume to the contrary that x > Q2R(max(x[k])). We have a k2 such that min(x[k2]) > max(Q2R(max(x[k])))[k2
] = max(x[k]). This contradicts the same lemma.
No.8969
So far, we have defined real numbers x and y to be equal if x is neither smaller nor larger than y. We now prove an equivalent definition that will be very useful in coming work:
¥ Two real numbers x and y are equal if and only if at every point k in the sequences of intervals that define them, there is a rational number common between the two intervals. In other words, for every natural k there is a rational r such that both r ∈ x[k] and r ∈ y[k].
Proof:
1. Suppose x = y. Given a natural number k, let r be the larger of min(x[k]) and min(y[k]). Clearly r ≥ min(x[k]) and r ≥ min(y[k]). Either r = min(x[k]) or r = min(y[k]). If r = min(x[k]), then we have r = min(x[k]) ≤ max(x[k]) from our conditions for real numbers, and we have r ≤ max(y[k]) because min(x[k]) = r > max(y[k]) would imply x > y, contradicting x = y. Similarly, if r = min(y[k]), we have r = min(y[k]) ≤ max(y[k]) from our conditions for real numbers, and we have r ≤ max(x[k]) since min(y[k]) = r > max(x[k]) would imply y > x, contradicting x = y. Thus r ∈ x[k] and r ∈ y[k].
2. Suppose that for any natural number k, there is an r such that r ∈ x[k] and r ∈ y[k]. To prove x = y, assume to the contrary that x <> y. Then either x < y or x > y. If x < y, then there is a k such that max(x[k]) < min(y[k]), and we have r ≤ max(x[k]) < min(y[k]) ≤ r, a contradiction. Similarly, if x > y, we have r ≤ max(y[k]) < min(x[k]) ≤ r, also a contradiction. Thus x = y.
No.8970
Just noticed a way that my current design can still have exponential blowup. I'll talk about the details more later. I'm going to try the optimizing layer idea to fix it. Not sure exactly what I'll put in the optimizing layer to solve the problem yet. I'll experiment with a few things after there's enough functions implemented that I can test what works better in practice.
The way I think I'll do it is have both a make_R_raw, and a make_R, where the raw version is the actual constructor, and the make_R is what you would use in practice to get the optimizations. A theorem would be proven stating that the results of make_R and make_R_raw are equal.
I'm going to move the expanding list structure into make_R, and I think I'll also change it to use the memoization function from the Coq standard library instead of working with the Stream type directly. For now I'll have it just do that and reducing fractions.
No.8971
>>8970Actually, never mind, turns out I can't move memoization into make_R because if I want to define sequences recursively the generating function has to know about the Stream so it can fetch memoized values instead of calling itself unmemoized.
I'll worry about the optimization issue later. Maybe I'll just have one or more optimize() functions that can be called as needed instead of building it in. This design does need to be simple more than it needs to be fast. If I want fast, I should probably be using a base-n approach, which I may work on after this one is finished.
No.8972
Back to math. Let's define
¥ [a, b]Q + [c, d]Q = [a + c, b + d]Q.The idea behind this definition is that
¥ If r ∈ [a, b]Q and s ∈ [c, d]Q, then r + s ∈ [a, b]Q + [c, d]Q.Proof: Given r ∈ [a, b]Q and s ∈ [c, d]Q, a ≤ r ≤ b and c ≤ s ≤ d. We can add the inequalities to get a + c ≤ r + s ≤ b + d. So r + s ∈ [a + c, b + d]Q.
To add two real numbers, we will simply add their intervals:
¥ (x + y)[k] = x[k] + y[k]For this to be a real number, we must satisfy the conditions.
>That the minimum of each interval be less than or equal to the maximum.Since min(x[k]) ≤ max(x[k]) and min(y[k]) ≤ max(y[k]), min((x+y)[k]) = min(x[k]) + min(y[k]) ≤ max(x[k]) + max(y[k]) = max((x+y)[k]).
>That each interval is at least as strict as the intervals before it; the minimums must be greater than or equal to previous minimums, and the maximums must be less than or equal to previous maximums.Suppose k2 ≥ k1. Since min(x)[k2] ≥ min(x)[k1] and min(y)[k2] ≥ min(y)[k1], we have min((x+y)[k2]) = min(x)[k2] + min(y)[k2] ≥ min(x)[k1] + min(y)[k1] = min((x+y)[k1]). Similarly, because max(x)[k2] ≤ max(x)[k1] and max(y)[k2] ≤ max(y)[k1], we have max((x+y)[k2]) = max(x)[k2] + max(y)[k2] ≤ max(x)[k1] + max(y)[k1] = max((x+y)[k1]).
>That for any positive rational number, there will eventually be an interval in the sequence whose length width (maximum - minimum) is smaller than that number.Let eps > 0. Then eps/2 > 0. There is a k1 such that width(x[k1]) < eps/2, and a k2 such that width(y[k2]) < eps/2. Let k3 be the larger of the two; then width(x[k3]) < eps/2 and width(y[k3]) < eps/2. Thus width((x+y)[k3]) = max((x+y)[k3]) - min((x+y)[k3]) = (max(x[k3]) + max(y[k3])) - (min(x[k3]) + min(y[k3])) = (max(x[k3]) - min(x[k3])) + (max(y[k3]) - min(y[k3])) = width(x[k3]) + width(y[k3]) < eps/2 + eps/2 = eps.
We also need to show that this addition function is compatible with equality; that is, that
¥ If x1 = x2 and y1 = y2, then x1 + y1 = x2 + y2.Let k be any natural number. By
>>8969 there is a rational r such that r ∈ x1[k] and r ∈ x2[k], and a rational s such that s ∈ y1[k] and s ∈ y2[k]. We then have r + s ∈ x1[k] + y1[k] = (x1 + y1)[k], and similarly r + s ∈ x2[k] + y2[k] = (x2 + y2)[k]. Therefore x1 + y1 = x2 + y2.
No.8973
It's a trivial consequence of the first lemma in
>>8972, but it's a useful shortcut to have proven
¥ If r ∈ x[k] and s ∈ y[k], then r + s ∈ (x + y)[k].We can prove a similar thing for Q2R, following from r ≤ r ≤ r:
¥ r ∈ Q2R(r)[k]Now time to prove algebraic properties of real number addition. These follow relatively simply from the same properties holding for rational numbers.
¥ Addition is commutative: x + y = y + x.Given any natural k, let r = min(x[k]) and s = min(y[k]). We have r ∈ x[k] and s ∈ y[k], so r + s ∈ (x + y)[k] and r + s = s + r ∈ (y + x)[k]. Therefore x + y = y + x.
¥ Addition is associative: (x + y) + z = x + (y + z).Given any natural k, let r = min(x[k]), s = min(y[k]), and t = min(z[k]). We have r ∈ x[k], s ∈ y[k], and t ∈ z[k]. Thus r + s ∈ (x + y)[k] and (r + s) + t ∈ ((x + y) + z)[k]. Similarly s + t ∈ (y + z)[k] and (r + s) + t = r + (s + t) ∈ (x + (y + z))[k]. Therefore (x + y) + z = x + (y + z).
We will write 0Q where it is necessary to distinguish it from the real number. We have 0 = Q2R(0Q).
¥ Addition has an identity: x + 0 = x.Given any natural k, let r = min(x[k]). We have r ∈ x[k] and 0Q ∈ 0[k]. Thus r = r + 0Q ∈ (x + 0)[k]. Therefore x + 0 = x.
This with the commutative property gives us
¥ 0 + x = x.Speaking of Q2R, let's not forget to confirm that adding two rational numbers as real numbers gives the correct result:
¥ Q2R(r + s) = Q2R(r) + Q2R(s)Given any natural k, we have r ∈ Q2R(r)[k], s ∈ Q2R(s)[k], and r + s ∈ Q2R(r + s)[k]. It follows that r + s ∈ (Q2R(r) + Q2R(s))[k]. Therefore Q2R(r + s) = Q2R(r) + Q2R(s).
No.8975
Subtraction comes next. But first we need to define additive inverses.
Let us first define
¥ -[a, b]Q = [-b, -a]Q.We have
¥ If r ∈ [a, b]Q, then -r ∈ -[a, b]Q.Proof: Since a ≤ r ≤ b, -b ≤ -r ≤ -a.
We can now define the additive inverse of a number as inverting all the intervals:
¥ (-x)[k] = -x[k].Proving that this is a real number:
>That the minimum of each interval be less than or equal to the maximum.Since min(x[k]) ≤ max(x[k]), we have min((-x)[k]) = -max(x[k]) ≤ -min(x[k]) = max((-x)[k]).
>That each interval is at least as strict as the intervals before it; the minimums must be greater than or equal to previous minimums, and the maximums must be less than or equal to previous maximums.Suppose k2 ≥ k1.
Since max(x[k2]) ≤ max(x[k1]), min((-x)[k2]) = -max(x[k2]) ≥ -max(x[k1]) = min((-x)[k1]).
Since min(x[k2]) ≥ min(x[k1]), max((-x)[k2]) = -min(x[k2]) ≤ -min(x[k1]) = max((-x)[k1]).
>That for any positive rational number, there will eventually be an interval in the sequence whose width (maximum - minimum) is smaller than that number.Let eps > 0. There exists a k such that width(x[k]) < eps. Therefore width((-x)[k]) = max((-x)[k]) - min((-x)[k]) = (-min(x[k])) - (-max(x[k])) = max(x[k]) - min(x[k]) = width(x[k]) < eps.
From r ∈ [a, b]Q, then -r ∈ -[a, b]Q it follows that
¥ If r ∈ x[k], then -r ∈ (-x)[k].The additive inverse is compatible with equality:
¥ If x1 = x2, then -x1 = -x2.Given a natural number k, there is a rational r such that r ∈ x1[k] and r ∈ x2[k]. Thus -r ∈ (-x1)[k] and -r ∈ (-x2)[k]. It follows that -x1 = -x2.
The additive inverse is compatible with the mapping from the rationals:
¥ Q2R(-r) = -Q2R(r)Given k, we have r ∈ Q2R(r)[k] and -r ∈ Q2R(-r)[k]. Thus -r ∈ (-Q2R(r))[k]. We conclude Q2R(-r) = -Q2R(r).
Our additive inverse is an additive inverse:
¥ x + (-x) = 0Given k, let r = min(x[k]). Since r ∈ x[k], we have -r ∈ (-x)[k], and 0Q = r + (-r) ∈ (x + (-x))[k]. Combining this with 0Q ∈ 0[k], we have x + (-x) = 0.
This is also true by commutativity of addition:
¥ (-x) + x = 0Taking the additive inverse twice gets you back the same number:
¥ -(-x) = xGiven k, let r = min(x[k]). Since r ∈ x[k], we have -r ∈ (-x)[k], and r = -(-r) ∈ (-(-x))[k]. Therefore -(-x) = x.
The additive inverse distributes over addition:
¥ -(x + y) = (-x) + (-y)Given k, let r = min(x[k]) and s = min(y[k]). We have r ∈ x[k] and s ∈ y[k]. From r + s ∈ (x + y)[k] we have -(r + s) ∈ (-(x + y))[k]; similarly, from -r ∈ (-x)[k] and -s ∈ (-y)[k] we have -(r + s) = (-r) + (-s) ∈ ((-x) + (-y))[k]. Therefore -(x + y) = (-x) + (-y).
You might notice a lot of the proofs in this post and
>>8973 use basically the same method over and over again, turning facts about the rationals into equivalent facts about the reals. There's probably some way to automate these proofs. I'll have to think about how to do it.
No.8978
>>8977very distracting image
No.8983
>>8975>You might notice a lot of the proofs in this post and >>8973 use basically the same method over and over again, turning facts about the rationals into equivalent facts about the reals. There's probably some way to automate these proofs. I'll have to think about how to do it.It's automated now. You can write tactics in Coq which are basically instructions for how to attack a proof.
The new tactic uses this new lemma:
¥ If for every k there exist rational numbers r ∈ x[k] and s ∈ y[k] such that r = s, then x = y.This is just a slight restatement of the second part of
>>8969. But it helps the machinery figure out that it needs to look for a proof that two rational numbers are equal. The tactic figures out which rational numbers it needs to prove equal by what it has to do to prove r ∈ x[k] and s ∈ y[k].
This additional lemma helps the tactic work on equations involving subtraction:
¥ If r ∈ x[k] and s ∈ y[k], then r - s ∈ (x - y)[k].This follows from the definition of subtraction and the similar lemmas for addition and additive inverses.
No.8984
There are also some theorems we want to prove relating addition to the order relations.
Let's start out with this one:
¥ If x + z < y + z, then x < y.
Suppose x + z < y + z. Then there is a k such that max((x + z)[k]) < min((y + z)[k]). Since max(x[k]) + min(z[k]) ≤ max(x[k]) + max(z[k]) = max((x + z)[k]) < min((y + z)[k]) = min(y[k]) + min(z[k]), we have max(x)[k] < min(y)[k]. Therefore x < y.
A corrolary is that
¥ If x < y, then x + z < y + z.
Suppose x < y. Then (x + z) + (-z) < (y + z) + (-z), implying x + z < y + z.
Combined:
¥ x < y if and only if x + z < y + z.
Given this equivalence and the fact we have defined the other order relations in terms of "less than," we have:
¥ x ≤ y if and only if x + z ≤ y + z.
¥ x <> y if and only if x + z <> y + z.
¥ x = y if and only if x + z = y + z.
By the commutative property, all of these also hold on the left:
¥ x < y if and only if z + x < z + y.
¥ x ≤ y if and only if z + x ≤ z + y.
¥ x <> y if and only if z + x <> z + y.
¥ x = y if and only if z + x = z + y.
By applying these theorems twice, we can add inequalities:
¥ If w < x and y < z, then w + y < x + z. [Proof: w + y < x + y < x + z]
¥ If w < x and y ≤ z, then w + y < x + z. [Proof: w + y < x + y ≤ x + z]
¥ If w ≤ x and y < z, then w + y < x + z. [Proof: w + y ≤ x + y < x + z]
¥ If w ≤ x and y ≤ z, then w + y ≤ x + z. [Proof: w + y ≤ x + y ≤ x + z]
Another theorem we want, relating additive inverses to inequalities is that
¥ If x < y, then -x > -y.
Suppose x < y. Then there is a k such that max(x)[k] < min(y)[k]. Thus min(-x)[k] = -max(x)[k] > -min(y)[k] = max(-y)[k], and we conclude -x > -y.
It works in the other direction too:
¥ If -x < -y, then x > y.
This is because x = -(-x) > -(-y) = y.
So we can write that
¥ x < y if and only if -x > -y.
Using this equivalence and the definitions of the other relation operators we can easily show:
¥ x ≤ y if and only if -x ≥ -y.
¥ x <> y if and only if -x <> -y.
¥ x = y if and only if -x = -y.
No.8990
While working on multiplication I noticed that the first two conditions for our real numbers to satisfy from
>>8908> That the minimum of each interval be less than or equal to the maximum.> That each interval is at least as strict as the intervals before it; the minimums must be greater than or equal to previous minimums, and the maximums must be less than or equal to previous maximums.can be combined into one criterion in a nice way:
¥ That each interval contains the endpoints of itself and all subsequent intervals. That is, if k2 ≥ k1, then min(x[k2]) ∈ x[k1] and max(x[k2]) ∈ x[k1].This implies the first old criterion because k1 ≥ k1, and part of min(x[k1]) ∈ x[k1] is min(x[k1]) ≤ max(x[k1]). It's easy to see it also implies min(x[k2]) ≥ min(x[k1]) and max(x[k2]) ≤ max(x[k1]) when k2 ≥ k1. Going the opposite direction, the min(x[k1]) ≤ min(x[k2]) and max(x[k2]) ≤ max(x[k1]) parts follow from the original second criterion, and the min(x[k2]) ≤ max(x[k1]) and min(x[k1]) ≤ max(x[k2]) parts follow from the consistency of bounds lemma proven in
>>8920. So this new criterion is logically equivalent to the ones it would replace.
Let's replace the first two criteria with this one criterion and see if it makes the things we have to prove simpler or more complex.
The bounds consistency lemma from
>>8920>every lower bound min(x[k1]) is less than or equal to every upper bound max(x[k2])can be proven as follows:
Either k2 ≥ k1, implying max(x[k2]) ∈ x[k1], or k1 ≥ k2, implying min(x[k1]) ∈ x[k2]. In either case min(x[k1]) ≤ max(x[k2]). That's a bit wordier, but conceptually simpler, I think.
>the minimum and maximum of x[k] are both elements of x[k]This is just the k2 = k1 case of the new criterion.
¥ Q2R meets the new criterion.min(Q2R(r)[k2]) = r ∈ [r, r]Q
max(Q2R(r)[k2]) = r ∈ [r, r]Q
¥ x + y meets the new criterion.Suppose k2 ≥ k1. Since min(x)[k2] ∈ x[k1] and min(y)[k2] ∈ y[k1], we have min((x+y)[k2]) = min(x)[k2] + min(y)[k2] ∈ x[k1] + y[k1] = (x+y)[k1]. Similarly, because max(x)[k2] ∈ x[k1] and max(y)[k2] ∈ y[k1], we have max((x+y)[k2]) = max(x)[k2] + max(y)[k2] ∈ x[k1] + y[k1] = (x+y)[k1].
¥ -x meets the new criterion.Suppose k2 ≥ k1.
Since max(x[k2]) ∈ x[k1], min((-x)[k2]) = -max(x[k2]) ∈ -(x[k1]) = (-x)[k1].
Since min(x[k2]) ∈ x[k1], max((-x)[k2]) = -min(x[k2]) ∈ -(x[k1]) = (-x)[k1].
Overall, not much difference besides the fact that there's one less criterion to prove. So let's go ahead and use this as the new definition. We'll keep the first of the old criteria (minimum less than or equal to maximum) around as a convenient lemma.
No.8991
Next is multiplication!
First, let's prove a simple result about rational numbers:
¥ If a ≤ r ≤ b, then min {a*c, b*c} ≤ r*c ≤ max {a*c, b*c}.
Proof: Either c ≥ 0 and min {a*c, b*c} ≤ a*c ≤ r*c ≤ b*c ≤ max {a*c, b*c}, or c ≤ 0 and min {a*c, b*c} ≤ b*c ≤ r*c ≤ a*c ≤ max {a*c, b*c}.
We'll define
¥ [a, b]Q * [c, d]Q = [min {a*c, a*d, b*c, b*d}, max {a*c, a*d, b*c, b*d}]Q.
As usual we want to prove
¥ If r ∈ [a, b]Q and s ∈ [c, d]Q, then r * s ∈ [a, b]Q * [c, d]Q.
Suppose a ≤ r ≤ b and c ≤ s ≤ d. We have
min {a*s, b*s} ≤ r*s ≤ max {a*s, b*s}.
and also
min {a*c, a*d} ≤ a*s ≤ max {a*c, a*d}
min {b*c, b*d} ≤ b*s ≤ max {b*c, b*d}.
Therefore
min {a*c, a*d, b*c, b*d} ≤ min {a*s, b*s} ≤ r*s ≤ max {a*s, b*s} ≤ max {a*c, a*d, b*c, b*d}
which means r*s ∈ [a, b]Q * [c, d]Q.
Let's also prove that
¥ Whenever [a, b]Q and [c, d]Q are nonempty (that is, a ≤ b and c ≤ d):
¥ There exists an r ∈ [a, b]Q and s ∈ [c, d]Q such that min([a, b]Q * [c, d]Q) = r*s.
¥ There exists an r ∈ [a, b]Q and s ∈ [c, d]Q such that max([a, b]Q * [c, d]Q) = r*s.
This follows pretty easily from the formula. The minimum and maximum are each members of {a*c, a*d, b*c, b*d}, each of which are obtained by multiplying a member of [a, b]Q with a member of [c, d]Q.
Next we define
¥ (x * y)[k] = x[k] * y[k]
and establish that it meets our conditions to be a real number.
>That each interval contains the endpoints of itself and all subsequent intervals. That is, if k2 ≥ k1, then min(x[k2]) ∈ x[k1] and max(x[k2]) ∈ x[k1].
Suppose k2 ≥ k1. We have min((x*y)[k2]) = r*s for some r ∈ x[k2] and s ∈ y[k2]. It follows that r ∈ x[k1] and s ∈ y[k1], implying r*s ∈ (x*y)[k1]. The same reasoning holds for max((x*y)[k2]).
>That for any positive rational number, there will eventually be an interval in the sequence whose width (maximum - minimum) is smaller than that number.
This one is a bit more work.
Let's first prove a useful lemma:
¥ If r1, r2 ∈ [a, b]Q, then |r2 - r1| ≤ width([a, b]Q).
We are given a ≤ r1 ≤ b and a ≤ r2 ≤ b. Either r2 - r1 ≥ 0 or r2 - r1 ≤ 0. In the first case, subtract r2 ≤ b and r1 ≥ a; we obtain |r2 - r1| = r2 - r1 ≤ b - a = width([a, b]Q). In the second case, subtract r1 ≤ b and r2 ≥ a; we obtain |r2 - r1| = r1 - r2 ≤ b - a = width([a, b]Q).
Another useful lemma:
¥ If r ∈ [a, b]Q, then |r| ≤ max{|a|, |b|}.
Suppose a ≤ r ≤ b. Either r ≥ 0 or r ≤ 0. If r ≥ 0, then 0 ≤ r ≤ b and |r| = r ≤ b = |b|. If r ≤ 0, then a ≤ r ≤ 0, and |r| = -r ≤ -a = |a|. In either case, |r| ≤ max{|a|, |b|}.
Now back to the main proof. We are given a rational number eps > 0. Let deltaX = (eps/2) / max{|min(y[0])|, |max(y[0])|, 1} and deltaY = (eps/2) / max{|min(x[0])|, |max(x[0])|, 1}. Since deltaX > 0, there is a k1 such that width(x[k1]) < deltaX, and since deltaY > 0, there is a k2 such that width(y[k2]) < deltaY. Let k3 be the larger of the two; then width(x[k3]) < deltaX and width(y[k3]) < deltaY. There exist r1 ∈ x[k3] and s1 ∈ y[k3] such that min((x*y)[k3]) = r1*s1. Similarly there exist r2 ∈ x[k3] and s2 ∈ y[k3] such that max((x*y)[k3]) = r2*s2. We can rewrite width((x*y)[k3]) as
width((x*y)[k3])
= r2*s2 - r1*s2
= (r2*s2 - r1*s2) + (r1*s2 - r1*s1)
= s2 * (r2 - r1) + r1 * (s2 - s1).
Because s2 ∈ y[k3] ⊆ y[0] ("⊆" meaning "is a subset of"), we have |s2| ≤ max{|min(y[0])|, |max(y[0])|} ≤ max{|min(y[0])|, |max(y[0])|, 1}. We also have |r2 - r1| ≤ width(x[k3]) < deltaX. It follows that
s2 * (r2 - r1)
≤ |s2 * (r2 - r1)|
= |s2| * |r2 - r1|
≤ max{|min(y[0])|, |max(y[0])|, 1} * |r2 - r1|
< max{|min(y[0])|, |max(y[0])|, 1} * deltaX
= eps/2.
Similarly, because r1 ∈ x[k3] ⊆ x[0], we have |r1| ≤ max{|min(x[0])|, |max(x[0])|, 1}. Also |s2 - s1| ≤ width(y[k3]) < deltaY. We obtain
r1 * (s2 - s1)
≤ |r1 * (s2 - s1)|
= |r1| * |s2 - s1|
≤ max{|min(x[0])|, |max(x[0])|, 1} * |s2 - s1|
< max{|min(x[0])|, |max(x[0])|, 1} * deltaY
= eps/2.
Combining these, we obtain
width((x*y)[k3])
= s2 * (r2 - r1) + r1 * (s2 - s1).
< eps/2 + eps/2
= eps.
This completes the proof that x * y represents a real number.
From the fact that r ∈ [a, b]Q and s ∈ [c, d]Q imply r * s ∈ [a, b]Q * [c, d]Q, it follows that
¥ If r ∈ x[k] and s ∈ y[k], then r * s ∈ (x * y)[k].
Multiplication as we have defined it is compatible with equality:
¥ If x1 = x2 and y1 = y2, then x1 * y1 = x2 * y2.
Given a natural number k, there is a rational r such that r ∈ x1[k] and r ∈ x2[k], and a rational s such that s ∈ y1[k] and s ∈ y2[k]. Thus r*s ∈ (x1*y1)[k] and r*s ∈ (x2*y2)[k]. We conclude x1*y1 = x2*y2.
No.8992
Now for a big list of properties of multiplication. All of these theorems can be proven in a mechanical fashion from the corresponding theorems for rational numbers by the same method used to prove most of the theorems in
>>8973 and
>>8975. Basically the idea is that for each variable or constant in the equation, you get a sequence of rational numbers by taking the minimums of each interval. Then you perform the operations that appear in the equation termwise on those sequences. For example, if one of the steps in the equation is to add two real numbers, we add the kth rational numbers in the sequences to get the kth rational number in a new sequence. At the end we have two sequences, guaranteed to be equal to each other by the fact that the equation we are trying to prove for the real numbers holds for the rational numbers. By the way we have defined our operations on real numbers, each kth term in the sequence corresponding to the left-hand side is guaranteed to be a member of the kth interval defining the real number on the left-hand side of the equation. The same goes for the right-hand side. Since at each step, the intervals on the left and right sides share a common rational number, the real numbers are equal by the lemma in
>>8969.
Multiplication is compatible with the mapping from the rationals.
¥ Q2R(r * s) = Q2R(r) * Q2R(s)Multiplication is commutative.
¥ x * y = y * xMultiplication is associative.
¥ (x * y) * z = x * (y * z)Multiplication is distributive over addition and subtraction.
¥ x * (y + z) = x * y + x * z¥ (x + y) * z = x * z + y * z¥ x * (y - z) = x * y - x * z¥ (x - y) * z = x * z - y * zMultiplying by 1 gives you the original number back.
¥ x * 1 = x¥ 1 * x = xMultiplying by 0 gives you 0.
¥ x * 0 = 0¥ 0 * x = 0Multiplying by -1 gives you the additive inverse.
¥ x * (-1) = -x¥ (-1) * x = -xMultiplying by the additive inverse of a number gives you the additive inverse of the original product.
¥ x * (-y) = -(x * y)¥ (-x) * y = -(x * y)
No.9000
>>8999Well, it's a combination of things. First, there are a few of these properties which lots of people have memorized, namely the field axioms.
¥ addition and multiplication are commutative¥ addition and multiplication associative¥ addition and multiplication have identities¥ additive and multiplicative inverses exist¥ multiplication is distributive over additionTack on a few extra axioms for the "less than" relation, and you have a complete set of axioms for the real numbers. So we obviously need those ones. Once we have them we can prove anything we want about the real numbers, but not necessarily conveniently.
Second, there are properties I expect I might need for proofs in the future. For some of these theorems, I've had to use the corresponding theorem for rational numbers repeatedly.
I would say most of the work here, especially on the Coq side, is in the harder theorems. Proving that that the intervals that come out of the multiplication algorithm in
>>8991 converge and therefore represent a real number was a fair amount of work. The list in
>>8992 was very little work at all. Those ones were proven entirely mechanically. I literally just wrote what I was proving and copypasted "Proof. fromQ. Qed." after it.
The main reason for writing out these long lists of theorems is in the nature of mechanical proof checking. To prove something to a machine, I have to spell out every minor step, either by hand or by some algorithm that automates the proofs. It's annoying to be working on some more challenging proof, and to have to do a bunch of extra steps because some obvious result doesn't exist as a theorem in the system. So if I think of a result that I might use in the future, and it's easy to prove, I'll prove it.
No.9001
>>8999As for complex numbers, I'll probably introduce them when I start doing trig stuff. Complex numbers are not a source of trouble that we grudgingly have to deal with. They are a wonderful tool that makes trigonometry easier.
Eventually I'd like to prove the fundamental theorem of algebra constructively (meaning having an algorithm that solves any polynomial equation), but that's probably a long way off.
No.9012
Here's a puzzle that came up while I was thinking about this. You may be familiar with the halting theorem, the problem of determining if a computer program halts or runs forever. It's well known that it is impossible to write an algorithm which answers this question in the general case.
https://en.wikipedia.org/wiki/Halting_problemSuppose we have two computer programs, A and B, both written in some Turing-complete programming language. Suppose also that we know that at least one of the programs (possibly both) does not halt. Is it possible to write an algorithm that correctly concludes, in a finite amount of time, that "A does not halt" or that "B does not halt"? If it is impossible, is there a proof that it is impossible?
No.9013
>>9012>Is it possible to write an algorithm that correctly concludes, in a finite amount of time, that "A does not halt" or that "B does not halt"?This reminds me heavily of convergent and divergent series. A convergent series will converge to a point whereas a divergent series will not. In effect, you could imagine a program that halts as being convergent, while a program that does not halt as being divergent. Although most of the ways for solving a series are not applicable in this scenario, I feel, there is one simple theorem -- although I can't remember what it is called exactly -- that fits this scenario very well: Suppose you have a series, Σ(a-1) from n=0 -> n=∞, if the series, Σ(a) from n=0 -> n=∞, converges then Σ(a-1) from n=0 -> n=∞ must converge because Σ(a-1) from n=0 -> n=∞ is contained within Σ(a) from n=0 -> n=∞.
No.9014
>>9012>Suppose also that we know that at least one of the programs (possibly both) does not halthow do we know? through an oracle?
No.9015
>>9014Perhaps, or maybe someone has come up with a clever proof.
The motivating problem is that I'm wondering if given x*y = 0 there is an algorithm which can decide that x = 0 or decide that y = 0. Given a program, we can construct a number as the intersection of the intervals
[0, 1], [0, 1/2], [0, 1/3], ...
for a program that continues indefinitely and
[0, 1], [0, 1/2], [0, 1/3], ..., [0, 1/(k-1)], [1/k, 1/k], [1/k, 1/k], [1/k, 1/k], ...
for a program that halts after k steps. This sort of construction has been useful for impossibility proofs before, namely to prove the undecidability of equality testing in
>>8947.
No.9018
>>9012>>9015Ok then, suppose we have the algorithm in question.
Then, we input two programs A and B, for which some oracle has told us that atleast one of them doesn't halt. We know nothing else of these programs.
The following scenario must be considered.
A doesn't halt, B doesn't halt.
I don't see how deciding this is any different from deciding the halting problem. But my reduction skills are lacking so I won't attempt to prove it.
No.9019
>>9018i was thinking about this problem too, and the only thing I got is that if we know one of them halts then we can just wait and then declare the other one as the non-halting one and itll be technically in finite time
but that just seems trivial
No.9020
>>9019it is trivial, but how is that a problem?
The same way is argued for the semidecidability of the halting problem.
No.9034
What wording would you use to describe a property which, being true for some natural number k, must be true for all subsequent natural numbers? The concept seems to come up a lot in this.
No.9035
>>9034Isn't that the principle of induction, or something related to it?
No.9037
>>9035That's a way of proving things. I'm talking about describing something already known. To give a specific example, one of the requirements chosen for x to represent a real number is that for any rational number eps > 0 there be a k such that width(x[k]) [the width of the kth interval being used to define x] is less then eps. But it's not just one k for which this is true; we can prove that since the widths of the intervals are decreasing, if it's true for some k, it's true for all k that come after.
Ideas for words to describe this property: increasingly true, increasing, monotone, monotonic, isotone, isotonic, weakening, upward, true upward, onward, true onward, expanding, improving, permanent, once-always
No.9444
Haven't done much with the code lately, but I've been working out how to do arithmetic with infinite (signed-digit) decimals.
. 5 2 5 2 5
---------------------------
±10 ±20 ±30 ±40 ±50 ±60 ±70
._
. . . . 510
0 42 510 232 500 222 490
. 0 42 90 132 180 222 270
---------------------------
× . 7 8 7 8 7 8
---------------------------
.6| 42 48 42 48 42 48
6| 42 48 42 48 42
6| 42 48 42 48
6| 42 48 42
6| 42 48
6| 42
(there seems to be a bug in the way the 2/22/22 thing interacts with code blocks)
No.9460
I've started to work on the code a bit again. So far I've added a few more useful theorems, some of which I plan to use to prove important stuff about multiplication and inequalities.
>>8920The following will be a common pattern, so it will also be convenient to prove as a lemma so we can repeat things less often:
¥ If a < b and c < d, then there is a natural number k such that a[k] < b[k] and c[k] < d[k]. [Recall that this is an abbreviation for max(a[k]) < min(b[k]) and max(c[k]) < min(d[k]).]Suppose a < b and c < d. Then there is a k1 such that max(a[k1]) < min(b[k1]) and a k2 such that max(c[k2]) < min(d[k2]). Let k be the larger of k1 and k2. Then max(a[k]) ≤ max(a[k1]) < min(b[k1]) ≤ min(b[k]) and max(c[k]) ≤ max(c[k2]) < min(d[k2]) ≤ min(d[k]).
For example, this can be used to shorten the proof that < is transitive:
¥ If x < y and y < z, then x < z.Suppose x < y and y < z. Then there is a k such that max(x[k]) < min(y[k]) and max(y[k]) < min(z[k]). Then max(x[k]) < min(y[k]) ≤ max(y[k]) < min(z[k]). Thus x < z.
>>8925Although they follow obviously from the compatibility of < with =, for technical convenience I added some theorems about transitivity of < and =:
¥ If x < y and y = z, then x < z.¥ If x = y and y < z, then x < z.These are trivial consequences of the fact that x = y implies x ≤ y and the already proven theorems about transitivity of < and ≤.
>>8975Another property I forgot: The additive inverse of 0 is 0.
¥ -0 = 0Proof is by the usual automatic method.
It's also convenient to know
¥ If x = -y, then y = -x.Suppose x = -y. Then y = -(-y) = -x.
>>8984Another useful fact: A number is positive if and only if its additive inverse is negative. A number is negative if and only if its additive inverse is positive.
¥ x > 0 if and only if -x < 0.Suppose x > 0. Then -x < -0 = 0. Suppose -x < 0. Then -x < -0, and x > 0.
¥ x < 0 if and only if -x > 0.Suppose x < 0. Then -x > -0 = 0. Suppose -x > 0. Then -x > -0, and x < 0.
A number is greater than another if and only if their difference is positive:
¥ x < y if and only if y - x > 0This follows from adding (-x) to both sides of the inequality.
>>8992Another useful equation about multiplication:
¥ (-x) * (-y) = x * y.Proof is by the usual automatic method.
No.9461
Now let's start to cover the relationship between multiplication and inequalities.
We start with the fact that multiplying two positive numbers results in a positive number.
¥ x > 0 and y > 0 imply x * y > 0
Suppose x > 0 and y > 0. Then there is a k such that x[k] > [0,0]Q and y[k] > [0,0]Q. The lower bound min((x*y)[k]) can be written as r*s, where r ∈ x[k] and s ∈ y[k]. Since r ≥ min(x[k]) > 0 and s ≥ min(y[k]) > 0, we have min((x*y)[k]) > 0. Therefore x*y > 0.
Multiplying a postitive times a negative results in a negative.
¥ x > 0 and y < 0 imply x * y < 0
Suppose x > 0 and y < 0. Then -y > 0. Thus -(x*y) = x*(-y) > 0. It follows that x*y < 0.
Multiplying two negatives makes a positive.
¥ x < 0 and y < 0 imply x * y > 0
Suppose x < 0 and y < 0. Then -x > 0 and -y > 0. Therefore x*y = (-x)*(-y) > 0.
We can also go the opposite direction:
¥ If y > 0, then x * y > 0 implies x > 0.
Suppose y > 0 and x*y > 0. Then there is a k such that min(y[k]) > 0 and min((x*y)[k]) > 0. Because min(x[k]) ∈ x[k] and min(y[k]) ∈ y[k], we have min(x[k]) * min(y[k]) ∈ (x*y)[k]. Thus min(x[k]) * min(y[k]) ≥ min((x*y)[k]) > 0. Combined with min(y[k]) > 0, this implies min(x[k]) > 0. Therefore x > 0.
From these we can establish
¥ If z > 0, then x < y if and only if x * z < y * z.
1. Suppose z > 0 and x < y. Then y - x > 0, and y*z - x*z = (y-x)*z > 0. Therefore x*z < y*z.
2. Suppose z > 0 and x*z < y*z. Then (y-x)*z = y*z - x*z > 0, and y - x > 0. Therefore x < y.
A quick lemma, provable automatically:
¥ (x - y) * (-z) = y * z - x * z
If we multiply by a negative number, the inequalities are reversed:
¥ If z < 0, then x < y if and only if x * z > y * z.
Suppose z < 0. Note that -z > 0.
1. Suppose x < y. Then y - x > 0, and x*z - y*z = (y-x)*(-z) > 0. Therefore x*z > y*z.
2. Suppose x*z < y*z. Then (x-y)*(-z) = y*z - x*z > 0, and x - y > 0. Therefore x < y.
Because x ≤ y is defined as x > y being false, we have:
¥ If z > 0, then x ≤ y if and only if x * z ≤ y * z.
¥ If z < 0, then x ≤ y if and only if x * z ≥ y * z.
We can also prove
¥ If z <> 0, then x <> y if and only if x * z <> y * z.
Suppose z <> 0. Then z < 0 or z > 0.
If z < 0, then x <> y, defined as x < y or x > y, is equivalent to x*z > y*z or x*z < y*z, which is the same as x*z <> y*z.
If z > 0, then x <> y, defined as x < y or x > y, is equivalent to x*z < y*z or x*z > y*z, which is the same as x*z <> y*z.
Because x = y is defined as x <> y being false, we immediately have
¥ If z <> 0, then x = y if and only if x * z = y * z.
Because multiplication is commutative, everything we have proven about multiplication on the right also works on the left:
¥ x < 0 and y > 0 imply x * y < 0
¥ If x > 0, then x * y > 0 implies y > 0.
¥ If z > 0, then x < y if and only if z * x < z * y.
¥ If z < 0, then x < y if and only if z * x > z * y.
¥ If z > 0, then x ≤ y if and only if z * x ≤ z * y.
¥ If z < 0, then x ≤ y if and only if z * x ≥ z * y.
¥ If z <> 0, then x <> y if and only if z * x <> z * y.
¥ If z <> 0, then x = y if and only if z * x = z * y.
No.9462
What do you think would be a good way to represent signed digits on Kissu? The idea is that (using base ten as an example) instead of using the digits 0 through 9, you use the digits -9 through 9. For example, if a number has 6 in the tens place and -9 in the ones place, that's 6*10 + -9*1 = 51.
So I'm looking for a way to write the negative digits.
With a line over them is one option. You can do this with Unicode tricks, but support varies.
Do you see a line over the 3 or the 4 below? It's supposed to be over the 3, but some fonts might not render it correctly.
3̅4
In
>>9444 I used code tags to make things monospaced and an underscore on the line above. This works, but has limitations, including the need to add a mostly blank line above the numbers.
_
34Strikethrough with Unicode is another option, but again, support varies.
3̵4
There's also strikethrough with markup. And various other formatting options.
34
But they won't work (and shouldn't) inside code tags. The monospace font of code tags might be needed to line up numbers in a calculation.
[str]3[/str]4There are a lot of altered versions of numbers in Unicode, and some of them seem to be better supported than the overline or strikethrough, but they seem to often mess up alignment, even inside code blocks.
3⑨4⑨4⑨4
3949494I could try using letters, but then you have to do extra mental work to convert it to the number in you head, and that's annoying.
Any options I haven't thought of?
No.9463
>>9462>What do you think would be a good way to represent signed digits on Kissu?Uhh... create new numbers from scratch?
Yes, I think that's the ideal solution. We will have to name and draw them, but I think math has needed this for a while.
No.9464
>>9463That could be fun, although we'd need Vern to install a special font in order to be able to type them. And it would be best if they bear some connection to their positive counterparts so that we don't have to memorize a whole new plus and times table just to calculate with them.
No.9465
>>9464Oh. Yeah, I guess you'd actually need something like that, huh. Isn't there some weird unicode thing that makes the text afterwards show backwards? I remember people doing it on desuarchive
No.9467
>>9465There's a thing that makes the characters go from right to left instead of left to right, but it doesn't change the characters themselves.
There are also scripts for writing ʇxǝʇ uʍop-ǝpᴉsdn or with various other transformations. They work by finding characters that look similar to a flipped version of your original character. That might be useful, but it wouldn't work for the number 8.
Let's try it anyway (using
https://www.upsidedowntext.com/):123456789
ƖᄅƐㄣϛ9ㄥ86111111111133333333335555555555
ƖƖƖƖƖƖƖƖƖƖƐƐƐƐƐƐƐƐƐƐϛϛϛϛϛϛϛϛϛϛSome but not all of the characters break monospacing on my machine. And of course upside-down doesn't work well for 6, 8, and 9.
mirrored text using
https://lingojam.com/MirrorYourText123456789
ƖςƐμटმ٢8୧111111111122222222223333333333444444444466666666667777777777
ƖƖƖƖƖƖƖƖƖƖςςςςςςςςςςƐƐƐƐƐƐƐƐƐƐμμμμμμμμμμმმმმმმმმმმ٢٢٢٢٢٢٢٢٢٢Looks better. The choices for 5 and 9 still break monospacing for me a bit, and of course 8 still doesn't work. Still a lot of the numbers aren't very recognizable after transformation.
No.9468
>>9466Not really new numbers but new numerals.
Here's the problem. Suppose we want to add two real numbers starting with 0.333 and 0.666. If the numbers really go on like that forever, you could just add the digits pairwise to get 0.999... (needs some work to prove it's valid, but it is), which is the same as 1. But what if somewhere in the 0.666... number there's a 5 or a 7? If there's a 5 somewhere your answer has to be a little less than 1, meaning it has to start with 0.999, and if there's a 7 somewhere your answer has to be a little greater than 1, meaning it starts with 1.000. You don't know which to use until you find that digit. But since the numbers might add up to 1 exactly, you may never find that digit. That means you can never write down any digits of the answer.
If you add digits -9 through -1, this problem goes away. You can start with 1.000 in all three cases. If you need the answer to be slightly less than 1, you use a negative digit when needed. If you need the answer to be slightly bigger than 1, you can use a positive digit.
No.9469
>>9467Hmm, that stuff looks kind of confusing on second thought.
I guess you could highlight the stuff
No.9477
I changed some of the theorems in
>>8966 since it turns out to be useful to prove the implication both ways:
¥ Assuming Markov, x ≤ y is false if and only if x > y is true.¥ Assuming Markov, x ≥ y is false if and only if x < y is true.¥ Assuming Markov, "w < x or y < z" is not false if and only if "w < x or y < z" is true.¥ Assuming Markov, x = y if and only if x <> y is true.The proof in the reverse direction (P -> not not P) is trivial:
Suppose P. If we assume not P, we have a contradiction. Therefore not not P.
No.9480
>>9477Also useful to show
¥ Assuming the law of the excluded middle, not (not P) if and only if P.1. Suppose not (not P). Either P is true, in which case we are done, or P is false, contradicting not (not P).
2. Suppose P. If we assume not P, we have a contradiction. Therefore not not P.
No.9481
>>9468Interesting... I think I kind of get it.
No.9482
Now back to multiplication and inequalities. But first, let's prove a useful lemma about apartness.
¥ x <> Q2R(r) if and only if there exists a k such that r ∈ x[k] is false.1. Suppose x <> Q2R(r). Then there is a k such that max(x[k]) < r or a k such that min(x[k]) > r. Both contradict r ∈ x[k].
2. Suppose r ∈ x[k] is false. Either r < min(x[k]) or r ≥ min(x[k]). If r < min(x[k]), we have Q2R(r) < x, and we are done. If r ≥ min(x[k]), it cannot be the case that r ≤ max(x[k]). Therefore r > max(x[k]) and Q2R(r) > x.
¥ x * y <> 0 if and only if x <> 0 and y <> 01. Suppose x*y <> 0. Then there is a k such that 0 ∈ (x*y)[k] is false. It cannot be the case that 0 ∈ x[k] or 0 ∈ y[k], since either would make 0 = 0*0 ∈ (x*y)[k]. Therefore x <> 0 and y <> 0.
2. Suppose x <> 0 and y <> 0. Either x < 0 or x > 0; similarly, either y < 0 or y > 0. In all cases, x*y < 0 or x*y > 0. Therefore x*y <> 0.
Since equality is defined as the negation of apartness, we immediately have
¥ x * y = 0 if and only if it is not true that both x <> 0 and y <> 0If we allow the use of some axioms, we can restate this in more familiar ways.
First, a quick lemma.
¥ not (P or Q) is equivalent to (not P) and (not Q)1. Suppose it is not (P or Q). If we assume P, we can prove P or Q, which contradicts not (P or Q). Therefore not P. By a similar argument, we can conclude not Q. Therefore (not P) and (not Q).
2. Suppose not P and not Q. If we assume P or Q, we either have P, contradicting not P, or Q, contradicting not Q. Therefore not (P or Q).
¥ Assuming Markov's principle, x * y = 0 if and only if it is not false that x = 0 or y = 0.Assuming Markov's principle, x <> 0 is equivalent to the negation of x = 0, and y <> 0 is equivalent to the negation of y = 0. So x * y = 0 is equivalent to not ((not x = 0) and (not y = 0)), which is equivalent to not (not (x = 0 or y = 0)).
As before, by "false" I mean that we can deduce a contradiction from the statement. By "not false" I mean that if we assume that we can deduce a contradiction from the statement, then we can deduce a contradiction. In intuitionistic logic, a statement being proven "not false" does not in general prove the statement true. We can prove a "not false" statement true by invoking the axiom of excluded middle (see
>>9480), but that would make our proof non-constructive. As mentioned in
>>8959, "It is not false that P or Q" can be thought of as a way of expressing in the setting of intuitionistic logic what we would call just "P or Q" in classical logic. If we've proven this, we know that either P or Q is true, but we don't necessarily have a constructive proof that would tell us which one is true.
Is there a way to show that no constructive proof exists? I was thinking about this in
>>9015. I suspect that there's no way to prove it constructively, but I haven't proven that yet.
¥ Assuming the law of the excluded middle, x * y = 0 if and only if x = 0 or y = 0.LEM implies Markov, and makes "it is not false that" equivalent to the statement being true.
No.9486
A few more quick theorems before we move on to division.
¥ x * y > 0 if and only if (x > 0 and y > 0) or (x < 0 and y < 0)
1. Suppose x*y > 0. Then x*y <> 0. We have x <> 0, meaning x < 0 or x > 0, and y <> 0, meaning y < 0 or y > 0. We can eliminate the x < 0, y > 0 and x > 0, y < 0 cases because they imply x*y < 0. Therefore x > 0 ans y > 0 or x < 0 or y < 0.
2. Suppose either x > 0 and y > 0 or x < 0 and y < 0. In either case we have x*y > 0.
Similarly
¥ x * y < 0 if and only if either x > 0 and y < 0 or x < 0 and y > 0.
¥ If x ≥ 0 and y ≥ 0, then x * y ≥ 0.
Suppose x ≥ 0 and y ≥ 0. Assume to the contrary that x*y < 0. Either x > 0 and y < 0, contradicting y ≥ 0, or x < 0 and y > 0, contradicting x ≥ 0. Therefore x*y ≥ 0.
¥ If y ≥ 0, then x * y > 0 implies x > 0.
Suppose y ≥ 0 and x * y > 0. Either x > 0 and y > 0, in which case we are done, or x < 0 and y < 0, contradicting y ≥ 0.
¥ If z ≥ 0, then x * z < y * z implies x < y.
Suppose z ≥ 0 and x * z < y * z. Then (y - x) * z = y * z - x * z > 0, and y - x > 0 by the previous theorem. Thus x < y.
Because multiplication is commutative, we also have
¥ If x ≥ 0, then x * y > 0 implies y > 0.
¥ If z ≥ 0, then z * x < z * y implies x < y.
No.9490
>>8984Some more theorems about addition:
¥ The sum of two positive numbers (x, y > 0) is a positive number.¥ The sum of two negative numbers (x, y < 0) is a positive number.¥ The sum of two numbers which are ≥ 0 is ≥ 0.¥ The sum of two numbers which are ≤ 0 is ≤ 0.These are immediate consequences of
¥ If w < x and y < z, then w + y < x + z.¥ If w ≤ x and y ≤ z, then w + y ≤ x + z.and the fact that 0 + 0 = 0.
No.9491
>>8967Another addition: some obvious consequences of
> r < s if and only if Q2R(r) < Q2R(s).> r <> s if and only if Q2R(r) <> Q2R(s).are
¥ 0 < 1¥ 0 <> 1
No.9492
Checking our progress by comparing what we've proven so far with the axioms of the reals according to baby Rudin.
Order axioms> (i) If x ∈ S and y ∈ S then one and only one of the statements x < y, x = y, y < x is true.The "one" part is proven here
>>8947 (assuming LEM / using double-negated or).
The "only one" part is proven in
>>8922 (note that by our definitions proving that x = y implies x ≤ y and x ≥ y is the same as proving it implies the falsehood of y < x and x < y)
> (ii) If x, y, z ∈ S, if x < y and y < z, then x < z.Proven in
>>8920Field axioms> (A1) If x ∈ F and y ∈ F, their sum x + y is in F.We defined the function in
>>8972 and all functions in Coq are total.
> (A2) Addition is commutative: x + y = y + x.> (A3) Addition is associative: (x + y) + z = x + (y + z) for all x, y, z ∈ F.> (A4) F contains an element 0 such that 0 + x = x for every x ∈ F.Proven in
>>8973> (A5) To every x ∈ F corresponds an element -x ∈ F such that x + (-x) = 0.Proven in
>>8975> (M1) If x ∈ F and y ∈ F, then their product xy is in F.We defined the function in
>>8991 and all functions in Coq are total.
> (M2) Multiplication is commutative: xy = yx for all x, y ∈ F.> (M3) Multiplication is associative: (xy)z = x(yz) for all x, y, z ∈ F.Proven in
>>8992> (M4) F contains an element 1 ≠ 0 such that 1x = x for every x ∈ F.That 1 ≠ 0 is proven in
>>9491 (note <> is symmetric per
>>8922, and if we want to be picky, we can get the negation of 1 = 0 by noting that it is the double negation of 1 <> 0, and statements in intuitionistic logic imply their double negation).
That 1x = x is proven in
>>8992.
> (M5) If x ∈ F and x ≠ 0 then there exists an element 1/x ∈ F such that x · (1/x) = 1.Still needs proving.> (D) The distributive law x (y + z) = xy + xz holds for all x, y, z ∈ F.Proven in
>>8992Ordered field axioms> (i) x + y < x + z if x, y, z ∈ F and y < z,Proven in
>>8984> (ii) xy > 0 if x ∈ F, y ∈ F, x > 0, and y > 0.Proven in
>>9461Least-upper-bound property> Suppose S is an ordered set, E ⊂ S, and E is bounded above. Suppose there exists an α ∈ S with the following properties:> (i) α is an upper bound of E.> (ii) If γ < α then γ is not an upper bound of E.> Then α is called the least upper bound of E [that there is at most one such α is clear from (ii)] or the supremum of E, and we write α = sup E.> An ordered set S is said to have the least-upper-bound property if the following is true:> If E ⊂ S, E is not empty, and E is bounded above, then sup E exists in S.Still needs proving.
No.9504
Division is multiplication by the reciprocal of a number, also called the additive inverse. So we should first define the reciprocal.
The approach we have taken with all the other operations so far is to take the bounds on the inputs at a particular step number and ask, if we know the inputs are within this interval, what are the smallest and largest possible values for the output? That gives us the interval for the output at the same step number.
Let's try the same thing with the reciprocal now. If we know our value is between r and s inclusive (r ≤ x ≤ s), what can we say about the value of its reciprocal?
If r and s are both positive, then we have bounds 1/s ≤ x ≤ 1/r.
If r and s are both negative, then we have bounds 1/s ≤ x ≤ 1/r again.
But what about when r is negative and s is positive? Then there's no lower or upper limit on what 1/x can be.
Division by zero is undefined, so if a number is zero, we shouldn't be trying to take its reciprocal in the first place. But some of the intervals for a nonzero number could contain zero. On the other hand, the intervals for a nonzero number can't contain zero forever. Eventually there has to be a lower bound greater than zero or an upper bound less than zero.
We can fix this issue by searching for that first interval which does not contain zero and using it as a replacement for all the prior intervals which do contain zero. After this preprocessing, we can use the usual approach.
While writing the comparison function, we've already written a function
>>8963 to perform this sort of search. It takes real numbers w, x, y, z and a proof of w < x or y < z, and finds the first k such that max(w[k]) < min(x[k]) or max(y[k]) < min(z[k]). By feeding it the numbers x, 0, 0, x and a proof of x <> 0 (defined as x < 0 or 0 < x), it is guaranteed to find a k such that max(x[k]) < 0 or 0 < min(x[k]), or in other words, 0 ∉ x[k]. Let's call this k value find_zeroless(x, p) where x is the number and p is the proof that x <> 0.
We'll adopt the practice used in Coq of denoting the reciprocal of x as /x to distinguish it from the division 1/x, which is to be defined in terms of /x as 1/x = 1 · /x. For division of rational numbers, Coq handles division by zero by simply assigning a bogus value of zero to the result. This won't work for real numbers because we need to explicitly use the proof that x <> 0, and there's no way to decide whether x <> 0 or x = 0 by an algorithm given x. So in order to compute the reciprocal of x, we'll need to pass both x and a proof p that x <> 0. Let's denote this as /x †p. Sometimes when writing about /x and find_zeroless, where there is no ambiguity, we will omit the proof argument for brevity, but it will still be there, at least until program extraction.
We define
¥ /[a, b]Q = [/b, /a]Q¥ (/x †p)[k] = /(x[max{k, find_zeroless(x, p)}])Our reciprocal operation on rational intervals behaves as we want provided 0 is not a member:
¥ r ∈ [a, b]Q implies /r ∈ /[a, b]Q provided 0 ∉ [a, b]Q.Either 0 < a ≤ r ≤ b or a ≤ r ≤ b < 0. In either case /b ≤ /r ≤ /a.
And in the cases we use it, 0 will indeed not be a member:
¥ If k ≥ find_zeroless(x), then 0 ∉ x[k].We already have 0 ∉ x[find_zeroless(x)]. Since x[k] ⊆ x[find_zeroless(x)], this implies 0 ∉ x[k].
In particular it follows that
¥ 0 ∉ x[max{k, find_zeroless(x)}].Now let's prove that /x meets the conditions to be a member of our real number type.
First, nestedness:
>That each interval contains the endpoints of itself and all subsequent intervals. That is, if k2 ≥ k1, then min(x[k2]) ∈ x[k1] and max(x[k2]) ∈ x[k1].Suppose k2 ≥ k1. Let k1' = max{k1, find_zeroless(x)} and k2' = max{k2, find_zeroless(x)}. We also have k2' ≥ k1', and thus min(x[k2']) ∈ x[k1'] and max(x[k2']) ∈ x[k1']. Since 0 ∉ x[k1'], it follows that
min((/x)[k2]) = /max(x[k2']) ∈ /(x[k1']) = (/x)[k1]
max((/x)[k2]) = /min(x[k2']) ∈ /(x[k1']) = (/x)[k1].
Some quick lemmas before proving the next part:
¥ If a ≤ b and 0 ∉ [a, b]Q, then min{a², b²} > 0.Either 0 < a ≤ b or a ≤ b < 0. In either case a² > 0 and b² > 0. Therefore min{a², b²} > 0.
¥ If r, s ∈ [a, b]Q and 0 ∉ [a, b]Q, then r·s ≥ min{a², b²}.Either 0 < a ≤ r, s, in which case r·s ≥ a·s ≥ a² ≥ min(a², b²}, or r, s ≤ b < 0, in which case r·s ≥ b·s ≥ b² ≥ min{a², b²}.
Now we can prove convergence:
>That for any positive rational number, there will eventually be an interval in the sequence whose width (maximum - minimum) is smaller than that number.Suppose eps > 0. Let n = find_zeroless(x) and c = min{min(x[n])², max(x[n])²}. Since min(x[n]) ≤ max(x[n]) and 0 ∉ x[n], we have c > 0. Thus eps·c > 0, and there exists a k such that width(x[k]) < eps·c. Let k' = max{k, n} and [a, b]Q = x[k']. Since a, b ∈ x[n] and 0 ∉ x[n], we have a·b ≥ c > 0 and a, b ≠ 0. Therefore
width(/(x[k'])) = 1/a - 1/b = (b - a) / (a·b) = width(x[k']) / (a·b) ≤ width(x[k]) / c < eps.
Thus /x as we have defined it is a real number.
No.9505
Proving equations involving division will require a modified version of the lemma in
>>8983.
¥ If for every k ≥ n there exist rational numbers r ∈ x[k] and s ∈ y[k] such that r = s, then x = y.Suppose such an n exists. Assume to the contrary that x <> y. Then either there is a k such that x[k] < y[k] or a k such that x[k] > y[k]. In either case, let k' = max{k, n}. Since k' ≥ n, there are rational numbers r and s such that r ∈ x[k'], s ∈ y[k'], and r = s. Since k' ≥ k, r ∈ x[k] and s ∈ y[k]. We have either r ≤ max(x[k]) < min(y[k]) ≤ s or s ≤ max(y[k]) < min(x[k]) ≤ r. Both contradict r = s.
We'll also need this:
¥ If x <> 0 as proven by p, k ≥ find_zeroless(x, p), and r ∈ x[max{k, find_zeroless(x, p)}], then /r ∈ (/x †p)[k].Since 0 ∉ x[max{k, find_zeroless(x, p)}], r ∈ x[max{k, find_zeroless(x, p)}] implies /r ∈ /(x[max{k, find_zeroless(x, p)}]) = (/x †p)[k].
We can now show our definition of /x is compatible with the equality relation we have defined for real numbers.
¥ If x = y and p and q are proofs of x <> 0 and y <> 0 respectively, then /x †p = /y †q.Let n = max{find_zeroless(x, p), find_zeroless(y, q)}, and suppose we are given a k ≥ n. This means k ≥ find_zeroless(x, p) and k ≥ find_zeroless(y, p). Since x = y, there exists an r such that r ∈ x[k] = x[max{k, find_zeroless(x, p)] and r ∈ y[k] = y[max{k, find_zeroless(y, q)]. Therefore /r ∈ (/x †p)[k] and /r ∈ /(y †q)[k]. By the preceding lemma, we conclude /x †p = /y †q.
The following lemma will be helpful in automatic proofs of equations involving division:
¥ If k ≥ find_zeroless(x), then min x[k] <> 0.This follows from the similar lemma showing 0 ∉ x[k] and the fact that min x[k] ∈ x[k].
All of these equalities can be proven automatic fashion.
¥ /(/x) = x¥ Q2R(/r) = /(Q2R(r))¥ /1 = 1¥ /(-x) = -(/x)¥ x · /x = 1¥ /x · x = 1¥ /(x · y) = (/x) · (/y)In all of the above equations, any number whose reciprocal is taken must be apart from zero.
Some of the automatic proofs are a bit different before, so let's demonstrate with the all-important
¥ x · /x = 1.Let n = find_zeroless(x), and suppose we are given a k ≥ n. For the left hand side, we have min(x[max{k, n}]) ∈ x[max{k, n}], so /min(x[max{k, n}]) ∈ (/x)[k]. Combine this with min(x[k]) ∈ x[k], and we have min(x[k]) · /min(x[max{k, n}]) ∈ (x · /x)[k]. For the right hand side, we simply have 1Q ∈ 1[k]. It remains to show that these rational numbers are equal. Since x <> 0 and k ≥ find_zeroless(x), we have min(x[k]) <> 0. We can now assert the corresponding rational number theorem, min(x[k]) · / min(x[k]) = 1Q. Since k ≥ n, we can replace k with max{k, n} to get min(x[k]) · /min(x[max{k, n}]) = 1Q. Therefore x · /x = 1.
>>9492> (M5) If x ∈ F and x ≠ 0 then there exists an element 1/x ∈ F such that x · (1/x) = 1.Now proven.
No.9511
More theorems about reciprocals. But first:
>>8984>>9460> x > 0 if and only if -x < 0.> x < 0 if and only if -x > 0.These can be usefully combined into
¥ x <> 0 if and only if -x <> 0.Back to reciprocals.
¥ x > 0 if and only if /x > 0We have x · /x = 1 > 0. Thus if x > 0, /x > 0, and if /x > 0, x > 0.
¥ x < 0 if and only if /x < 0x < 0 ↔ -x > 0 ↔ /(-x) > 0 ↔ -(/x) > 0 ↔ /x < 0
¥ If x <> 0, then /x <> 0.Follows from the definition of <> as < or >.
¥ If x > 0 and y > 0, then x < y if and only if /y < /x.¥ If x < 0 and y < 0, then x < y if and only if /y < /x.We can prove both of these the same way. If either x > 0 and y > 0 or x < 0 and y < 0, we have xy > 0 and /(xy) = (/x)(/y) > 0.
Then x < y ↔ x · y · /y < y · x · /x ↔ /y < /x.
¥ If x > 0 and y > 0, then x ≤ y if and only if /y ≤ /x.¥ If x < 0 and y < 0, then x ≤ y if and only if /y ≤ /x.These follow from the previous because ≤ is defined as not >.
¥ If x, y <> 0, then x <> y if and only if /x <> /y.If x, y <> 0, then xy <> 0. Then x <> y ↔ x · y · /y <> y · x · /x ↔ /y <> /x ↔ /x <> /y.
¥ If x, y <> 0, then x = y if and only if /x = /y.Follows from the previous because = is defined as not <>.
¥ If x, y <> 0, then /x < /y if and only if 0 < y < x, y < x < 0, or x < 0 < y.1. Suppose /x < /y. Either x > 0 or x < 0, and either y > 0 or y < 0.
1a. If x > 0 and y > 0, we have 0 < y < x.
1b. If x > 0 and y < 0, we have /y < 0 < /x, contradicting /x < /y.
1c. If x < 0 and y > 0, we have x < 0 < y.
1d. If x < 0 and y < 0, we have y < x < 0.
2. Suppose 0 < y < x, y < x < 0, or x < 0 < y.
2a. If 0 < y < x, then /x < /y.
2b. If y < x < 0, then /x < /y.
2c. If x < 0 < y, then /x < 0 < /y.
No.9512
What would be a good way to make these numbers easily available for people here to play around with them? Things like doing addition / subtraction / multiplication / division and eventually other more complicated operations to arbitrarily many decimal points. (I'll need to do some work on converting these things to decimals first.)
I was thinking about using the program extraction feature to export the programs to some other language, then make a little website with a REPL on it. (For those unfamiliar, REPL means read-eval-print-loop; you type the thing you want to evaluate in some programming language and it prints the result.)
I could also do something similar without exporting from Coq by using this thing:
https://coq.vercel.app/I'd probably have to include some examples on the page.
Another option is to use the program extraction feature to make the objects available in [insert your favorite language here], so that interested people could download something and play with it on their machine.
Are there any particular programming languages lots of people here are familiar that would be worth targeting?
No.9513
Division is defined as multiplying by the reciprocal:
¥ x/y †p = x·(/y †p)
(The †p indicates a proof of y <> 0).
Since multiplication and the reciprocal are compatible with equality, so is division:
¥ If x1 = x2 and y1 = y2, and p1 and p2 prove y1 <> 0 and y2 <> 0 respectively, then x1 / y1 †p1 = x2 / y2 †p2.
Suppose x1 = x2 and y1 = y2. Then /y1 †p1 = /y2 †p2. Therefore x1 / y1 †p1 = x1 · (/y1 †p1) = x2 · (/y2 †p2) = x2 / y2 †p2.
A quick lemma to help with automated proofs:
¥ If r ∈ x[k] and s ∈ y[max{k, find_zeroless(y, p)}], then r/s ∈ (x/y †p)[k].
If s ∈ y[max{k, find_zeroless(y, p)}], we have /s ∈ (x/y †p)[k]. Given this and r ∈ x[k], we have r/s = r·(/s) ∈ (x·(/y †p))[k] = (x/y †p)[k].
Division is the inverse of multiplication. A few ways of stating this:
¥ If y <> 0, (x · y) / y = x.
¥ If y <> 0, (x / y) · y = x.
These can be proven automatically.
Another way to say this:
¥ If y <> 0, x / y = z if and only if x = z · y.
1. Suppose x / y = z. Then x = (x / y) · y = z · y.
2. Suppose x = z · y. Then x / y = (z · y) / y = z.
You can divide both sides of an inequality by a positive number. Dividing both sides by a negative number reverses the direction of the inequality.
¥ If z > 0, then x < y if and only if x / z < y / z.
¥ If z > 0, then x ≤ y if and only if x / z ≤ y / z.
¥ If z < 0, then x < y if and only if x / z > y / z.
¥ If z < 0, then x ≤ y if and only if x / z ≥ y / z.
These follow trivially from the related theorems about multiplication, and the fact that the reciprocal of a positive number is positive, and the reciprocal of a negative number is negative.
Next is completeness.
No.9515
>>9512>What would be a good way to make these numbers easily available for people here to play around with them?I really don't know anything about programming, but an interactive website like you mentioned is probably the best way. This stuff is way over my head and I'm a visual/interactive learning person.
Math... we must destroy it.
No.9519
>>9514No rush, it will probably be a bit before I work on this. I'm going to start proving stuff about completeness next, and I'll probably write the programs to convert to and from decimal representations as part of that. I think these theorems are going to be more interesting than proving elementary properties of the basic arithmetic operations.
No.9526
There are several versions of the completeness axiom for the real numbers. Probably the most commonly used one is the supremum property, also known as the least-upper-bound property or Dedekind completeness. I talked about this axiom in
>>9492, and it's the only one on the list I haven't proven yet.
There's a fun paper called "Real Analysis in Reverse" which examines lots of different properties of the real numbers, and talks about which ones, taken together with the ordered field axioms (every axiom in
>>9492 except the last one, or in other words, most of what you learned in high school algebra), imply the supremum property, and which ones don't.
https://arxiv.org/abs/1204.4483The author suggests treating the paper as a series of puzzles:
>It’s been fun for me to write this article, and I have a concrete suggestion for how the reader may have the same kind of fun. Start by reading the second section and trying to decide on your own which of the properties are equivalent to completeness and which aren’t. If you’re stumped in some cases, and suspect but cannot prove that some particular properties aren’t equivalent to completeness, read the third section to see if any of the ordered fields discussed there provide a way to see the inequivalence. And if you’re still stumped, read the fourth section. You can treat the article as a collection of math puzzles, some (but not all) of which can be profitably contemplated in your head.You may want to try them out for yourself. Here's the list of properties from the paper (you may want to read them in the paper itself, where the typesetting will be better):
>(1) The Dedekind Completeness Property: Suppose S is a nonempty subset of R that is bounded above. Then there exists a number c that is an upper bound of S such that every upper bound of S is greater than or equal to c.>(2) The Archimedean Property: For every x ∈ R there exists n ∈ N with n > x. Equivalently, for every x ∈ R with x > 0 there exists n ∈ N with 1/n < x.>(3) The Cut Property: Suppose A and B are nonempty disjoint subsets of R whose union is all of R, such that every element of A is less than every element of B. Then there exists a cutpoint c ∈ R such that every x < c is in A and every x > c is in B . (Or, if you prefer: Every x ∈ A is ≤ c, and every x ∈ B is ≥ c. It’s easy to check that the two versions are equivalent.) Since this property may be unfamiliar, we remark that the Cut Property follows immediately from Dedekind completeness (take c to be the least upper bound of A).>(4) Topological Connectedness: Say S ⊆ R is open if for every x in S there exists ϵ > 0 so that every y with |y−x| < ϵ is also in S. Then there is no way to express R as a union of two disjoint nonempty open sets. That is, if R = A ∪ B with A, B nonempty and open, then A ∩ B is nonempty.>(5) The Intermediate Value Property: If f is a continuous function from [a, b] to R, with f(a) < 0 and f(b) > 0, then there exists c in (a, b) with f(c) = 0.>(6) The Bounded Value Property: If f is a continuous function from [a, b] to R, there exists B in R with f(x) ≤ B for all x in [a, b].>(7) The Extreme Value Property: If f is a continuous function from [a, b] to R, there exists c in [a, b] with f(x) ≤ f(c) for all x in [a, b].>(8) The Mean Value Property: Suppose f : [a, b] → R is continuous on [a, b] and differentiable on (a, b). Then there exists c in (a, b) such that f′(c) = (f(b)−f(a))/(b−a).>(9) The Constant Value Property: Suppose f : [a, b] → R is continuous on [a, b] and differentiable on (a, b), with f′(x) = 0 for all x in (a, b). Then f(x) is constant on [a, b].>(10) The Convergence of Bounded Monotone Sequences: Every monotone increasing (or decreasing) sequence in R that is bounded converges to some limit.>(11) The Convergence of Cauchy Sequences: Every Cauchy sequence in R is convergent.>(12) The Fixed Point Property for Closed Bounded Intervals: Suppose f is a continuous map from [a, b] ⊂ R to itself. Then there exists x in [a, b] such that f(x) = x.>(13) The Contraction Map Property: Suppose f is a map from R to itself such that for some constant c < 1, |f(x)−f(y)| ≤ c|x−y| for all x, y. Then there exists x in R such that f(x) = x.>(14) The Alternating Series Test: If a₁ ≥ a₂ ≥ a₃ ≥ ... and a_n → 0, then ∑_{n=1}^∞ (−1)ⁿ a_n converges.>(15) The Absolute Convergence Property: If ∑_{n=1}^∞ |a_n| converges in R, then ∑_{n=1}^∞ a_n converges in R.>(16) The Ratio Test: If | a_{n+1} / a_n | → L in R as n → ∞, with L < 1, then ∑_{n=1}^∞ a_n converges in R.>(17) The Shrinking Interval Property: Suppose I₁ ⊇ I₂ ⊇ ... are bounded closed intervals in R with lengths decreasing to 0. Then the intersection of the I_n’s is nonempty.>(18) The Nested Interval Property: Suppose I₁ ⊇ I₂ ⊇ ... are bounded closed intervals in R. Then the intersection of the I_n’s is nonempty.Some of these imply the supremum property, and some don't. And sometimes two of the properties taken individually don't imply the supremum property, but do imply it when taken together.
The particular properties I'm planning to talk about over the next few posts are (1), (2), (3), (11), and (17).
No.9532
>>9526Let's start by proving the Archimedean property.
But first we'll need to be able to convert between various number types. Let's follow the pattern of Q2R in denoting the conversion functions. These already exist in the Coq standard library (although not by the name we're giving them here):
¥ N2Z converts natural numbers to integers¥ Z2Q converts integers to rational numbers¥ Z2N converts integers to natural numbers (The result for negative numbers is unspecified. As implemented, it's 0, but we should avoid relying on that.)We can easily define the additional functions N2Q, N2R, and Z2R by combining these and our own Q2R.
With that done, we now prove the Archimedean property:
¥ For every real number x, there is a natural number n such that N2R(n) > x.This proof is pretty simple. Because of the way we represent real numbers, we already have a series of rational upper bounds for x. So we can just take the first upper bound and use the first natural number integer greater than it. To be specific, let
n = Z2N(max{Qfloor(max(x[0
])) + 1, 0})
where Qfloor is a function (from the standard libary) which for a given rational number returns the greatest integer less than or equal to it. Note that max{Qfloor(max(x[0])) + 1, 0} ≥ 0. Then we have
N2R(n) = Z2R(N2Z(Z2N(max{Qfloor
(max(x[0])) + 1, 0}))) = Z2R(max{Qfloor(max(x[0
])) + 1, 0}) ≥ Z2R(Qfloor(max(x[0])) + 1) > Q2R(max(x[0])) ≥ x.
This has the important corollary:
¥ For every real number x, if x > 0, there is a natural number n such that /N2R(n) < x.(Recall that we use /x to denote the reciprocal of x.)
Since x > 0, /x is defined and is also > 0. By the previous theorem, there is an n such that N2R(n) > /x. Since N2R(n) > /x > 0, we have /N2R(n) < //x = x.
No.9533
>>9526Next up:
>(17) The Shrinking Interval Property: Suppose I₁ ⊇ I₂ ⊇ ... are bounded closed intervals in R with lengths decreasing to 0. Then the intersection of the I_n’s is nonempty.This is reminiscent of how we constructed our real numbers in the first place. The main difference is that in our construction of the real numbers, the endpoints of the intervals had to be rational numbers, but in this theorem, the endpoints are themselves real numbers.
Since we're trying to be constructive whenever possible, rather than just proving the existence of a number in the intersection, we'd like to have an algorithm to calculate it. Calculating the number, as usual, means computing a sequence of nested intervals with rational endpoints that converge to the right result, based on the sequences of intervals that represent the input numbers. The efficiency of the algorithm matters. We're going to be using it later on to compute infinite sums. I've personally gone through a few designs for this algorithm in my head, and I'll post my current design once it's implemented and proven.
You might be able to come up with something better than what I've got. How would you do it?
No.9534
>>9533Design 3 turned out not to work. I think design 4 will work though.
No.9535
>>9533Our input is a sequence of pairs of real numbers, representing upper and lower bounds. And we're representing the real numbers themselves as sequences of pairs of rational numbers, representing upper and lower bounds. So we have a sequence of pairs of sequences of pairs of rational numbers. And our output needs to be a sequence of pairs of rational numbers.
design 1My first idea here was the simple idea of making a sequence where the kth output lower bound is the kth rational lower bound from the kth real lower bound, and the kth output upper bound is the kth rational upper bound from the kth real upper bound.
The problem is that while this gives us valid upper and lower bounds for the number, they don't necessarily converge. To see why, consider the sequence of real intervals
[0A, 0A], [0B, 0B], [0C, 0C], ...
where the 0A, 0B, 0C, ... are all different representations of the real number 0, with
0A = [-1/1, 1/1]Q, [-1/2, 1/2]Q, [-1/3, 1/3]Q, [-1/4, 1/4]Q, ...
0B = [-1/1, 1/1]Q, [-1/1, 1/1]Q, [-1/2, 1/2]Q, [-1/3, 1/3]Q, ...
0C = [-1/1, 1/1]Q, [-1/1, 1/1]Q, [-1/1, 1/1]Q, [-1/2, 1/2]Q, ...
Even though the interval sequences defining the real numbers converge to zero, and the sequence of real numbers converges to zero, if we merge them together with the simple recipe, we get the sequence
[-1/1, 1/1]Q, [-1/1, 1/1]Q, [-1/1, 1/1]Q, ...
which doesn't converge at all! So we'll have to do something a bit smarter.
No.9540
>>9533>>9535design 2An obvious modification to design 1 which ought to make it converge: Instead of just using the kth rational lower bound from the kth real lower bound, look at all the kth rational lower bounds from real lower bounds 0 through k and take the best (the largest). Similarly look at all the kth rational upper bounds from real upper bounds 0 through k and take the best (the smallest).
For the example from
>>9535 that broke convergence for design 1, we get
¥ [-1/1, 1/1]Q, [-1/2, 1/2]Q, [-1/3, 1/3]Q, ...converging to zero as desired.
It's a simple solution, but it feels like it might require us to do some needless work. Let's look at how it would perform in a concrete example. We'll try using nested real intervals to calculate tan⁻¹(√(1/2)).
One way to calculate √(1/2) is the simple method in
>>8908. We can work out one decimal place at a time by squaring the candidate number and seeing if it comes out lower than 1/2. We get the following sequence:
¥ [0.7, 0.8]Q, [0.70, 0.71]Q, [0.707, 0.708]Q, [0.7071, 0.7072]Q, [0.70710, 0.70711]Q, ...The arctangent function has the following power series, which converges when |x| ≤ 1:
¥ tan⁻¹(x) = x - x³/3 + x⁵/5 - x⁷/7 + x⁹/9 - x¹¹/11 + ...A nice thing about this series is that it's an alternating series, meaning the terms we're adding are alternately positive and negative. This combined with the fact that the absolute value of the terms is decreasing means that each partial sum is a new bound on the number. So we immediately get the following nested interval representation for the case 0 ≤ x ≤ 1 (when -1 ≤ x ≤ 0, the intervals need to be in the other direction):
¥ tan⁻¹(x) = the number in the intersection of¥ [x - x³/3, x],¥ [x - x³/3, x - x³/3 + x⁵/5],¥ [x - x³/3 + x⁵/5 - x⁷/7, x - x³/3 + x⁵/5],¥ [x - x³/3 + x⁵/5 - x⁷/7, x - x³/3 + x⁵/5 - x⁷/7 + x⁹/9],¥ [x - x³/3 + x⁵/5 - x⁷/7 + x⁹/9 - x¹¹/11, x - x³/3 + x⁵/5 - x⁷/7 + x⁹/9],¥ ...Note that to handle the case of irrational x, these need to be intervals with real number endpoints. So we'll have to use the algorithm we're writing to find it.
Let's start by working out the real numbers involved. With our existing algorithms for arithmetic operations we would get
¥ x = [0.7, 0.8]Q, [0.70, 0.71]Q, [0.707, 0.708]Q, [0.7071, 0.7072]Q, [0.70710, 0.70711]Q, ...¥ x - x³/3 = [0.7 - 0.8³/3, 0.8 - 0.7³/3]Q, [0.70 - 0.71³/3, 0.71 - 0.70³/3]Q, [0.707 - 0.708³/3, 0.708 - 0.707³/3]Q, ...¥ x - x³/3 + x⁵/5 = [0.7 - 0.8³/3 + 0.7⁵/5, 0.8 - 0.7³/3 + 0.8⁵/5]Q, [0.70 - 0.71³/3 + 0.70⁵/5, 0.71 - 0.70³/3 + 0.71⁵/5]Q, [0.707 - 0.708³/3 + 0.707⁵/5, 0.708 - 0.707³/3 + 0.708⁵/5]Q, ...¥ x - x³/3 + x⁵/5 - x⁷/7 = [0.7 - 0.8³/3 + 0.7⁵/5 - 0.8⁷/7, 0.8 - 0.7³/3 + 0.8⁵/5 - 0.7⁷/7]Q, [0.70 - 0.71³/3 + 0.70⁵/5 - 0.71⁷/7, 0.71 - 0.70³/3 + 0.71⁵/5 - 0.70⁷/7]Q, [0.707 - 0.708³/3 + 0.707⁵/5 - 0.708⁷/7, 0.708 - 0.707³/3 + 0.708⁵/5 - 0.707⁷/7]Q, ...Merging the bounds together with the design 2 algorithm, we get
¥ tan⁻¹(x) =¥ [max{0.7 - 0.8³/3}, min{0.8}]Q,¥ [max{0.70 - 0.71³/3, 0.70 - 0.71³/3}, min{0.71, 0.71 - 0.70³/3 + 0.71⁵/5}]Q,¥ [max{0.707 - 0.708³/3, 0.707 - 0.708³/3, 0.707 - 0.708³/3 + 0.707⁵/5 - 0.708⁷/7}, min{0.708, 0.708 - 0.707³/3 + 0.708⁵/5, 0.708 - 0.707³/3 + 0.708⁵/5}]Q,¥ ...It's honestly not as bad as I thought it would be. On the one hand, we're clearly doing a lot of unnecessary comparisons. It's obvious in this case that the best bound out of each set that we're taking the max/min of will be the last bound. But I thought computing the extraneous bounds would require a lot of extra work, when in fact they can be computed with work we would have to do anyway. It's enough to make me consider implementing this simple algorithm instead of design 4. But before I do that, I'll write out design 4 so I can see if it's really that much more complex when I write out all the details.
No.9545
design 3
Let m index the pairs of real number bounds, and let n index the pairs of rational number bounds that define each real number. At a particular m and n, you have four rational numbers. There are two real numbers, an upper bound and a lower bound, and at stage n each of those real numbers has a rational upper and lower bound.
The motivation: Notice that if we fix m and increase n, we get increasingly better bounds, but only up to a point. Unless the two real numbers are actually equal, eventually the lower bound on the upper bound becomes larger than the upper bound on the lower bound. This creates a limit on how close the upper bound on the upper bound and the lower bound on the lower bound can get to each other. If the next pair of real numbers (real number pair m+1) has upper bounds on the upper bound and lower bounds on the lower bound that are inside this gap, then the real number m is guaranteed to be no longer useful for computing better approximations to our target value. I've drawn a crude picture to try to explain what I mean.
The idea of design 3 was to increment n at each step, but only increment m if you encounter a situation like the one I just described. And at each such step, use the upper bound on the upper bound and the lower bound on the lower bound as rational bounds on the target value.
But I hadn't entirely thought this idea through, and after further thought I realized that the situation triggering an advance in m wasn't guaranteed to happen, even if real number pair m exhibited a gap preventing further progress. After some further thinking, I came to design 4, which I think will work.
design 4
This is pretty similar to the previous except with a different condition for triggering m to increment. This time, the trigger is when for real number pair m the gap between the lower bound on the upper bound and the upper bound on the lower bound is at least half the distance between the upper bound on the upper bound and lower bound on the lower bound. As before, we use the upper bound on the upper bound and the lower bound on the lower bound as bounds on the target value. A complication is that these bounds are not guaranteed to be nested, but that can be fixed by taking the minimum of all the upper bounds found so far, and the maximum of all the lower bounds found so far.
I think that since this version of the real numbers has been focused on simplicity, and the efficiency problems weren't as bad as I thought, I'm going to go with design 2 for now. I might look at design 4 again when I try for a more efficient version.
No.9561
>>9462I had another idea regarding signed digits. The digits could be written as something like
↓1, ↓2, ↓3, ↓4, ↓5, ↓6, ↓7, ↓8, ↓9, 0, 1, 2, 3, 4, 5, 6, 7, 8, 9
with the ↓ representing -10. So for example 2.65000↓37 would be equal to 2.6499937.
You could also write it as a line through all the previous digits until you encounter a nonzero one, like 2.6
500037. Since there's no ↓0 digit, there's no ambiguity here; you can tell the strikethrough is coming from the ↓3. This wouldn't work if the negative digit was the first nonzero digit, but in that case, you could just use a negative sign in front of the whole number and adjust how you write digits accordingly.
The nice thing about this is that it makes it easy to convert the result into standard form.
No.9592
I'm thinking maybe I should start working on conversion to / from decimals earlier than I planned so I can have something for people to play around with as I talked about in
>>9512.
In other news, some small bits of progress as I work on the proof of the nested interval theorem:
Proved some more missed theorems that ought to have proven earlier.
>>8977>>9513Proofs are by the usual automatic method.
¥ Q2R(r - s) = Q2R(r) - Q2R(s)¥ If s <> 0 and Q2R(s) <> 0 by p, then Q2R (r / s) = Q2R(r) / Q2R(s) † p.>>8920A useful lemma about proving x < y:
¥ For any k1 and k2, if max(x[k1]) < min(y[k2]), then x < y.Let k be the larger of k1 and k2. Then max(x[k]) ≤ max(x[k1]) < min(y[k2]) ≤ min(y[k]), proving x < y.
Also some progress on proof automation. Coq has some nice tactics called ring and field that can be used to automate reasoning about basic algebra, like proving (a - b) + (b - c) = a - c. To use them, you first have to prove the ring / field axioms (already done, although I had to reverse the direction of some equations), then declare the structure a ring / field to Coq. I had to use the less powerful ring option (which can't do stuff with division) for the technical reason that Coq's field tactic expects division to take only two numbers as arguments, whereas we have to feed in an additional proof that the denominator is nonzero.
No.9594
>>6896>If the number happens to be exactly one, it might even cause our program to get into an infinite loop.But why though ?
Can you give a little explanation on what that is ?
No.9595
>>9594>Can you give a little explanation on what that is ?Can you give a little explanation on why that is ?
No.9596
>>9594Because the number may be exactly 1 but we don't know if it's exactly 1. In order to output that first "1" digit instead of a "0" we need to compute the number to sufficient precision to know that it's not less than 1. But if the number happens to be exactly 1, infinite precision is required. We can't output "0." either, because the number could be slightly larger than 1, and in order to know this isn't the case, we need infinite precision.
To give a more concrete example, think about what happens when we first compute √2 and then square it. Computing √2 means being able to compute ever-better approximations to √2. With more computation, we can know that the value is between 1.4 and 1.5, between 1.41 and between 1.42, between 1.414 and 1.415, and so on. More computation means better precision. What does that tell us about the square of this number? First we know it's between 1.96 and 2.25, then we know it's between 1.9881 and 2.0164, then we know it's between 1.999396 and 2.002225. But we never have it precisely enough to know if it starts with 1.9 or with 2.0.
There might be a more rigorous proof using what I've learned are called "fugitive sequences." I've mentioned these before in
>>8947 :
>Why do we expect not to be able to prove trichotomy constructively? Because that would mean that we have a way to compute for any given pair of real numbers whether or not they are equal. Confirming that two numbers are equal would in general require us to compute both numbers to infinite precision. To make the impossibility more clear, we could define a real number in decimal form as follows: It starts with 0.999 and the 9's go on until a given Turing machine halts. Is this number equal to 1? We can't know without solving the halting problem.That said, I don't know the proof. Maybe solving
>>9012 would help.
No.9597
Here's an interesting paper I found about various ways people have found to construct the real numbers:
https://arxiv.org/pdf/1506.03467.pdfThe implementation I'm working on now is essentially the same as Bachmann's construction.
>>6896>Cauchy sequences in their full generality feel a bit too complicated for my taste. It's possible the complexity at the beginning makes the implementation of stuff later on easier, though. But I'm going to try something else first.I should say that after trying various things I appreciate the Cauchy sequence construction (actually due to Cantor, it turns out) a lot more. In particular the fact that you can define arithmetic operations simply by doing the operations termwise on the the input sequences is really nice.
Making the modulus of convergence explicit, which is necessary to actually compute the number, also reduces the apparent complexity of the Cauchy sequence construction a bit. To explain this a bit more: If I just look at a finite number of terms in a Cauchy sequence, I have no idea what the number is. You need an infinite number of terms to know what limit the sequence converges to, and you can't compute that. What you have to do instead is go to the proof that the sequence is Cauchy. If I have some precision ε > 0 which which I want to know the number, the proof (assuming it's constructive) gives me an N beyond which the terms in the sequence have to be less than ε apart from each other, and it can be proven that at that point in the sequence, you are no more than ε away from the limit. A modulus of convergence is an explicit function that takes ε and gives you the appropriate N. Suppose we call this function f. Instead of saying
> for every ε > 0 there exists an N such that for m,n > N, |x_m - x_n| < εwe can just say
> for every ε > 0 and m,n > f(ε), |x_m - x_n| < εwhich, although it says the same thing, is potentially easier to wrap your head around because of the smaller number of nested quantifiers.
No.9598
>>9540Time for the formal proof that design 2 works.
First some new notation. We've been denoting the minimum and maximum of a closed interval [a, b] as min([a, b]) = a and max([a, b]) = b. Because we'll be working with sequences of intervals whose endpoints are also sequences of intervals, a notation that can be chained more cleanly will be helpful. So instead we'll use [a, b].min = a and [a, b].max = b.
We define the intersection of nested shrinking intervals of real numbers as
¥ nested_RI_int(I, p, q)[n] = [max{I[m].min[n].min | m ≤ n}, min{I[m].max[n].max | m ≤ n}]Qwhere I is a sequence of bounded closed intervals with two real numbers as endpoints,
where p is a proof that the intervals are nested, meaning
¥ k2 ≥ k1 implies I[k2].min, I[k2].max ∈ I[k1],and where q is a proof that the widths of the intervals converge to zero, meaning
¥ for every real number eps > 0 there is a k such that I[k].max - I[k].min < eps.(Strictly speaking the intervals converging to zero would be the stronger statement that
for every real number eps > 0 there is an n such that k ≥ n implies I[k].max - I[k].min < eps.
In other words, the intervals get sufficiently small and stay that way forever. This stronger statement can be deduced from p and q together since p prevents intervals from having widths larger than previous intervals. So we won't need it as a condition.)
max{f(m) | m ≤ n} is computed recursively:
¥ max{f(m) | m ≤ 0} = f(0)¥ max{f(m) | m ≤ n+1} = max{max{f(m) | m ≤ n}, f(n+1)}as is min{f(m) | m ≤ n}:
¥ min{f(m) | m ≤ 0} = f(0)¥ min{f(m) | m ≤ n+1} = min{min{f(m) | m ≤ n}, f(n+1)}To say that our function returns real numbers we need proofs of
¥ nestedness and¥ convergence.We also need to show
¥ compatibility with equality (changing one of the inputs to a different representation of the same real number shouldn't change the output) and¥ proof the number constructed lies within all the intervals.And we should also show
¥ uniqueness: there is only one real number in the intersection.But first we need some lemmas about the min/max of a finite set of rationals as defined recursively above.
¥ There is a k ≤ n such that min{f(m) | m ≤ n} = f(k).Proceed by induction. The base case f(0) = f(0) is trivial. Assume for some n that there is a k ≤ n such that min{f(m) | m ≤ n} = f(k). Then either min{f(m) | m ≤ n+1} = min{f(m) | m ≤ n} = f(k) or min{f(m) | m ≤ n+1} = f(n+1).
¥ If k ≤ n, then min{f(m) | m ≤ n} ≤ f(k).Again proceed by induction. In the base case, k ≤ 0 implies k = 0, so we only need to show f(0) ≤ f(0), which is trivial. Assume for some n that k ≤ n implies min{f(m) | m ≤ n} ≤ f(k). Given k ≤ n+1, we either have k ≤ n or k = n+1. If k ≤ n, then min{f(m) | m ≤ n+1} = min{min{f(m) | m ≤ n}, f(n+1)} ≤ min{f(m) | m ≤ n} ≤ f(k). If k = n+1, then min{f(m) | m ≤ n+1} = min{min{f(m) | m ≤ n}, f(n+1)} ≤ f(n+1) = f(k).
By similar proofs, we have:
¥ There is an m ≤ n such that max{f(n) | m ≤ n} = f(m).¥ If k ≤ n, then max{f(m) | m ≤ n} ≥ f(k).nestednessWe need to show
¥ whenever n2 ≥ n1,¥ max{I[m].min[n1].min | m ≤ n1} ≤ max{I[m].min[n2].min | m ≤ n2} ≤ min{I[m].max[n1].max | m ≤ n1} and¥ max{I[m].min[n1].min | m ≤ n1} ≤ min{I[m].max[n2].max | m ≤ n2} ≤ min{I[m].max[n1].max | m ≤ n1}.¥ max{I[m].min[n1].min | m ≤ n1} ≤ max{I[m].min[n2].min | m ≤ n2}There is a k ≤ n1 such that max{I[m].min[n1].min | m ≤ n1} = I[k].min[n1].min. Since n2 ≥ n1 and the intervals defining I[k].min are nested, we have I[k].min[n1].min ≤ I[k].min[n2].min. Since k ≤ n1 ≤ n2, I[k].min[n2].min ≤ max{I[m].min[n2].min | m ≤ n2}, completing the proof.
¥ min{I[m].max[n2].max | m ≤ n2} ≤ min{I[m].max[n1].max | m ≤ n1}Similar to the previous. There is a k ≤ n1 such that min{I[m].max[n1].max | m ≤ n1} = I[k].max[n1].max. Since n2 ≥ n1 and the intervals defining I[k].max are nested, we have I[k].max[n1].max ≥ I[k].max[n2].max. Since k ≤ n1 ≤ n2, I[k].max[n2].max ≥ min{I[m].max[n2].max | m ≤ n2}, completing the proof.
Now we need to do the parts
¥ max{I[m].min[n2].min | m ≤ n2} ≤ min{I[m].max[n1].max | m ≤ n1}¥ max{I[m].min[n1].min | m ≤ n1} ≤ min{I[m].max[n2].max | m ≤ n2}.From the fact that the intervals I are nested we can show
¥ I[m1].min ≤ I[m2].max for any m1, m2.Either m1 ≥ m2, in which case I[m1].min ∈ I[m2], or m2 ≥ m1, in which case I[m2].max ∈ I[m1].
To prove p ≤ q for rational p and q, it is sufficient to disprove p > q.
1. Suppose max{I[m].min[n2].min | m ≤ n2} > min{I[m].max[n1].max | m ≤ n1}. Then there are j, k such that I[j].min[n2].min > I[k].max[n1].max, showing I[j].min > I[k].max. This contradicts I[j].min ≤ I[k].max.
2. Suppose max{I[m].min[n1].min | m ≤ n1} > min{I[m].max[n2].max | m ≤ n2}. Then there are j, k such that I[j].min[n1].min > I[k].max[n2].max, showing I[j].min > I[k].max. Again, this contradicts I[j].min ≤ I[k].max.
No.9600
>>9598convergenceWe need to show
¥ for every rational number eps > 0 there is an n such that ¥ min{I[m].max[n].max | m ≤ n} - max{I[m].min[n].min | m ≤ n} < eps.Since eps/3 > 0, there is an k such that
width(I[k]) = I[k].max - I[k].min < eps/3
and therefore
I[k].max[n].min - I[k].min[n].max ≤ I[k].max - I[k].min < eps/3.
Furthermore there is an n1 such that
width(I[k].min[n1]) < eps/3
and an n2 such that
width(I[k].max[n2]) < eps/3.
Let n = max{k, n1, n2}. Then
width(I[k].min[n]) < eps/3
width(I[k].max[n]) < eps/3.
Adding together
width(I[k].max[n]) < eps/3
I[k].max[n].min - I[k].min[n].max < eps/3
width(I[k].min[n]) < eps/3
we obtain
I[k].max[n].max - I[k].min[n].min < eps.
Since k ≤ n, we have I[k].max[n].max ≥ min{I[m].max[n].max | m ≤ n} and I[k].min[n].min ≤ max{I[m].min[n].min | m ≤ n}. Thus
min{I[m].max[n].max | m ≤ n} - max{I[m].min[n].min | m ≤ n} ≤ I[k].max[n].max - I[k].min[n].min < eps.
proof the number constructed lies within all the intervalsWe need to show
¥ for any k, nested_RI_int(I, p, q) ∈ I[k].By nested_RI_int(I, p, q) ∈ I[k], we mean I[k].min ≤ nested_RI_int(I, p, q) ≤ I[k].max.
1. Assume to the contrary that I[k].min > nested_RI_int(I, p, q). Then there is an n such that I[k].min[n].min > nested_RI_int(I, p, q)[n].max. Let n' = max{n,k}; then
I[k].min[n'].min ≥ I[k].min[n].min > nested_RI_int(I, p, q)[n].max ≥ nested_RI_int(I, p, q)[n'].min = max{I[m].min[n'].min | m ≤ n'}.
But since k ≤ n', I[k].min[n'].min ≤ max{I[m].min[n'].min | m ≤ n'}, a contradiction.
2. Assume to the contrary that I[k].max < nested_RI_int(I, p, q). Then there is an n such that I[k].max[n].max < nested_RI_int(I, p, q)[n].min. Let n' = max{n,k}; then
I[k].max[n'].max ≤ I[k].max[n].max < nested_RI_int(I, p, q)[n].min ≤ nested_RI_int(I, p, q)[n'].max = min{I[m].max[n'].max | m ≤ n'}.
But since k ≤ n', I[k].max[n'].max ≥ min{I[m].max[n'].max | m ≤ n'}, a contradiction.
uniquenessWe need to show
¥ If I is a sequence of nested closed intervals whose widths converge to zero, and we have both x ∈ I[k] for all k and y ∈ I[k] for all k, then x = y.For this we won't need the nestedness part. So we can restate this with fewer conditions as
¥ If I is a sequence of closed intervals in which we can find an interval with width smaller than any positive real number eps, and we have both x ∈ I[k] for all k and y ∈ I[k] for all k, then x = y.(we can't say the widths converge to zero anymore because that's not true without the nestedness condition)
Assume to the contrary that x <> y. Either x > y or y > x. If x > y, let eps = x - y, and note eps > 0. Then there is a k such that I[k].max - I[k].min < eps. Since x ≤ I[k].max and y ≥ I[k].min, we have eps = x - y ≤ I[k].max - I[k].min, a contradition. If y > x, the argument is the same with x and y interchanged. Therefore x = y.
compatibility with equalityWe need to show
¥ if I1[k].min = I2[k].min and I1[k].max = I2[k].max for all k, then nested_RI_int(I1, p1, q1) = nested_RI_int(I2, p2, q2).We know nested_RI_int(I1, p1, q1) ∈ I1[k] for all k. Since the bounds for I1 and I2 are the same, nested_RI_int(I1, p1, q1) ∈ I2[k] for all k. Since we also have nested_RI_int(I2, p2, q2) ∈ I2[k] for all k, and the real number in this intersection is unique, it follows that nested_RI_int(I1, p1, q1) = nested_RI_int(I2, p2, q2).
Our proofs about nested real intervals are now complete.
No.11068
Going to make a conscious effort to go and reread through this thread again after having beefed up my mathematical knowledge a bit. Hopefully this time nothing goes over my head
No.11139
>>11068Thanks for the interest. Does everything make sense now?
I've been making progress toward getting a demo calculator site working using the stuff I've programmed so far, by converting the Coq code into OCaml and then converting the OCaml code to Javascript. One missing ingredient I need to add is some friendly output, something that can be used to extract decimal digits from a number.
Let's define a function round(x) that takes a real number as input and returns either the number rounded down or rounded up (which we get will depend not just on the value of the number but also on how it's computed). To do this, we just need to iterate through the sequence of intervals (using the Coq library function constructive_ground_ep
silon_nat) until we find the first natural number k such that
¥ floor(max(x[k])) ≤ ceil(min(x[k])).where ceil and floor are ceiling and floor functions for rational numbers. Then we just return
¥ floor(max(x[k])).We must first prove that such a k exists so that the iteration is guaranteed to terminate. From our definition of real numbers, we know there exists a k such that max(x[k]) - min(x[k]) < 1. For this k we have floor(max(x[k])) - ceil(min(x[k])) ≤ max(x[k]) - min(x[k]) < 1. Thus floor(max(x[k])) - ceil(min(x[k])) ≤ 0, and floor(max(x[k])) ≤ ceil(min(x[k])).
Next we want to show that the function works as intended, meaning that
¥ round(x) - 1 < x < round(x) + 1.For the first part:
round(x) - 1 = floor(max(x[k])) - 1 ≤ ceil(min(x[k])) - 1 < min(x[k]) ≤ x
For the second part:
round(x) + 1 = floor(max(x[k])) + 1 > max(x[k]) ≥ x
To extract n decimal digits of the number x, we can simply compute
¥ round(10^n * x)and format the result by putting the decimal point in the proper place. The result will either correct rounded down or rounded up.
Next step is to build a parser for the calculator input.
No.11169
>>11164I added a bit of documentation. Currently the default input is
¥ intersect (i => repeat i (x => [4 / (min x + max x), (min x + max x) / 2]) [1, 2])which computes the square root of two. You can see what's going on by trying out
¥ (i => repeat i (x => [4 / (min x + max x), (min x + max x) / 2]) [1, 2]) 0¥ (i => repeat i (x => [4 / (min x + max x), (min x + max x) / 2]) [1, 2]) 1¥ (i => repeat i (x => [4 / (min x + max x), (min x + max x) / 2]) [1, 2]) 2and so on to display the intervals it's computing which converge to sqrt(2).
No.11171
>>11169After the latest update this should be
¥ intersect (repeat (xs => let avg := (min xs + max xs) / 2 in [2 / avg, avg]) [1, 2])and
¥ repeat (xs => let avg := (min xs + max xs) / 2 in [2 / avg, avg]) [1, 2] 0¥ repeat (xs => let avg := (min xs + max xs) / 2 in [2 / avg, avg]) [1, 2] 1¥ repeat (xs => let avg := (min xs + max xs) / 2 in [2 / avg, avg]) [1, 2] 2to see how it works.
No.11177
>>9477Correction:
> Assuming Markov, x = y if and only if x <> y is true.¥ Assuming Markov, x = y is false if and only if x <> y is true.
No.11198
While fiddling with the expression in
>>11171 to see if I could turn it into a general square root function, I realized we need a way to define piecewise functions.
Specifically, I want to be able to do
¥ piecewise(a,f,g)and get a function such that
¥ piecewise(a,f,g)(x) = f(x) for x ≤ a¥ piecewise(a,f,g)(x) = g(x) for x ≥ a.You might wonder why we can't just do something like
¥ if x < a then f(x) else g(x).The problem is that when x = a exactly, the comparison requires infinite precision and never terminates. So we'll have to do things a bit differently.
We'll need the two parts meet at a common value:
¥ f(a) = g(a).Otherwise we would have to compute x to infinite precision in order to decide whether to use f or g.
I found I wasn't able to prove the convergence of the result without assuming a stronger condition than above, namely that
¥ if f(x) <> g(x), then x <> a.One way to prove this for a given a, f, and g would be to first prove the three conditions
¥ f(a) = g(a)¥ if f(x) <> f(a), then x <> a¥ if g(x) <> g(a), then x <> a.Let's first prove that for any x, y, and z,
¥ if x <> z, then x <> y or y <> z.If x < z, we have y < z or x < y. If z < x, we have y < x or z < y. In either case, x <> y or y <> z.
This also gives us a slightly shorter proof of the transitivity of equality than the one in
>>8925:
¥ If x = y and y = z, then x = z.Assume to the contrary that x <> z. Then x <> y or y <> z, both of which contradict the premises.
It will also be useful to extend this out to longer chains:
¥ If w <> z, then w <> x, x <> y, or y <> z.Proof: w <> z implies w <> x or x <> z. The second case implies x <> y or y <> z.
Back to proving that
¥ f(a) = g(a)¥ if f(x) <> f(a), then x <> a¥ if g(x) <> g(a), then x <> aimply
¥ if f(x) <> g(x), then x <> a.Suppose f(x) <> g(x). Then either f(x) <> f(a), implying x <> a, or f(a) <> g(a), contradicting our premises, or g(a) <> g(x), implying x <> a.
So far, when we've defined a function we've usually been proving that it's respectful of real number equality in the sense that
¥ if x = a, then f(x) = f(a).but now we're interested in
¥ if f(x) <> f(a), then x <> a.Classical logic would allow us to deduce the former from the latter (Markov's principle would also suffice per
>>8966). But we'd like to avoid invoking axioms if possible since they cause Coq's execution to get stuck. It might be useful to go back and prove the apartness version for some of the functions we've already defined. Alternatively we should be able to get it as a consequence of the usual definition of continuity of f at x = a, namely
¥ f is continuous at a if for every eps > 0 there exists a delta > 0 such that |x - a| < delta implies |f(x) - f(a)| < eps.If f(x) <> f(a), then |f(x) - f(a)| > 0, so there exists delta > 0 such that |x - a| < delta implies |f(x) - f(a)| < |f(x) - f(a)| and is therefore false. Thus |x - a| ≥ delta > 0 and x <> a.
But we need to construct absolute values first, at least if we want to use absolute values rather than having to write out things like a - delta < x < a + delta.
We'll define our piecewise function making function as
¥ piecewise(a,f,g,p)(x)[k] = f(x)[k] if x[k] < a[k]¥ piecewise(a,f,g,p)(x)[k] = g(x)[k] if x[k] > a[k]¥ piecewise(a,f,g,p)(x)[k] = [min {f(x)[k].min, g(x)[k].min}, max {f(x)[k].max, g(x)[k].max}]Q otherwise(remember: [a,b]Q < [c,d]Q if b < c)
where p is a proof that
¥ for all x, f(x) <> g(x) implies x <> a.As usual, we will need to show that this defines a real number. These are always kind of tedious, but hopefully I won't have to do too many more of these, and instead I'll be able to define new functions in terms of stuff I've already written. In particular, this piecewise function stuff will get us the absolute value function as well as functions returning the lesser and greater of two arguments.
No.11199
Some useful lemmas first:
¥ If x and y are real numbers and k2 ≥ k1, then x[k1] < y[k1] implies x[k2] < y[k2].Proof: x[k2].max ≤ x[k1].max < y[k1].min ≤ y[k2].min
This can also be used to simplify the proof of one of the lemmas in
>>9460:
¥ If a < b and c < d, then there is a natural number k such that a[k] < b[k] and c[k] < d[k].Suppose a < b and c < d. Then there is a k1 such that a[k1] < b[k1] and a k2 such that c[k2] < d[k2]. Let k be the larger of k1 and k2. Then a[k] < b[k] and c[k] < d[k].
Another lemma, this one extracted from the proof of
>>8969:
¥ Given two nonempty closed rational intervals u and v, if neither u < v nor v < u, there is a rational number r such that r ∈ u and r ∈ v, specifically max {u.min, v.min}.Clearly r ≥ u.min and r ≥ v.min. Either r = min(x[k]) or r = min(y[k]). If r = u.min, then we have r = u.min ≤ u.max since u is nonempty, and r = u.min ≤ v.max since v < u is false. Similarly, if r = v.min, we have r = v.min ≤ v.max since v is nonempty, and we have r = v.min ≤ u.max since u < v is false. Thus r ∈ u and r ∈ v.
A third lemma will be useful to show that our first and second cases are actually distinct:
¥ Given two nonempty closed rational intervals u and v, if u < v, then it is not the case that v < u.Proof: u.min ≤ u.max < v.min ≤ v.max, so v.max < u.min is false.
As the last lemma, we note that
¥ if r ∈ [a, b]Q, c ≤ a, and b ≤ d, then r ∈ [c, d]Qsince c ≤ a ≤ r and r ≤ b ≤ d
which we can extend to work on two elements at a time:
¥ If r, s ∈ [a, b]Q, c ≤ a, and b ≤ d, then r, s ∈ [c, d]Q.
No.11200
Now back to the main proof.
First, we must prove that the intervals are nested:
¥ If k2 ≥ k1, then piecewise(a,f,g,p)(x)[k2].min ∈ piecewise(a,f,g,p)(x)[k1] and piecewise(a,f,g,p)(x)[k2].max ∈ piecewise(a,f,g,p)(x)[k1].
Suppose k2 ≥ k1. There are three cases.
1) If x[k1] < a[k1], then x[k2] < a[k2]. Thus piecewise(a,f,g,p)(x)[k2] = f(x)[k2], whose minimum and maximum are both in f(x)[k1] = piecewise(a,f,g,p)(x)[k1].
2) If x[k1] > a[k1], then x[k2] > a[k2]. Thus piecewise(a,f,g,p)(x)[k2] = g(x)[k2], whose minimum and maximum are both in g(x)[k1] = piecewise(a,f,g,p)(x)[k1].
3) Otherwise, piecewise(a,f,g,p)(x)[k1] = [min {f(x)[k1].min, g(x)[k1].min}, max {f(x)[k1].max, g(x)[k1].max}]Q.
It suffices to show that piecewise(a,f,g,p)(x)[k2].min and piecewise(a,f,g,p)(x)[k2].max are within
u = [min {f(x)[k2].min, g(x)[k2].min}, max {f(x)[k2].max, g(x)[k2].max}]Q.
Once we have that, we know that they are within piecewise(a,f,g,p)(x)[k1] since
f(x)[k1].min ≤ f(x)[k2].min and f(x)[k1].max ≤ f(x)[k2].max and
g(x)[k1].min ≤ g(x)[k2].min and g(x)[k1].max ≤ g(x)[k2].max
imply
min {f(x)[k1].min, g(x)[k1].min} ≤ min {f(x)[k2].min, g(x)[k2].min} and max {f(x)[k2].max, g(x)[k2].max} ≤ max {f(x)[k1].max, g(x)[k1].max}.
We have three subcases:
3a) If x[k2] < a[k2], then piecewise(a,f,g,p)(x)[k2] = f(x)[k2].
Since f(x)[k2] is nonempty, its min and max are within f(x)[k2].
Since min {f(x)[k2].min, g(x)[k2].min} ≤ f(x)[k2].min and f(x)[k2].max ≤ max {f(x)[k2].max, g(x)[k2].max}, they are also within u.
3b) If x[k2] > a[k2], then piecewise(a,f,g,p)(x)[k2] = g(x)[k2].
Since g(x)[k2] is nonempty, its min and max are within g(x)[k2].
Since min {f(x)[k2].min, g(x)[k2].min} ≤ f(x)[k2].min and f(x)[k2].max ≤ max {f(x)[k2].max, g(x)[k2].max}, they are also within u.
3c) If x[k2] > a[k2], then piecewise(a,f,g,p)(x)[k2] = u.
We know u is nonempty since min {f(x)[k2].min, g(x)[k2].min} ≤ f(x)[k2].min ≤ f(x)[k2].max ≤ max {f(x)[k2].max, g(x)[k2].max}.
Therefore the min and max of u are within u.
Second, we must prove that the widths of the intervals converge to zero:
¥ For every rational eps > 0 there is a k such that piecewise(a,f,g,p)(x)[k].max - piecewise(a,f,g,p)(x)[k].min < eps.
There is a k1 such that width(f(x)[k1]) < eps/2 and a k2 such that width(g(x)[k2]) < eps/2. Consider two cases:
1) Either f(x)[k1] < g(x)[k2] or g(x)[k2] < f(x)[k1].
Then we have f(x) <> g(x), and it follows that x <> a. Two subcases:
1a) x < a
Then there is a k3 such that x[k3] < a[k3]. Let k = max {k1, k3}; we have x[k] < a[k] and thus width(piecewise(a,f,g,p)(x)[k]) = width(f(x)[k]) ≤ width(f(x)[k1]) < eps/2 < eps.
1b) x > a
Then there is a k3 such that x[k3] > a[k3]. Let k = max {k2, k3}; we have x[k] > a[k] and thus width(piecewise(a,f,g,p)(x)[k]) = width(g(x)[k]) ≤ width(g(x)[k2]) < eps/2 < eps.
The remaining case:
2) Neither f(x)[k1] < g(x)[k2] nor g(x)[k2] < f(x)[k1].
Then there is a rational number r = max {f(x)[k1].min, g(x)[k2].min} such that r ∈ f(x)[k1] and r ∈ g(x)[k2]. Let k = max {k1, k2}.
We must consider three subcases:
2a) If x[k] < a[k], then
width(piecewise(a,f,g,p)(x)[k]) = width(f(x)[k]) ≤ width(f(x)[k1]) < eps/2 < eps.
2b) If x[k] > a[k], then
width(piecewise(a,f,g,p)(x)[k]) = width(g(x)[k]) ≤ width(g(x)[k2]) < eps/2 < eps.
2c) Otherwise:
Since r ≥ f(x)[k1].min and f(x)[k].max ≤ f(x)[k1].max, we have f(x)[k].max - r ≤ f(x)[k1].max - f(x)[k1].min < eps/2.
Since r ≥ g(x)[k2].min and g(x)[k].max ≤ g(x)[k2].max, we have g(x)[k].max - r ≤ g(x)[k2].max - g(x)[k2].min < eps/2.
We know that max {f(x)[k].max, g(x)[k].max} is either f(x)[k].max or g(x)[k].max. In either case, max {f(x)[k].max, g(x)[k].max} - r < eps/2.
Since r ≤ f(x)[k1].max and f(x)[k].min ≥ f(x)[k1].min, we have r - f(x)[k].min ≤ f(x)[k1].max - f(x)[k1].min < eps/2.
Since r ≤ g(x)[k2].max and g(x)[k].min ≥ g(x)[k2].min, we have r - g(x)[k].min ≤ g(x)[k2].max - g(x)[k2].min < eps/2.
We know that min {f(x)[k].min, g(x)[k].min} is either f(x)[k].min or g(x)[k].min. In either case, r - min {f(x)[k].min, g(x)[k].min} < eps/2.
Thus width(piecewise(a,f,g,p)(x)[k]) = max {f(x)[k].max, g(x)[k].max} - min {f(x)[k].min, g(x)[k].min} = (max {f(x)[k].max, g(x)[k].max} - r) + (r - min {f(x)[k].min, g(x)[k].min}) < eps/2 + eps/2 = eps.
Our function returns real numbers, as it should!
No.11201
A next obvious thing to do is to prove that our piecewise function making function behaves as we want; that is, that
¥ x ≤ a implies piecewise(a,f,g,p)(x) = f(x) and
¥ x ≥ a implies piecewise(a,f,g,p)(x) = g(x).
It turns out to be more useful to first show
¥ piecewise(a,f,g,p)(x) <> f(x) implies x > a and
¥ piecewise(a,f,g,p)(x) <> g(x) implies x < a.
1) Suppose piecewise(a,f,g,p)(x) <> f(x).
Then there is a k such that piecewise(a,f,g,p)(x)[k] < f(x)[k] or piecewise(a,f,g,p)(x)[k] > f(x)[k].
There are three cases:
1a) If x[k] < a[k], then
piecewise(a,f,g,p)(x)[k] = f(x)[k], so we have f(x)[k] < f(x)[k] meaning f(x)[k].max < f(x)[k].min, which is impossible since f(x)[k] is nonempty.
1b) If x[k] > a[k], then
x > a, and we are done.
1c) Otherwise,
piecewise(a,f,g,p)(x)[k] = [min {f(x)[k].min, g(x)[k].min}, max {f(x)[k].max, g(x)[k].max}]Q, so we have
max {f(x)[k].max, g(x)[k].max} < f(x)[k].min ≤ f(x)[k].max or
f(x)[k].min ≤ f(x)[k].max < min {f(x)[k].min, g(x)[k].min},
both of which are impossible.
2) Suppose piecewise(a,f,g,p)(x) <> g(x).
This is substantially identical to (1), but I've written it out anyway. There are again three cases:
2a) If x[k] < a[k], then
x < a, and we are done.
2b) If x[k] > a[k], then
piecewise(a,f,g,p)(x)[k] = g(x)[k], so we have g(x)[k].max < g(x)[k].min, which is impossible.
2c) Otherwise,
piecewise(a,f,g,p)(x)[k] = [min {f(x)[k].min, g(x)[k].min}, max {f(x)[k].max, g(x)[k].max}]Q, so we have
max {f(x)[k].max, g(x)[k].max} < g(x)[k].min ≤ g(x)[k].max or
g(x)[k].min ≤ g(x)[k].max < min {f(x)[k].min, g(x)[k].min},
both of which are impossible.
The originally desired results
¥ x ≤ a implies piecewise(a,f,g,p)(x) = f(x) and
¥ x ≥ a implies piecewise(a,f,g,p)(x) = g(x).
are obtained by taking the contrapositive.
Another thing we want to prove is that our piecewise function is compatible with equality. Let's prove a similar result involving apartness first:
¥ If piecewise(a1,f1,g1,p1)(x1) <> piecewise(a2,f2,g2,p2)(x2), then a1 <> a2, f1(x1) <> f2(x2), g1(x1) <> g2(x2), or x1 <> x2.
Suppose piecewise(a1,f1,g1,p1)(x1) <> piecewise(a2,f2,g2,p2)(x2). Then piecewise(a1,f1,g1,p1)(x1) <> f1(x1), f1(x1) <> f2(x2), or f2(x2) <> piecewise(a2,f2,g2,p2)(x2). From these we can conclude that
x1 > a1, f1(x1) <> f2(x2), or x2 > a2.
Similarly, we have piecewise(a1,f1,g1,p1)(x1) <> g1(x1), g1(x1) <> g2(x2), or g2(x2) <> piecewise(a2,f2,g2,p2)(x2). We can conclude that
x1 < a1, g1(x1) <> g2(x2), or x2 < a2.
In the cases where f1(x1) <> f2(x2) or g1(x1) <> g2(x2), we are done. The cases where x1 > a1 and x1 < a1 or where x2 > a2 and x2 < a2 are contradictory. The remaining cases are:
1) x1 > a1 and x2 < a2
From x2 < a2 we have either a1 < a2, and we are done, or x2 < a1, implying x2 < a1 < x1.
2) x2 > a2 and x1 < a1
From x1 < a1 we have either a2 < a1, and we are done, or x1 < a2, implying x1 < a2 < x2.
We can now prove that
¥ If a1 = a2, f1(x1) = f2(x2), g1(x1) = g2(x2), and x1 = x2, then piecewise(a1,f1,g1,p1)(x1) = piecewise(a2,f2,g2,p2)(x2).
Assume to the contrary that piecewise(a1,f1,g1,p1)(x1) <> piecewise(a2,f2,g2,p2)(x2). Then a1 <> a2, f1(x1) <> f2(x2), g1(x1) <> g2(x2), or x1 <> x2, all of which contradict our premises.
No.11202
I've added the piecewise function generating function to the calculator demo:
https://ccd0.github.io/toyreals0/So you can now do things like this:
let abs := piecewise 0 (x => -x) (x => x) in abs (-4.5)
No.11360
>>9012Been thinking about this question again. Haven't solved it, but I noticed we can remove this condition:
>Suppose also that we know that at least one of the programs (possibly both) does not halt.Suppose we have the desired algorithm, and we feed it two computer programs, both of which halt. In the original question, the behavior of the algorithm is unspecified, so it may give either answer, or some nonsense answer, or simply never halt. We can produce a modified algorithm by running the original algorithm in parallel with programs A and B. If both A and B halt before the original algorithm halts, or if the original algorithm halts first with nonsense output, let the modified algorithm output the choice "A" (arbitrarily chosen, "B" would work just as well for this argument). Otherwise, let the modified algorithm await the result of the original algorithm and halt with that output. The modified algorithm is guaranteed to halt in all cases, and is guaranteed to output "A" if A does not halt but B does halt, and is guaranteed to output "B" if B does not halt but A does halt. Furthermore, any algorithm with this property meets the original specification.
Thus we can restate the problem as follows:
¥Is it possible to write an algorithm which, given two computer programs A and B, correctly concludes, in a finite amount of time, either that "if A halts then B halts" or that "if B halts then A halts"? If it's not possible, how can we prove that?
No.11361
>>11360I think I've got it using a variation on the original halting problem argument.
Suppose we have a function compare_halt with four arguments
¥compare_halt(prog0, data0, prog1, data1)satisfying the specification
¥if prog(data0) halts and prog(data1) does not halt, the output is 0¥if prog(data1) halts and prog(data0) does not halt, the output is 1¥otherwise the output can be either 0 or 1.Consider the program
function F([i, prog]) {
if compare_halt(prog, [0, prog], prog, [1, prog]) == i then
infinite_loop()
else
return
}
If compare_halt(F, [0, F], F, [1, F]) evaluates to 0, then F([0, F]) hangs and F([1, F]) halts.
But the specification says compare_halt(F, [0, F], F, [1, F]) must evaluate to 1 in this case.
If compare_halt(F, [0, F], F, [1, F]) evaluates to 1, then F([0, F]) halts and F([1, F]) hangs.
But the specification says compare_halt(F, [0, F], F, [1, F]) must evaluate to 0 in this case.
Thus the proposed compare_halt function is impossible.
No.11364
>>11361Some corrolaries.
¥There is no general algorithm which, given two programs that output infinite decimals as streams, computes the infinite decimal representing their sum.Let A and B be two computer programs.
Let x start with "0." followed by 0's until program A halts, at which point the digits change to 1's.
Let y start with "0." followed by 9's until program B halts, at which point the digits change to 8's.
If A halts and B does not halt, then x+y > 1, and the ones place of x+y must be 1. If B halts and A does not halt, then x+y < 1, and the ones place of x+y must be 0. Thus there is no way to calculate the ones place of x+y.
Note that it's possible to get around this problem by using more than 10 digits. For example, infinite decimals built using the digits -9 through 9, like I talked about in
>>9462, don't have this problem.
¥There is no general algorithm which converts an object of our real number type to a stream of digits giving an infinite decimal representation of the number.In
>>9596 I mentioned I didn't know a proof of this; now we have one. We can easily convert a stream of digits into a stream of nested rational intervals. For example, 3.14... becomes [3,4], [3.1,3.2], [3.14,3.15], ... . If we could convert back into an infinite decimal stream, then we could take the two infinite decimal streams from the previous proof, convert them into nested interval streams, add them, then convert to the desired inifinite decimal stream.
Note that there would be no problem converting to infinite decimal form if we allow the digits -9 through 9. Also, there's no problem with doing what's done now in
https://ccd0.github.io/toyreals0/which is to produce the number of digits desired which the guarantee that the result is either rounded up or rounded down. This is different from outputting an infinite stream of digits since a result like
1.000
can change to
0.9999
when another digit is required.
¥There is no general algorithm which, given two numbers x and y for which x*y = 0, correctly names either x or y as the number that equals 0.As I mentioned in
>>9015, this was the original problem that got me thinking about this. Let A and B be two computer programs. Let x[k] = [0, 1/k] if A has not halted by the kth step, and x[k] = [1/n, 1/n] if A halts at step n and k ≥ n. Define y from B similarly. Under this construction, x=0 if and only if A halts, and similarly for y and B. If we know x*y = 0, we know either x=0 or y=0, but we don't necessarily know which. Suppose we did have a procedure which, given x and y for which x*y = 0, could correctly conclude "x=0" or "y=0". This is the same as concluding correctly that "A does not halt" or "B does not halt" under the condition that at least one of A and B do not halt. By the argument in
>>11360, this is equivalent to the impossible algorithm in
>>11361.
No.11942
>>9444I was thinking about how to hand-multiply infinite (signed-digit) decimals again. Pic related might be more work than necessary, but it does have the advantage of making it less confusing. It also has the advantage of giving the greatest possible number of digits for a fixed number of digits of the input, which might be helpful if further digits of the numbers you're multiplying are difficult to compute.
Most of the process should be self-explanatory, but some things I should explain. First of all, obviously we can't multiply infinitely many digits. The goal here is a process that if carried on long enough, could give you arbitrarily many digits of the answer, assuming you can get the required number of digits of the two numbers you're multiplying.
Second, as mentioned in
>>9462, I'm using signed digits. Digits can be anything in {-9,-8,-7,-6,-5,-4,-3,
-2,-1,0,1,2,3,4,5,6,7,
8,9}. To represent the negative digits, I draw a line over the digit. Everything is still in base 10, but there are now more ways to represent the same number. For example, early on in the computation, I add 140 + 56 and write the answer as 20(-4) rather than 196. When adding the numbers, I chose to convert the 6 to 1(-4) and carry the 1. I'll explain why I did that in a moment.
When a signed-digit infinite decimal number is truncated to a finite number of digits, the uncertainty in the number is ±1 in the final digit. For example, 1.414 could be the truncation of any number between 1.414[(-9)(-9)(-9),rep
eating] and 1.414[999,repeating] inclusive, which are the same thing as 1.413 and 1.415 respectively. At various stages in the calculation, I've computed the uncertainty in the answer at that point. For example, when multiplying numbers truncated to 1.414*1.414, the result is 2.00(-1)404 with an uncertainty of ±0.002829. The uncertainty can be calculated by adding 1414+1414+1. By considering the uncertainty, we can figure out which digits can be taken as final digits of the answer. At the 1.414*1.414 stage, the digits 2.00 can be taken as final since 2.00(-1)404 ± 0.002829 is guaranteed to be between 2.0(-1) and 2.01 inclusive. In the pic, I've drawn boxes around the digits which have been taken as final.
This should explain why I wrote the answer to 1.4*1.4 as 2.0(-4) instead of 1.96. The first digit of 2.0(-4) can be taken as final since 2.0(-4) ± 0.29 is guaranteed to be between 2.(-1) and 2.1 inclusive. But the first digit of 1.96 ± 0.29 cannot be taken as final. If the answer had been written as 1.96, I would have had to rewrite it as 2.(-1)6 or 2.0(-4) in order to take the first digit as final. To increase the likelihood that I would get digits that can be taken as final, I was choosing to write digits in the range {-5,-4,-3,-2,-1,0,1,2,
3,4,5} when possible.
No.11947
>>11943You're probably underrating your mental capacity. Some of the stuff in this thread might be complicated, but
>>11942 is just me getting closer and closer to the result of a multiplication problem by repeatedly adding a digit to each of the two numbers I'm multiplying.
1 * 1 = 1
1.4 * 1 = 1.4
1.4 * 1.4 = 2.0(-4) [another way of writing 1.96]
1.41 * 1.4 = 2.0(-3)4 [another way of writing 1.974]
and so on indefinitely.
In this case the two numbers I'm multiplying are both the square root of 2, which is
1.41421356237309504880
16887... (continues infinitely)
so the result should be 2. But you could use the same algorithm to multiply any two infinite decimals.
The ± thing is me calculating how far the current result could possibly be from the correct answer, which lets me know how many of digits I've calculated so far are necessarily correct. I drew a box around the digits I know are correct at each stage.
No.11996
>>9731I found a paper they wrote about that functionality after they implemented it:
https://cacm.acm.org/magazines/2017/8/219594-small-data-computing/fulltextIt's an interesting read. Also it usefully gives the error guarantees on the calculation:
>We wanted to ensure at a minimum that the displayed final answer was never off by one or more in the final displayed digit.That's what I expected, but it's nice to know it instead of guessing. It's also the same standard I used for
>>11164.
They made use of a preexisting Java library to do the calculations:
https://www.hboehm.info/crcalc/
No.12030
I want to try to get to the answer of questions like
>>8932 about precisely what axioms we need to add to Coq in order to reason about "all" the real numbers as discussed in
>>8919, in the sense that we can prove the versions of the completeness property commonly used in classical mathematics. After that, maybe we'll look at countability and subcountability.
In order to do that, I need to finish off what I planned out in
>>9526 and write proofs of some more of those properties. Some of those properties require adding axioms to Coq, and some don't. Without using any additional axioms, we've already proven
> (1) The Archimedean propertyin
>>9532 and
> (17) The Shrinking Interval Propertyin
>>9598 and the posts that follow. In classical mathematics these two together are enough to prove the other properties, but since Coq uses only constructive reasoning by default, we'll need to use axioms for some of them.
The next property I want to attack is
> (11) The Convergence of Cauchy Sequences: Every Cauchy sequence in R is convergent.Both "Cauchy sequence" and "convergent" are usually defined using the absolute value function. So we'll have to define that first.
No.12031
The absolute value function should satisfy
¥ |x| = -x for x ≤ 0¥ |x| = x for x ≥ 0.We've already defined a function for making piecewise functions in
>>11198. We can use this to define the absolute value as
¥ |x| = piecewise(0, (x => -x), (x => x), p)(x)where p is the necessesary proof of
¥ if -x <> x, then x <> 0which can be obtained by subtracting x from both sides, then dividing by -2.
It follows immediately from the theorems we've already proven about the piecewise function making function that our absolute value function is compatible with equality
¥ x = y implies |x| = |y|and that
¥ |x| = -x for x ≤ 0¥ |x| = x for x ≥ 0as desired.
Now comes the time to prove theorems about the absolute value function. To avoid spending too long on this, for now I'm going to limit the list to things which I know I need or for which I've needed the analogous theorem in the rational numbers. We can add more theorems later as needed. For now we want to show:
¥ |x| ≥ 0¥ |-x| = |x|¥ x ≤ |x|¥ |x + y| ≤ |x| + |y|¥ |x * y| = |x| * |y|¥ |x - y| ≤ z if and only if x ∈ [y - z, y + z]The obvious way to prove theorems like these in classical logic would be to split into the two cases x ≤ 0 and x ≥ 0, and prove the results in each case. For a moment it seems that not assuming the excluded middle will get in our way, since we don't have a proof that x ≤ 0 or x ≥ 0, only of its double negation. Fortunately it's not as bad as it looks. All statements involving ≤ and = are negative statements; we have defined ≤ as not > and = as not <>. It turns out that in intuitionistic logic, if we want to prove a negative statement, then we can use any fact that we've proven the double negation of. To say this more precisely,
¥ If P implies not Q, then not not P implies not Q.Proof: Given that P implies not Q, and given not not P, assume to the contrary that Q is true. If we also assume P, then we obtain not Q, which contradicts Q; thus we have not P. But not P contradicts not not P; from this contradiction we conclude not Q.
Since we have the double negation of x ≤ y or x ≥ y from
>>8947, an immediate corrolary is that
¥ given any statement P, we can prove not P by proving that not P follows from x ≤ y and that not P follows from x ≥ y.We can now grind through the theorems:
¥ |x| ≥ 0If x ≤ 0, then |x| = -x ≥ 0.
If x ≥ 0, then |x| = x ≥ 0.
¥ |-x| = |x|If x ≤ 0, then -x ≥ 0; thus |-x| = -x = |x|.
If x ≥ 0, then -x ≤ 0; thus |-x| = -(-x) = x = |x|.
¥ x ≤ |x|If x ≤ 0, then 2x ≤ 0 and x ≤ -x = |x|.
If x ≥ 0, then x = |x|.
¥ |x + y| ≤ |x| + |y|If x + y ≤ 0, then |x + y| = -(x + y) = (-x) + (-y) ≤ |-x| + |-y| = |x| + |y|.
If x + y ≥ 0, then |x + y| = x + y ≤ |x| + |y|.
¥ |x * y| = |x| * |y|If x ≤ 0 and y ≤ 0, then -x * -y ≥ 0; thus |x * y| = |-x * -y| = -x * -y = |x| * |y|.
If x ≤ 0 and y ≥ 0, then -x * y ≥ 0; thus |x * y| = |-x * y| = x * -y = |x| * |y|.
If x ≥ 0 and y ≤ 0, then x * -y ≥ 0; thus |x * y| = |x * -y| = x * -y = |x| * |y|.
If x ≥ 0 and y ≥ 0, then x * y ≥ 0; thus |x * y| = x * y = |x| * |y|.
¥ |x - y| ≤ z if and only if x ∈ [y - z, y + z]Suppose |x - y| ≤ z.
Then -(x - y) ≤ |-(x - y)| = |x - y| ≤ z; thus -z ≤ x - y and y - z ≤ x.
Also x - y ≤ |x - y| ≤ z, so x ≤ y + z.
Suppose x ∈ [y - z, y + z], meaning y - z ≤ x ≤ y + z.
If x - y ≤ 0, then |x - y| = -(x - y). Since -z ≤ x - y, we have |x - y| = -(x - y) ≤ z.
If x - y ≥ 0, then |x - y| = x - y ≤ z.
No.12033
An anon on IRC asked if I could explain why 0.999... = 1. So I'm going to switch tracks to infinite decimals for a bit.
We can, of course, give a definition of what a infinite decimal means and dryly conclude from our definition that 0.999... = 1. I think this is not particularly enlightening because it answers the wrong question. What we really want to know is why it is pragmatic to define infinite decimals in such a manner that 0.999... = 1.
Let's start by reminding ourselves why we need infinite decimals in the first place. The idea of decimal fractions was popularized in the West by Simon Stevin in a 1585 booklet called De Thiende. He described how to do arithmetic with these decimals, which was much simpler than arithmetic with general fractions. Eventually he demonstrates how to do the problem that we would today write as 0.4 ÷ 0.03. He notes that the calculation produces infinitely many 3's, and pragmatically recommends truncating the calculation at the precision required. Centuries later, you were likely introduced to infinite decimals by a similar calculation. Let's look at it in more detail.
Using the modern algorithm, we would begin by converting 0.4 ÷ 0.03 to the equivalent division problem 40 ÷ 3. We then ask how many times 3 goes into 4, the tens place of 40; the answer is 1 time. We write 1 in the tens place of our answer, and subtract 10*3=30 from 40, obtaining the remainder 10. From the presence of the remainder we know that the answer is greater than 10. But we also know at this point of the calculation that the answer is less than 20; otherwise the 3 would have gone in 2 times instead of 1 time. We can write
¥ 10 < 0.4 ÷ 0.03 < 20.
At the next step in the division process, when we see that 3 goes into 10 3 times but not 4 times, and there is still a remainder, we know that
¥ 13 < 0.4 ÷ 0.03 < 14.
Continuing the calculation, we learn that
¥ 13.3 < 0.4 ÷ 0.03 < 13.4
¥ 13.33 < 0.4 ÷ 0.03 < 13.34
¥ 13.333 < 0.4 ÷ 0.03 < 13.334
and so on for as far as we care to compute. Although the division algorithm never gives us an exact answer, it does give us an arbitrarily extendable list of upper and lower bounds on the answer.
For this division problem, we could have used fractions to obtain an exact answer 13 1/3. But some quantities can't be expressed as a fraction, at least not if we restrict the numerator and denominator to integers (whole numbers, possibly negative). For example, there is no fraction meeting those conditions whose square is 2, even though we need a square root of 2 to compute basic things like the diagonal of a square. We can make a similar calculation for the square root of 2. Since 1^2 = 1 is too small and 2^2 = 4 is too large, the square root of 2 must be between 1 and 2:
¥ 1 < √2 < 2
And since 1.4^2 = 1.96 is too small while 1.5^2 = 2.25 is too large, we have
¥ 1.4 < √2 < 1.5.
We can continue:
¥ 1.41 < √2 < 1.42
¥ 1.414 < √2 < 1.415
¥ 1.4142 < √2 < 1.4143
¥ 1.41421 < √2 < 1.41422
¥ 1.414213 < √2 < 1.414214
...
You might wonder if we can invent a quantity ε such that
¥ 0 < ε < 1
¥ 0 < ε < 0.1
¥ 0 < ε < 0.01
¥ 0 < ε < 0.001
and so on indefinitely. I'll examine this more in the next installment.
For now, was that clear enough for everyone to understand? Any questions?
No.12035
>>12033Let's assume for a moment that a number like ε satisfying
¥ 0 < ε < 1¥ 0 < ε < 0.1¥ 0 < ε < 0.01¥ 0 < ε < 0.001,and so on indefinitely, exists, and let's look at some properties that number would have.
I won't be proving these in Coq, at least for the moment, because we're talking about number systems that are different from the one I'm describing in the project. The basic assumptions I'm making about a number system containing ε are that it satisfies the properties of an ordered field, which means all the properties listed in
>>9492 except the least-upper-bound property.
First of all, ε is smaller than any positive rational number.
(A rational number is a number that can be written as a fraction with integers for the numerator and denominator. Since we are not French speakers, positive means greater than zero, and negative means less than zero; zero itself is neither positive nor negative.)
The proof: Given a positive rational number p/q, let n be the number of digits of q. Then 10^n, that is, 1 followed by n zeros, will be greater than q, and 1/10^n will be less than p/q. Since ε < 1/10^n, it is also less than p/q.
Since ε is positive, it's also obvious that ε is larger than any negative rational number.
Definition:
¥ An infinitesimal number is a number smaller than every positive rational number and larger than every negative rational number.Our hypothetical number ε is an infinitesimal number by this definition. So is zero.
Let's look at what happens when we multiply ε by 2. We should expect that
¥ 0 < 2ε < 2¥ 0 < 2ε < 0.2¥ 0 < 2ε < 0.02¥ 0 < 2ε < 0.002,and so on indefinitely. Notice that 0.2 is less than 1, 0.02 is less than 0.1, and so on. We can conclude
¥ 0 < 2ε < 1¥ 0 < 2ε < 0.1¥ 0 < 2ε < 0.01,meaning that 2ε satisfies the same upper and lower bounds that ε did. By the same logic we used to show ε was an infinitesimal number, 2ε is also an infinitesimal number.
In fact, multiplying an infinitesimal number by any rational number will give us another infinitesimal number. Let x be an infinitesimal number. For any positive integers p and q, by definition we have
-p/q < x < p/q.
Let's see what happens when we multiply x by the positive rational number r/s, with r and s being positive integers. Let p and q be arbitrary positive integers. Since p*s and q*r are both positive integers, we have
-(p*s)/(q*r) < x < (p*s)/(q*r).
Multiplying both inequalities by r/s, we get
-p/q < x*(r/s) < p/q.
Thus x*(r/s) is also an infinitesimal number.
The same thing happens if we multiply x by a negative number -r/s, with r and s being positive integers again. Let p and q be arbitrary positive integers, and we again have
-(p*s)/(q*r) < x < (p*s)/(q*r).
Multiplying both inequalities by -r/s, we get
p/q > x*(-r/s) > -p/q.
Since we multiplied by a negative number, the directions of the inequalities flip. But the result is the same; x*(-r/s) is an infinitesimal number.
The last case is multiplying by 0. Since x*0 = 0 and 0 is infinitesimal, this case works too.
Another notable feature of ε is that if its reciprocal exists, it will be larger than any positive integer:
¥ 1/ε > 1¥ 1/ε > 10¥ 1/ε > 100¥ 1/ε > 1000...
Any positive integer number we can name, we can take 10^n where n is the number of digits in that positive number, and get a bigger positive number, and we've just said that 1/ε is even bigger than that.
Yet another thing to know about ε: When dividing 0.4 ÷ 0.03, we found that
¥ 13 < 0.4 ÷ 0.03 < 14¥ 13.3 < 0.4 ÷ 0.03 < 13.4¥ 13.33 < 0.4 ÷ 0.03 < 13.34¥ 13.333 < 0.4 ÷ 0.03 < 13.334and so on indefinitely. If we add ε to the quotient, then we have
¥ 0.4 ÷ 0.03 + ε < 13.4 + 0.1 = 13.5¥ 0.4 ÷ 0.03 + ε < 13.34 + 0.01 = 13.35¥ 0.4 ÷ 0.03 + ε < 13.334 + 0.001 = 13.335and so on.
Since 13.5, 13.35, and 13.335 are less than 14, 13.4, and 13.34 respectively, we also have
¥ 13 < 0.4 ÷ 0.03 + ε < 14¥ 13.3 < 0.4 ÷ 0.03 + ε < 13.4¥ 13.33 < 0.4 ÷ 0.03 + ε < 13.34and so on. Thus 0.4 ÷ 0.03 + ε satisfies the same bounds we found for the original number 0.4 ÷ 0.03.
The same situation applies to √2 + ε. Here it goes even further:
¥ for any two rational numbers that √2 is between, √2 + ε is between the same rational numbers.Proof: Assume to the contrary that there are rational numbers p/q and r/s (p,q,r,s integers) such that
p/q < √2 < r/s
but
r/s ≤ √2 + ε.
Then
2 < (r/s)^2 < (√2 + ε)^2
2 < r^2/s^2 < 2 + 2ε√2 + ε^2
0 < r^2/s^2 - 2 < 2ε√2 + ε^2
0 < (r^2-2s^2)/s^2 < 2ε√2 + ε^2 = ε(2√2 + ε) < 3ε < every positive rational.
This is a contradiction; if (r^2-2s^2)/s^2 is positive and rational, it cannot be be less than every positive rational number.
No.12036
Correction: There are some < signs in
>>12035 that should be ≤ signs.
Instead of
> 2 < (r/s)^2 < (√2 + ε)^2> 2 < r^2/s^2 < 2 + 2ε√2 + ε^2> 0 < (r^2-2s^2)/s^2 < 2ε√2 + ε^2 = ε(2√2 + ε) < 3ε < every positive rational.it should say
2 < (r/s)^2 ≤ (√2 + ε)^2
2 < r^2/s^2 ≤ 2 + 2ε√2 + ε^2
0 < (r^2-2s^2)/s^2 ≤ 2ε√2 + ε^2 = ε(2√2 + ε) < 3ε < every positive rational.
No.12037
>>12033>>12035So to answer the question of whether there's a number system with a number ε such that
¥ 0 < ε < 1¥ 0 < ε < 0.1¥ 0 < ε < 0.01¥ 0 < ε < 0.001,and so on, the answer is yes, there are many. There are the hyperreal numbers which are useful as an alternate way to do calculus. There are the surreal numbers which arose out of game theory. And you can even just postulate such a number ε and add it to the reals sort of like how we add i to make the complex numbers. In the case of the complex numbers, you get the complex numbers from all the linear functions of i. If you add ε, your new set of numbers consists of all the rational functions (polynomial divided by polynomial) of ε.
But we can see that a nonzero infinitesimal number like ε will cause trouble if we want our numbers to be expressed by infinite decimals. The whole idea behind infinite decimals is that we can identify a number by comparing it with finite decimals. If we allow ε into our system, then we can no longer distinguish ε from 2ε, or 0.4 ÷ 0.03 from 0.4 ÷ 0.03 + ε, or √2 from √2 + ε by comparing them with finite decimals. We would need something else. For example, in the surreal numbers, ε is the simplest number between {0} and {1, 1/2, 1/4, 1/8, ...}, and 2ε is the simplest number between {ε} and {1, 1/2, 1/4, 1/8, ...}.
Another definition:
¥ Two numbers are infinitesimally close if the difference between them is infinitesimal.There are many equivalent ways to construct the real numbers, but all of them are designed so that we never construct a real number infinitesimally close to another real number. I don't think anyone knows whether it's possible for two real-life quantities (such as distances, times, weights, and volumes) to be infinitesimally close to each other yet not equal. If it is possible, then when we model these infinitesimally close quantities with real numbers, we are choosing to ignore any infinitesimal difference and assign the same real number to both.
A simple way to avoid constructing numbers infinitesimally close to other numbers is to change the less-than signs in the construction of √2
> 1.41 < √2 < 1.42> 1.414 < √2 < 1.415> 1.4142 < √2 < 1.4143> 1.41421 < √2 < 1.41422> 1.414213 < √2 < 1.414214> ...to at-most signs:
¥ 1.41 ≤ √2 ≤ 1.42¥ 1.414 ≤ √2 ≤ 1.415¥ 1.4142 ≤ √2 ≤ 1.4143¥ 1.41421 ≤ √2 ≤ 1.41422¥ 1.414213 ≤ √2 ≤ 1.414214¥ ...That way, instead of constructing a number ε such that
> 0 < ε < 1> 0 < ε < 0.1> 0 < ε < 0.01> 0 < ε < 0.001> ...we get
¥ 0 ≤ ε ≤ 1¥ 0 ≤ ε ≤ 0.1¥ 0 ≤ ε ≤ 0.01¥ 0 ≤ ε ≤ 0.001¥ ...which is satisfied by ε = 0.
And instead of constructing a number x such that
¥ 0 < x < 1¥ 0.9 < x < 1¥ 0.99 < x < 1¥ 0.999 < x < 1¥ ...,which would be infinitesimally close to 1, and which would get us an infinitesimal number by just doing 1-x, we get
¥ 0 ≤ x ≤ 1¥ 0.9 ≤ x ≤ 1¥ 0.99 ≤ x ≤ 1¥ 0.999 ≤ x ≤ 1¥ ...which is satisfied by x = 1.
This is how we've been modeling real numbers in this thread since
>>8908 which has the full details.
No.12038
>>11996>They made use of a preexisting Java library to do the calculations:>https://www.hboehm.info/crcalc/I said that like they just used someone else's code, but on second look I see the guy who wrote that library is the author of the CACM paper. He developed it while he was working at SGI and then got hired by Google to work on Android stuff.
No.12423
>>11942>>11948I've been thinking again about how to do hand arithmetic with infinite decimals, in this case division. I've tried this before, but my calculation of the error on the result at each step was an ugly and unmemorable formula. I think I've found a better way to think about the error on the result, which is to first work out the error on the remainder at each step. There are two contributions, one from the truncation error on the dividend, and one from the error on what's been subtracted from the dividend (due to truncation error on the divisor). Then the error bound on the result is
(|remainder| + |error on remainder|) / (lower bound on |divisor|).
You could also forgo taking the absolute value of the remainder and work out separately the error bounds in each direction, which could occasionally help squeeze out a digit of the quotient a bit earlier.